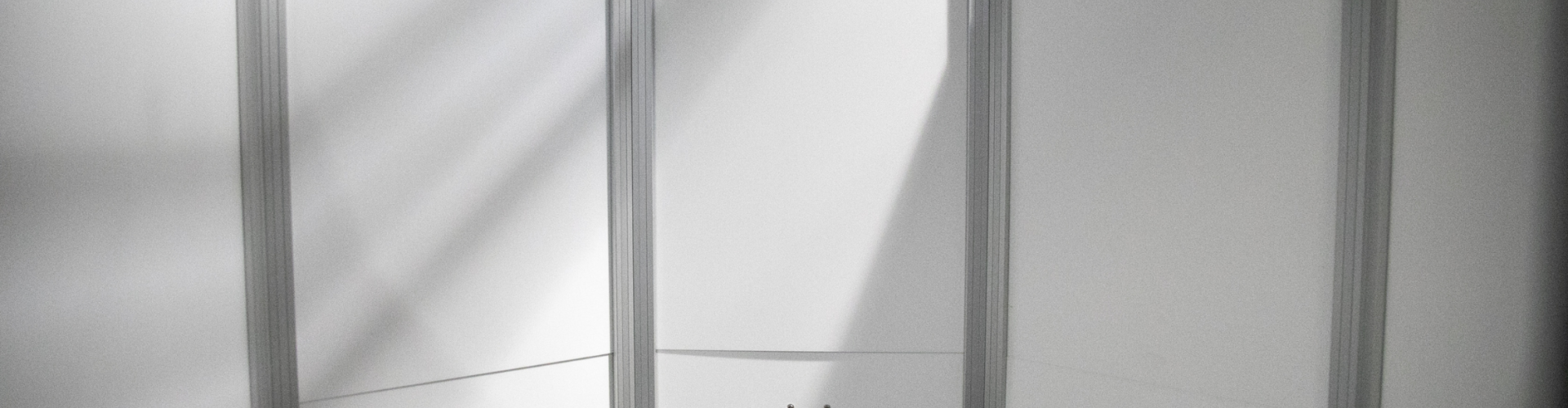
What can mice teach us about football?
Understanding decision-making in multi-agent competitive environments
By April Cashin-Garbutt
Football is adored by fans all around the world. But what makes “the beautiful game” so captivating? Is it the interactive nature, the uncertainty of the outcome, or the complexity of the strategy? All of these elements enticed researchers at the Sainsbury Wellcome Centre to study embodied multi-agent decision-making in a dynamically changing world. But what can mice really teach us about football?
Studying real-life decisions
From the inception of her lab at the Sainsbury Wellcome Centre, Dr Ann Duan has been interested in studying real-life decision-making and recent advances in technology are allowing her to realise this ambition.
“A lot of decision-making research over the past few decades has been extremely controlled and has focused on how isolated factors influence specific cognitive processes during tasks. But recent technical advances mean we are finally equipped to take on more complex approaches to decision-making. This is allowing us to get closer to understanding real-life decisions,” explained Dr Duan, Group Leader at SWC and last author on a new preprint in bioRxiv.
The kind of decisions the researchers are investigating are just like the choices football players make during a match. In a multi-agent environment, players must make rapid decisions, but they also need to be flexible and integrate many sources of information. They need to consider their own position on the field; the location of the opposition, how fast they are, their previous strategy, their ongoing behaviour, and many more elements, all of which need to be integrated.
To begin to understand how such complex multi-agent decisions are made in a very dynamically changing world, research teams led by Dr Duan and Dr Jeffrey C. Erlich at SWC designed a novel behavioural task for mice. This new approach is allowing them to unravel decision-making in an ethologically-relevant but still quantifiable way.
