Abstract
The mammalian hippocampus contains a cognitive map that represents an animal’s position in the environment and generates offline “replay” for the purposes of recall, planning, and forming long term memories. Recently, it’s been found that artificial neural networks trained to predict sensory inputs develop spatially tuned cells, aligning with predictive theories of hippocampal function. However, whether predictive learning can also account for the ability to produce offline replay is unknown. I will report recent results that spatially-tuned cells, which robustly emerge from all forms of predictive learning, do not guarantee the presence of a cognitive map with the ability to generate replay. Offline simulations only emerged in networks that used recurrent connections and head-direction information to predict multi-step observation sequences, which promoted the formation of a continuous attractor reflecting the geometry of the environment. These offline trajectories were able to show wake-like statistics, autonomously replay recently experienced locations, and could be directed by a virtual head direction signal. Further, we found that networks trained to make cyclical predictions of future observation sequences were able to rapidly learn a cognitive map and produced sweeping representations of future positions reminiscent of hippocampal theta sweeps. These results demonstrate how hippocampal-like representation and replay can emerge in neural networks engaged in predictive learning, and suggest that hippocampal theta sequences reflect a circuit that implements a data-efficient algorithm for sequential predictive learning. Together, this framework provides a unifying theory for hippocampal functions and hippocampal-inspired approaches to artificial intelligence.
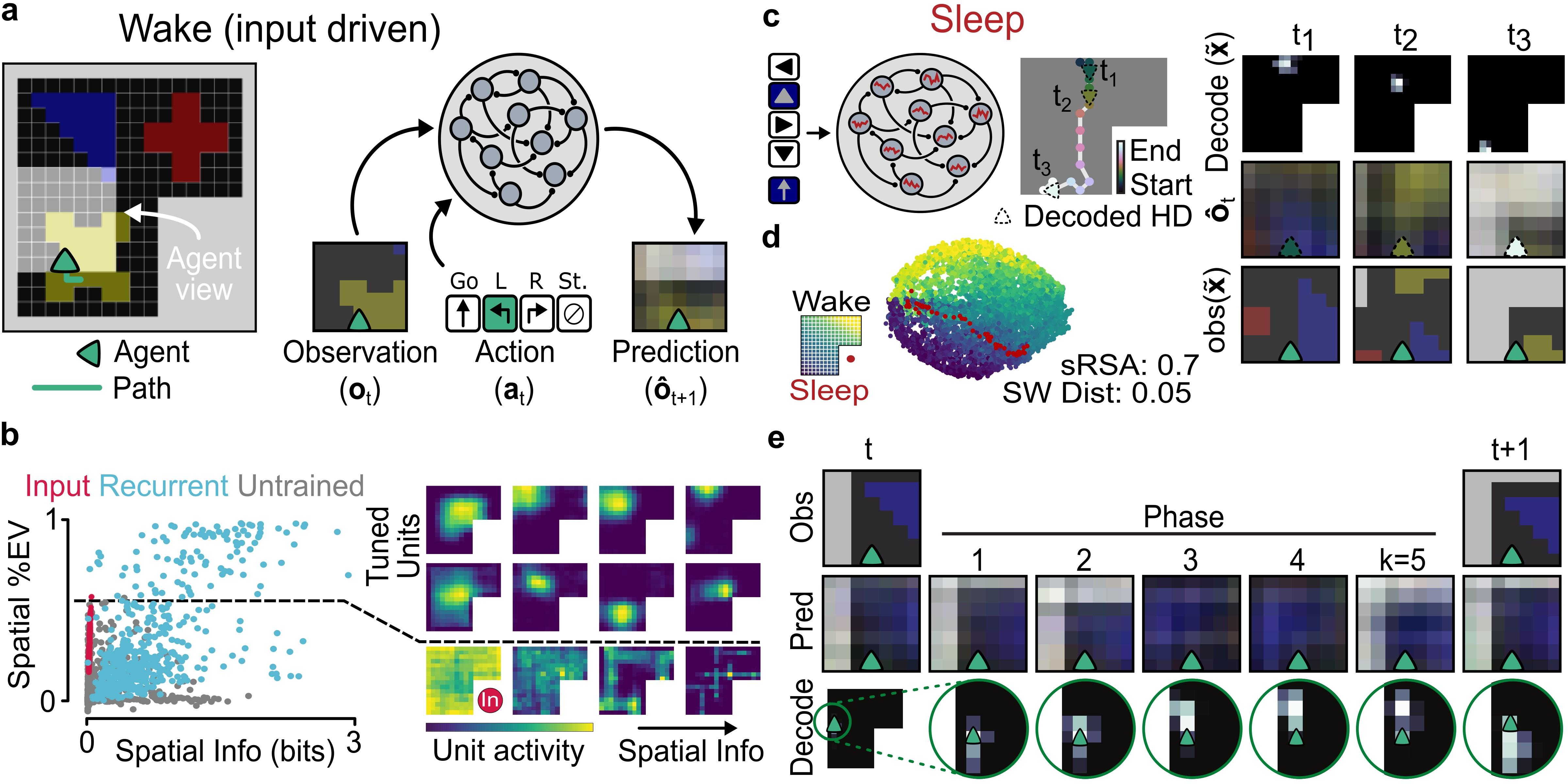
Biography
Dan did his PhD with György Buzsáki and John Rinzel at NYU, and is now a postdoc with Blake Richards and Adrien Peyrache. His work focuses on the generation and use of offline, or “spontaneous", activity to support learning in biological and artificial neural networks. He uses biologically-inspired neural network models, neural data analysis, and works closely with experimental collaborators. He also has a strong interest Philosophy of Science and how it can be applied to scientific practice — most notably the use of computational models in neuroscience, and how mechanistic and normative approaches can work together to understand neural systems.