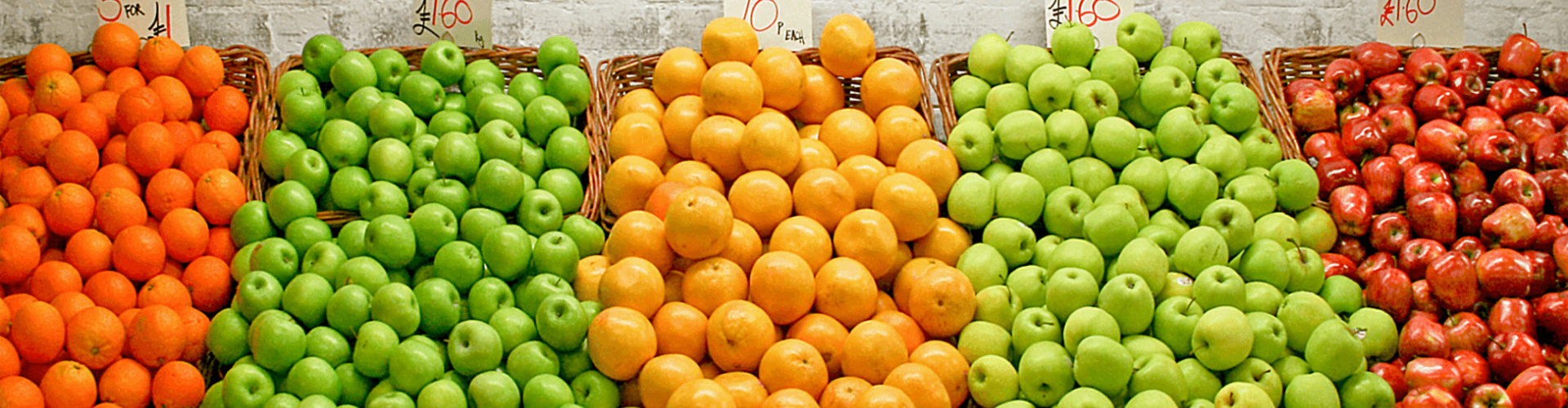
Context-dependent neural codes: task-agnostic or task-specific?
By April Cashin-Garbutt
Context is everything – without it we cannot make sense of the world. It is essential that our brains can control our behaviour in changing contexts and switch nimbly between tasks. Take a trip to the supermarket for example. When choosing which fruit to buy, you can judge the items by size but you can also switch to judging by colour. Here we are using the same sensory stimulus, e.g. the same fruit, in different ways depending on what we’re trying to achieve. This ability to switch between context-dependent tasks is essential and it’s something we carry out numerous times a day.
Exactly how the brain acquires representations to support context-dependent tasks is still an open question for neuroscience. Researchers at the Sainsbury Wellcome Centre and Gatsby Computational Neuroscience Unit are working together with neuroscientists at the University of Oxford to understand the geometry of neural codes in both artificial neural networks and the human brain to help answer this question.
Task-agnostic versus task-specific representations
There are two alternative ways in which the brain can solve a context-dependent task. In the fruit decision example, the neural code could be task-agnostic by having lots of neurons responding to many idiosyncratic combinations of size, colour, and context. Then the appropriate response for a task can be produced by combining the activity of neurons that just happen to activate to the necessary sizes, colours, and context. The other option is to have a very task-specific code, which uses neurons that respond only to colour for the colour task, and another set of neurons that respond only to size for the size task.
