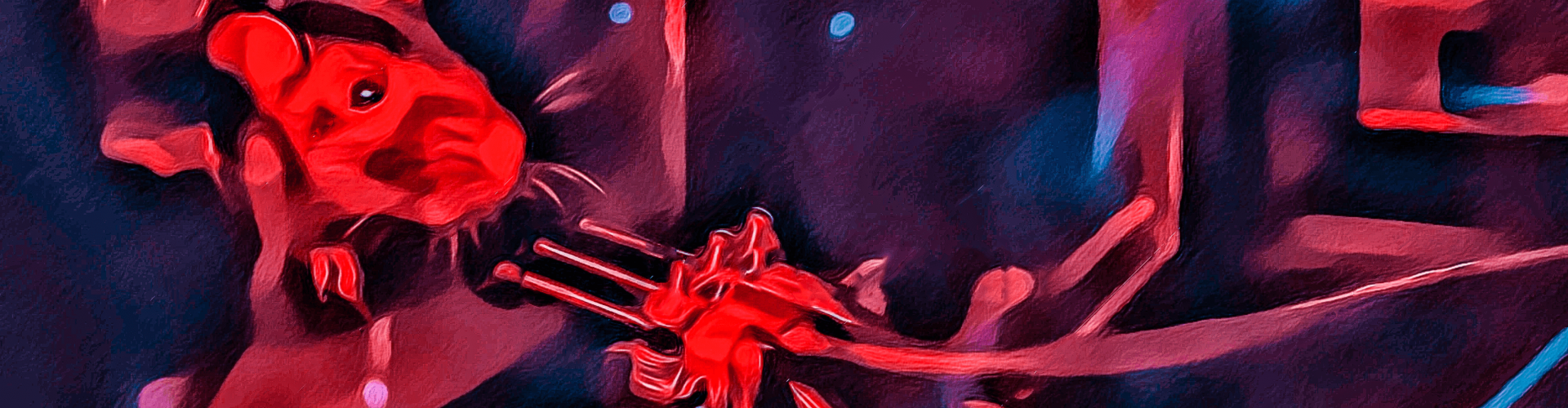
Learning to learn: sacrificing short-term reward for long-term gain
By April Cashin-Garbutt
Choosing what to learn and deciding how to do so is a huge decision. Such choices can have significant long-term impact and we often decide to sacrifice a lot in the short-term to gain a bigger future reward. Neuroscientists are starting to unpack whether and why our brains may prioritise learning early on to yield considerable advantages long-term.
Studying the impact of decision-making on learning
During their time at Harvard University, Andrew Saxe, now Joint SWC/GCNU Group Leader, and Javier Masís, now Postdoctoral Research Fellow at the Princeton Neuroscience Institute, worked together with their team to study the impact of decision-making on learning. They studied rats taking part in a visual discrimination task.
“When evaluating decision-making tasks, scientists frequently ask whether subjects are maximising their reward rate by optimally balancing the speed and accuracy of their choices. If you’re very quick, you’ll answer many questions inaccurately. If you’re very slow, you’ll answer few questions accurately. For every skill level, there’s an optimal balance of the two,” explained Dr Javier Masís. “We found that at the end of training, most of our rodent subjects were indeed optimally balancing the speed and accuracy of their choices,” he added. "But the puzzling thing is that at the start of training they most certainly were not optimal, and we had to know why.”
Previous studies have traditionally focused on data from animals that have already learned a task. Instead, Andrew, Javier and team were interested in the entire learning period and how rats progress to become very good at a task. They suspected that the fact that the rats could learn the task had a lot to do with this apparent paradox in their behavior.
“We observed that early on in learning, when the rats were very bad at a task, they did not randomly guess the answer quickly to try to maximise reward, but instead slowed down. While this led to the rats paying a big cost in terms of initial reward rate, they were able to observe the stimulus more fully at the slower rate, which allowed them to learn and get better at the task faster than they would have otherwise, resulting in greater rewards over time,” said Associate Professor Andrew Saxe.
They found evidence suggesting that rats have cognitive control of the learning process and that they only invest time in a task that they are able to master. To test this, the team gave one group of rats some blank screens, and the other group of rats a new visual discrimination. If the team’s hypothesis was correct, then the rats with the blank screens should speed up and move towards the ‘fast guessing’ strategy as there was nothing possible to learn, whereas the rats with the visual information should slow down to try to learn the task. This is precisely what the researchers observed, demonstrating that the rats strategically manage the learning process.
“Our findings show that rats can evaluate their learning possibilities and make choices about whether they should spend time on a task. This struck us as particularly sophisticated as they are changing their strategy depending on the learning prospects that are available to them,” explained Dr Andrew Saxe.