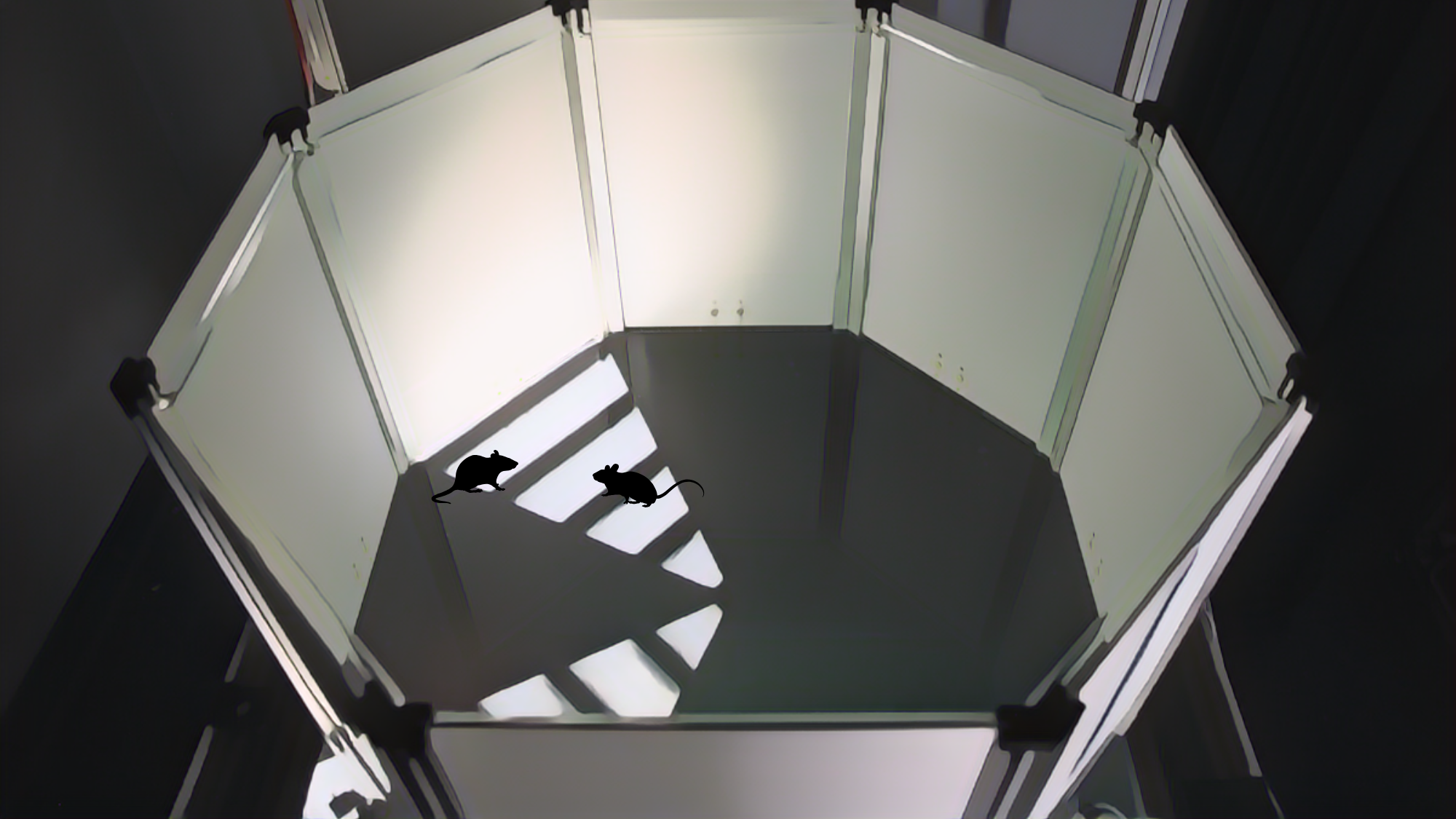
Introducing the Octagon
In collaboration with NeuroGEARS and the FabLab at SWC, the team have developed a foraging arena called “the Octagon” which blends the embodied nature of spatial mazes with the flexibility of virtual reality paradigms. This eight-sided arena allows researchers to present mice with dynamically changing reward locations using floor-projected visual displays. One visual pattern indicates a patch has a high reward and another pattern indicates a low reward.
“We train each mouse on its own to learn what the visual patterns mean, and gradually they develop a preference for the high value patch in a generalisable way. And then as soon as we put them together so that only the faster choice gets rewarded on each trial and the slower mouse gets nothing, they naturally get the idea that they are in a race.
Competition needs no training. As a result, we have a really rich task that has all this natural behavioural variance and complexity without having to overtrain the mice, as the task taps into something very natural for the animals,” explained Dr Erlich, Group Leader at SWC and co-corresponding author on the new bioRxiv preprint.