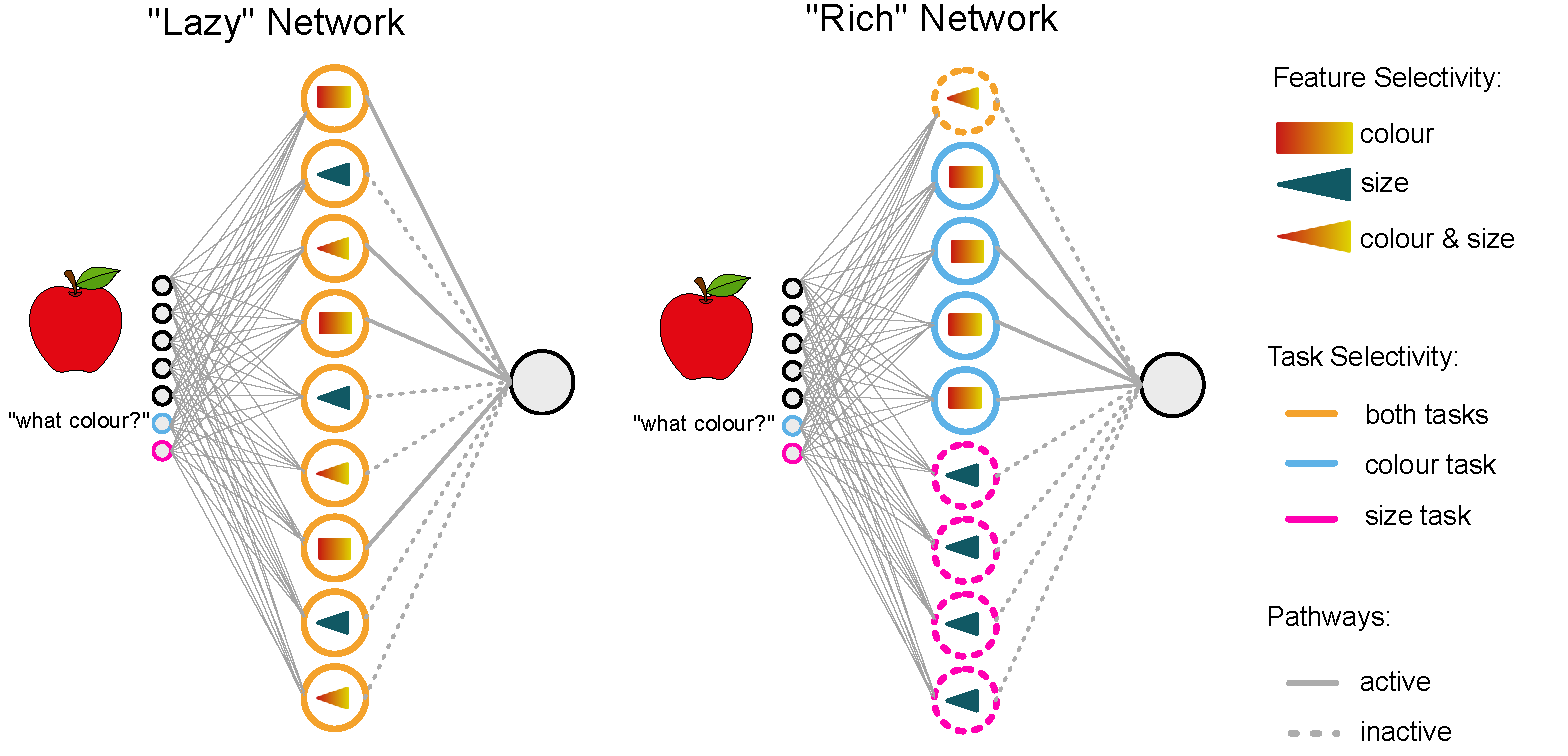
These task-agnostic and task-specific representations match closely with two distinct ways that artificial neural networks can solve problems, referred to as the ‘lazy’ and ‘rich’ regimes in machine learning literature. In the lazy regime, the exact same representation works for any task so no changes to the representation are necessary for a new task, instead you just change how you read it out. Whereas, in the rich regime, the code learns rich features that are relevant to the task being performed.
Comparing computational simulations to biological brains
While both the task-agnostic and task-specific representations could successfully solve our fruit task behaviourally, it was not known which way the brain achieves this neurally. To determine whether biological brains use neural coding patterns that are consistent with the lazy or rich learning regimes, the researchers compared computational simulations with behavioural testing and neuroimaging in humans and reanalysed a dataset with single cell recording data from macaque brains.
Firstly, the researchers explored computational simulations to understand the relative costs and benefits of the task-agnostic and task-specific schemes. This gave them insights into trade-offs between learning speed and robustness for example and also equipped them with predictions about how biological systems might solve these tasks.
Next the researchers trained human participants on a similar task and collected fMRI data to look at neural activity from the human brain. While fMRI data provides a view into the coarse geometry of the neural representation, it cannot resolve single neurons. So in addition, the researchers used a freely available dataset with single cell recordings, collected by Valerio Mante (ETH Zurich) and collaborators from the brains of macaques that had been given a very similar task judging direction and colour in specific contexts. This dataset allowed the scientists to test more fine-grained predictions that had arisen from the theoretical work.
By comparing the artificial neural networks with the biological brains during context-dependent tasks, the researchers found evidence that humans and macaques use a rich regime. This means that the representations were highly task-specific, for example in the colour context, only colour was encoded.
Exploring the geometry of neural codes
The researchers explored a new approach by studying neural geometry. Traditionally, neuroscientists have looked at the change of activity in individual neurons by measuring their firing rate. Recently, a new view has emerged in the neuroscience community that focuses on the geometry of the activity of populations of neurons in response to different stimuli. This approach is giving scientists insight into how tasks shape the way information is represented in the brain across many neurons.
