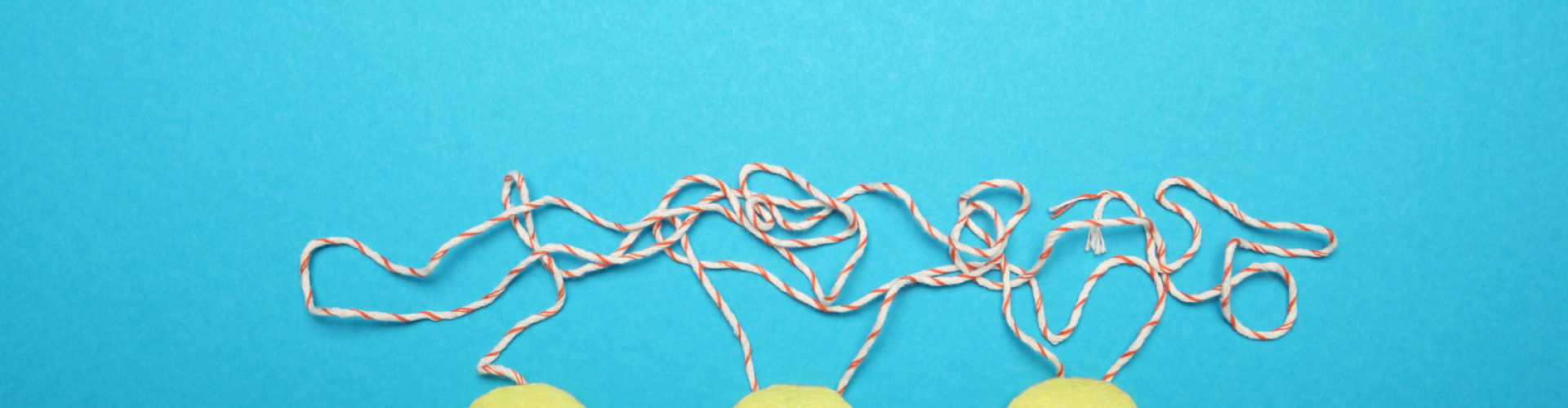
Understanding hippocampal predictive maps: a three-pronged approach
By Hyewon Kim
“On the one hand we have good theoretical models of how the hippocampus functions as a predictive map, and on the other hand we know a lot about the biology of the hippocampus,” described Tom George, SWC PhD student in the Barry and Clopath labs. “But how to connect these has, until recently, been unclear.”
Studying successor representation from different perspectives
Last March, Tom and his advisor Caswell Barry were approached by Ching Fang (working with Emily Mackevicius and Larry Abbott at Columbia University) with a proposal. Having learned that both groups (and a third based in the Clopath lab at Imperial) were pursuing similar ideas about predictive maps in the hippocampus, it was suggested that the three groups could submit their work to the same journal simultaneously. The manuscripts would be reviewed independently and if all accepted, be published at the same time.
Last week, the works from the three groups were published in eLife, jointly addressing the question of how the hippocampus learns to predict the future state of the animal given the current state, known as the successor representation. The three papers offer different angles from which to understand this phenomenon.“It's really exciting that three groups can co-publish in this way – eLife is particularly good at fostering collaborative science,” commented Caswell.
One of the works, with Ching as leading author and who was a recent visiting PhD Student at the Gatsby Computational Neuroscience Unit, shows how recurrent neural networks learn predictive maps with their long-term stable dynamics. “Our perspective focuses on recurrent network models and how neuromodulators can freely tune the predictive strength of the representations,” she described. “This paints a more dynamic role of the hippocampus in retrieving memories and suggests that the hippocampal predictive map may be flexibly adjusted depending on the task at hand.”
Another approach, from the Clopath lab, derives synthetic learning rules that approximate those in the hippocampus. Finally, the work from Tom and Will de Cothi in the Barry lab capitalises on existing knowledge about the circuitry and learning rules in the hippocampus to approximate successor representations under a range of biologically plausible assumptions.
“There is a lot of energy amongst neuroscientists to move towards a model of scientific publishing that is more collaborative and open, so having this triply coordinated publication is an exciting step towards that,” Ching shared. “I think it’s also strengthened each of our individual papers to be able to discuss with groups across different institutions and perspectives.”