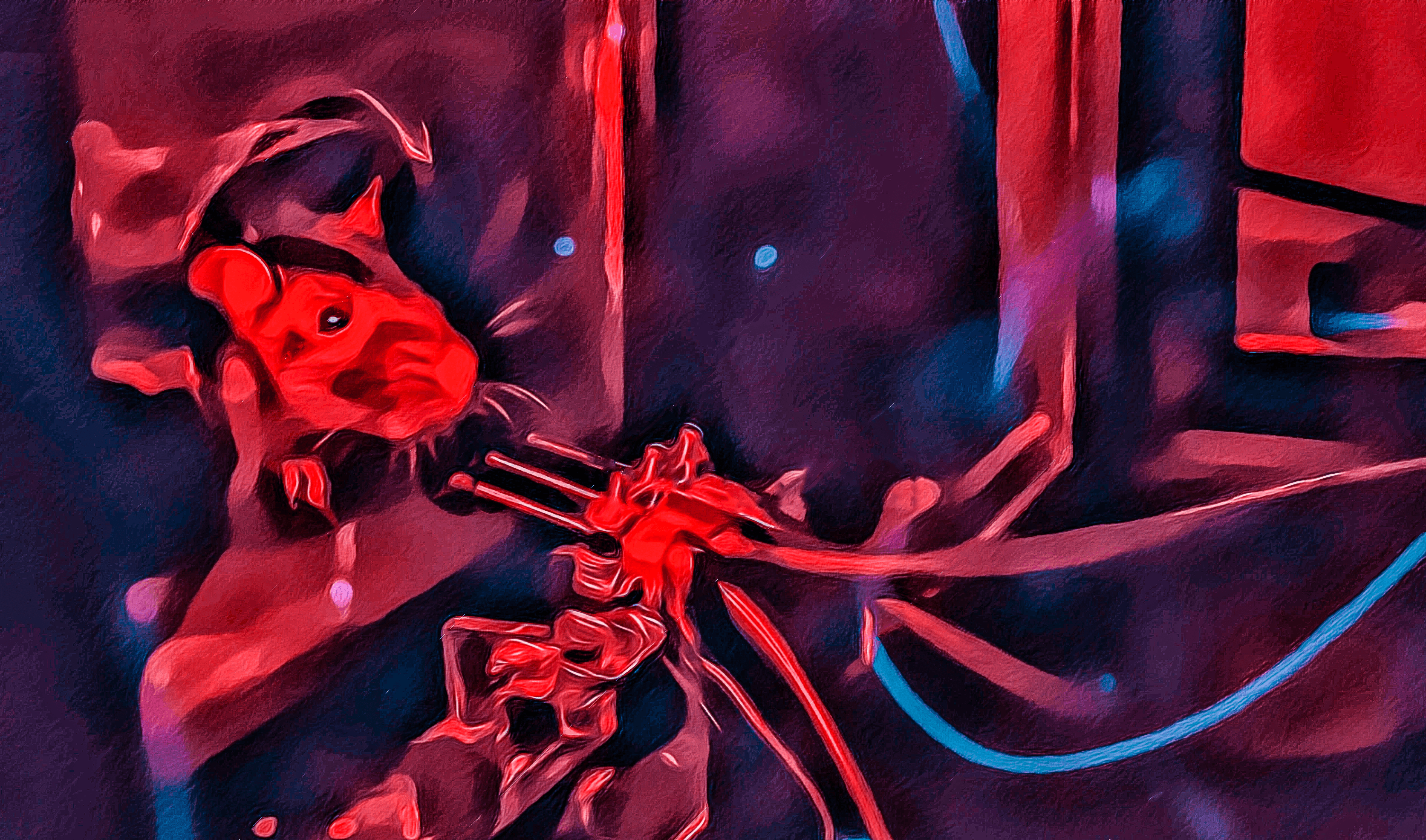
Image by Javier Masís with the aid of Colorcinch, based on an original photograph by Juliana Y. Rhee.
Exploring learning strategies using artificial neural networks
To test their hypothesis that the rats’ slower initial responding did in fact lead to faster learning and more long-term reward, the team used artificial neural network models to simulate the impact of different response strategies (like fast guessing to maximise reward, or starting slow) on learning speed. The model that they developed showed the same trade-off between going slowly at the beginning and learning faster, versus going faster at the beginning and learning more slowly.
“Classical models for decision-making don’t take into account the fact that subjects can learn and improve over time, so we built a model that extended these classical decision-making models to capture the whole learning period,” explained Dr Javier Masís.
“Artificial neural network models are a great model for learning, so we created an artificial neural network that was equivalent to the classical models with the difference that it could also learn,” elaborated Dr Andrew Saxe. “Not only that, but we could solve it mathematically to show that at least for this specific model, there is a very clear trade-off between how much initial reward you want versus how fast you want to learn something.”
“With the model in hand, we now had evidence that the rats’ strategy of slowing down to learn faster was actually the right thing to do in order to maximise total reward, meaning not reward at one particular point in time, but rather over the entire learning period instead,” said Dr Javier Masís.
Extending into human studies and other elements of cognitive control
Following their initial findings, the team are following up with human studies as there are many settings where we have to guess how much we can learn in a new situation and then change our behaviour in terms of how we choose to interact. The researchers also plan to explore other elements of cognitive control in rodents.
“Slowing down is a very simple version of cognitive control, but there are lots of other elements such as which tasks do you engage with in the first place. We plan to investigate how sophisticated cognitive control is in rodents by comparing easy tasks that don’t lead to much reward, with hard tasks that do,” commented Dr Andrew Saxe.
Andrew and team also plan to study how changes in levels of attention and vigilance impact learning. In addition to rodents, they will explore this in humans, which will allow them to have more control of people’s expectations for how much they can learn and see if humans modulate their behaviour near optimally accounting for the learning process.
Maximising learning in AI and human learning
By exploring how changes in strategy impact learning, the hope is that this research could help maximise learning in artificial intelligence. Previous research from DeepMind has shown that their very impressive state-of-the-art deep learning systems don’t do well on similar visual discrimination tasks as they start randomly guessing. This research may help to fix such issues when facing speeded tasks.
The researchers also hope that this work could help optimise curricula for human learning by feeding into the existing knowledge of how to change your behaviour to maximise your learning.
Read the research paper in eLife: Strategically managing learning during perceptual decision making