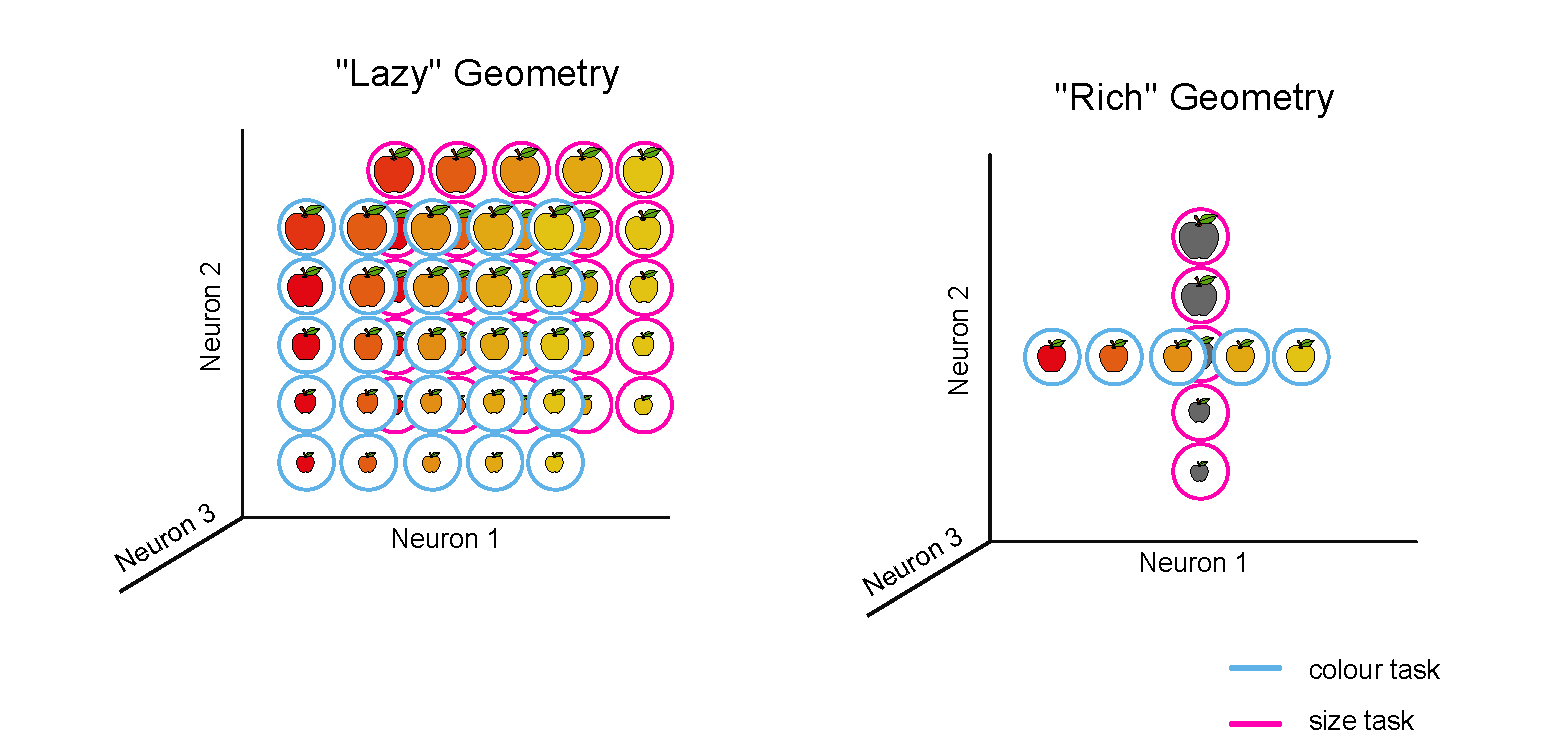
When comparing the geometry of neural codes in both neural networks and biological brains during context-dependent tasks, the researchers found that the colour and shape were mapped onto orthogonal axes, one for each context.
The reason the researchers think these orthogonal axes appear is that the different banks of units are allocated to each task, so different subsets of neurons encode the relevant dimensions in the colour context and the shape context. The researchers have shown that not only is there a suppression of task-irrelevant information but also that the brain is representing the information in a very specific way in the population activity. Simulations in artificial networks suggest this rich representation may be more robust to neural noise, providing one possible reason why the brain might use this scheme.
Next steps
The next steps for the researchers is to understand how these representations build-up. It is not possible that every task in our lives can be allocated a completely different set of neurons as we would run out at some point. Also, it would make more sense to reuse some of the things we already know, but how the brain can assemble the right representations is a key problem for neuroscientists to solve.
Understanding how biological brains use such representations might also advance artificial intelligence. As while deep neural networks have revolutionised engineering, multitasking is one area where they currently struggle. Investigating these types of behaviour that we as humans can do easily, might help us improve AI systems in the future.
Read the paper in Neuron: Orthogonal representations for robust context-dependent task performance in brains and neural networks