Strategic preference shifts
Once the trained mice had learned a preference for the high reward patch, the researchers put two trained cage mates together and introduced a simple rule: the mouse that gets to a patch first (whether high or low) receives a reward and the other mouse receives nothing.
“Suddenly, the chance of getting a reward changes from trial to trial depending on where they are and what they choose. For example, they could be close to the low patch but decide to go to the high patch and potentially receive nothing at all if they were too slow. So, each trial is a unique decision scenario where the animals must integrate lots of information like the goal location and value, the mouse’s own position relative to the goal and even how fast is their opponent,” explained Dr Duan.
“There is actually no general optimal strategy. There’s only an optimal strategy if you know the strategy of your opponent,” explained Dr Erlich.
The team found that under competition, animals choose the high reward patch less than when they’re by themselves. This makes sense as choosing the high patch can sometimes increase the chance of losing and getting nothing at all. By analysing the data, the researchers showed that animals shift their strategy depending on how far away they are from the high patch and the speed of their opponent. The faster the opponent, the more the mouse changed their strategy compared to the solo context.
Competing against a ghost
The researchers also explored how much mice integrate opponent information to make their decision versus how much of this could be achieved by reward learning alone. To do this, they used deep Q-learning networks (DQN) to simulate a ‘socially-blind’ mouse playing against an invisible “ghost” opponent.
In this scenario, the simulated mouse did not know there was an opponent, but they experienced that, in some sessions, there were trials where they received no reward (i.e., when the ghost opponent responded first). Using these simulations, the team were able to determine to what extent mice could adjust to the consequences of the ghosts’ actions, as opposed to integrating opponent information to guide ongoing choices.
“In reinforcement learning there’s a dichotomy of model-free versus model-based. In model-free, your outcomes (i.e. the size of the reward in this trial) drive your future actions, whereas in model-based, you actually know how the world works (i.e. you know that if your opponent is close to high you should go to your closest patch because otherwise you will get nothing). And we wanted to understand which was the case.
What we found was that even a “socially”-blind DQN can shift its strategy from a solo session to a social session and back again. This means that it can infer that it is in a solo session as it always gets rewards. Then when suddenly it doesn’t receive a reward (as it is in a social session), it knows it needs to update its strategy and choose low more,” explained Dr Erlich.
“We were surprised to find how many behavioural phenomena that we initially thought were opponent-guided can be achieved by learning alone. This doesn’t mean that the animals are doing this by learning alone, but that they can. Importantly, there are some behavioural metrics that would never be achieved by learning alone, for example the sensitivity to opponent position on a trial-by-trial basis, and these simulations allowed us to discover what these metrics are to guide analyses and interpretations,” explained Dr Duan.
Understanding the decision process
In addition, the researchers decided to build a dynamical model from first principles. This was because, while useful, DQN is somewhat of a black box that makes it hard to know the internal mechanisms underlying each strategy.
The authors built on bistable attractor models, classically used to model solo decision-making, to see how different parameters, such as value input, decision noise, and sensitivity to initial conditions, can influence the dynamics of the decision process within a trial, across trial conditions, and across the solo and social contexts. The team plan to use this model as a framework to make predictions of brain mechanisms and understand neural activity and perturbations in the future.
Next steps
“Now we have a behavioural and theoretical framework to probe social decision-making by extending approaches used in solo decision-making studies. This means that going forward we have a solid foundation for interpreting neural data and perturbations of neural circuits during “football”-like behaviours in mice,” explained Dr Duan.
In the future, the team hope to explore the neural mechanisms underlying individual differences in behaviour. This could include things like sex, social dominance, and curriculum shaping.
They are also interested in investigating how wild type animals compare with mouse models of autism spectrum disorder (ASD).
“The complexity of this task means that we will be able to determine which individual components of decision-making are better, the same or worse, in mouse models of developmental disorders compared with their wild type littermates. This is one of the main future directions we are exploring in the longer term,” concluded Dr Duan.
Find out more
- Read the preprint in bioRxiv, ‘Mice dynamically adapt to opponents in competitive multi-player games’
- Learn more about research in the Duan Lab and Erlich Lab.