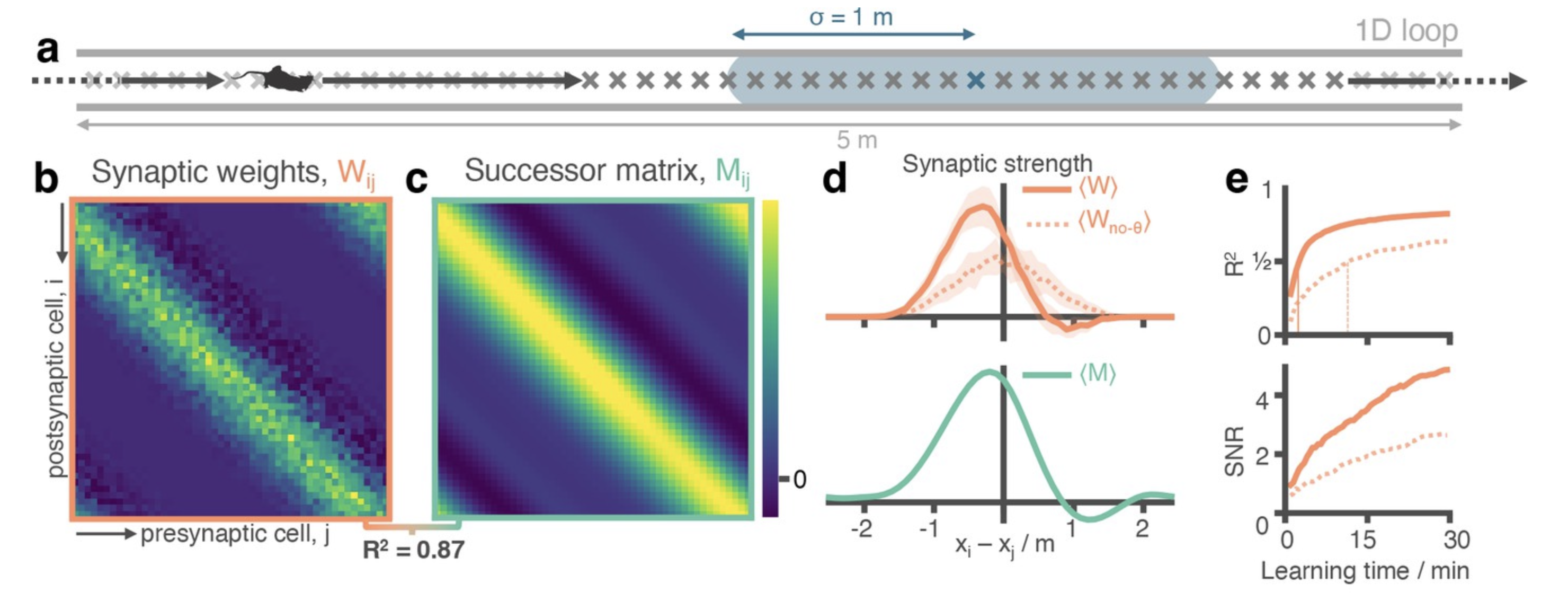
Figure adapted from George et al. eLife 2023
Understanding Hebbian learning rules and theta sweeps
In 1949, Donald Hebb observed that the weight of the synaptic connection between two neurons can either get stronger or weaker depending on the timing of the neurons’ activation – neurons that fire together, wire together. “Learning rules in much of the brain, including hippocampus, are likely to be pretty simple,” Tom explained. “Spike-time dependent Hebbian learning rules have been measured in hippocampal neurons.” When a presynaptic neuron fires before a postsynaptic neuron, the connection between the two is made stronger. But when the postsynaptic neuron fires first, the connection is then made weaker. This form of Hebbian learning is known as spike-timing dependent plasticity (STDP).
Action potential spikes in the hippocampus are coherent in timing to theta oscillations in the brain. These coordinated spikes share the timescales of the Hebbian STDP synaptic learning rules meaning that cells in hippocampus bind in a predictive manner, much like successor representations. “By taking into account the biological components already known about hippocampus, such as neural oscillations and which parts are connected to which other parts, we were able to connect synaptic learning rules to the broader phenomenon of predictive maps,” Tom described.
Pursuing biologically plausible models of reinforcement learning
Up until these studies, there were no good frameworks for understanding how hippocampal predictive maps, which are fundamental to an agent deciding how to act and behave in the future, would be learned. By showing similar learning principles in biological terms, Tom and Caswell hope this work will be the start of further pursuing biologically plausible models of reinforcement learning and cognitive maps in the brain. “Our independent findings show that there are many biologically plausible solutions to learn predictive maps and that this type of representation can be quite accessible in neural circuits,” Ching added.
“This experience has been a reminder of what makes science fun in the first place: working together with people who are excited about the same problems and combining our different ideas together,” she continued. “The fact that predictive maps can arise from different types of simple learning rules suggests that predictive representations may be ubiquitous in the brain and also found in other brain regions outside the hippocampus.”
“The successor representation has been very influential and, in the last few years, has reshaped how we think about the spatial memory system,” Caswell added. “What was missing though was a plausible way for the brain to implement the computations necessary to generate such a representation. Our work, and the work of the other two groups, show that in the hippocampus a combination of existing phenomena are sufficient to generate a very close approximation of the successor representation.”
“The fact that we were all working toward the same question could have easily been ignored,” Tom pointed out. But by choosing to submit their manuscripts to the same journal at the same time, choosing collaboration over competition, the three groups spanning the Atlantic were able to share with the world a richer view of how the hippocampus enables goal-directed spatial navigation through successor representations.
Read the eLife paper: Rapid learning of predictive maps with STDP and theta phase precession; George*, de Cothi*, Stachenfeld and Barry
Learn more in the simultaneously published papers in eLife:
Neural learning rules for generating flexible predictions and computing the successor representation; Fang, Aronov, Abbott and Mackevicius
Learning predictive cognitive maps with spiking neurons during behaviour and replays; Bono*, Zannone*, Pedrosa and Clopath