Abstract:
Much work has been devoted to formal characterizations of the ways that humans and other animals make decisions in mostly stable, near-asymptotic, regimes in behavioural tasks. Although this has been highly revealing, it hides aspects of learning that happen on the way to this asymptote. I will discuss three attempts that we have been making to use parametric and non-parametric models to capture more of the course of learning: in a spatial alternation task for rats, the International Brain Lab task for mice, and an alternating serial reaction time task for humans. Many incompletely addressed challenges arise - for instance separating individual differences in outcomes into differences between models, differences between parameters within a model, or path dependence from stochasticity in early choices and outcomes.
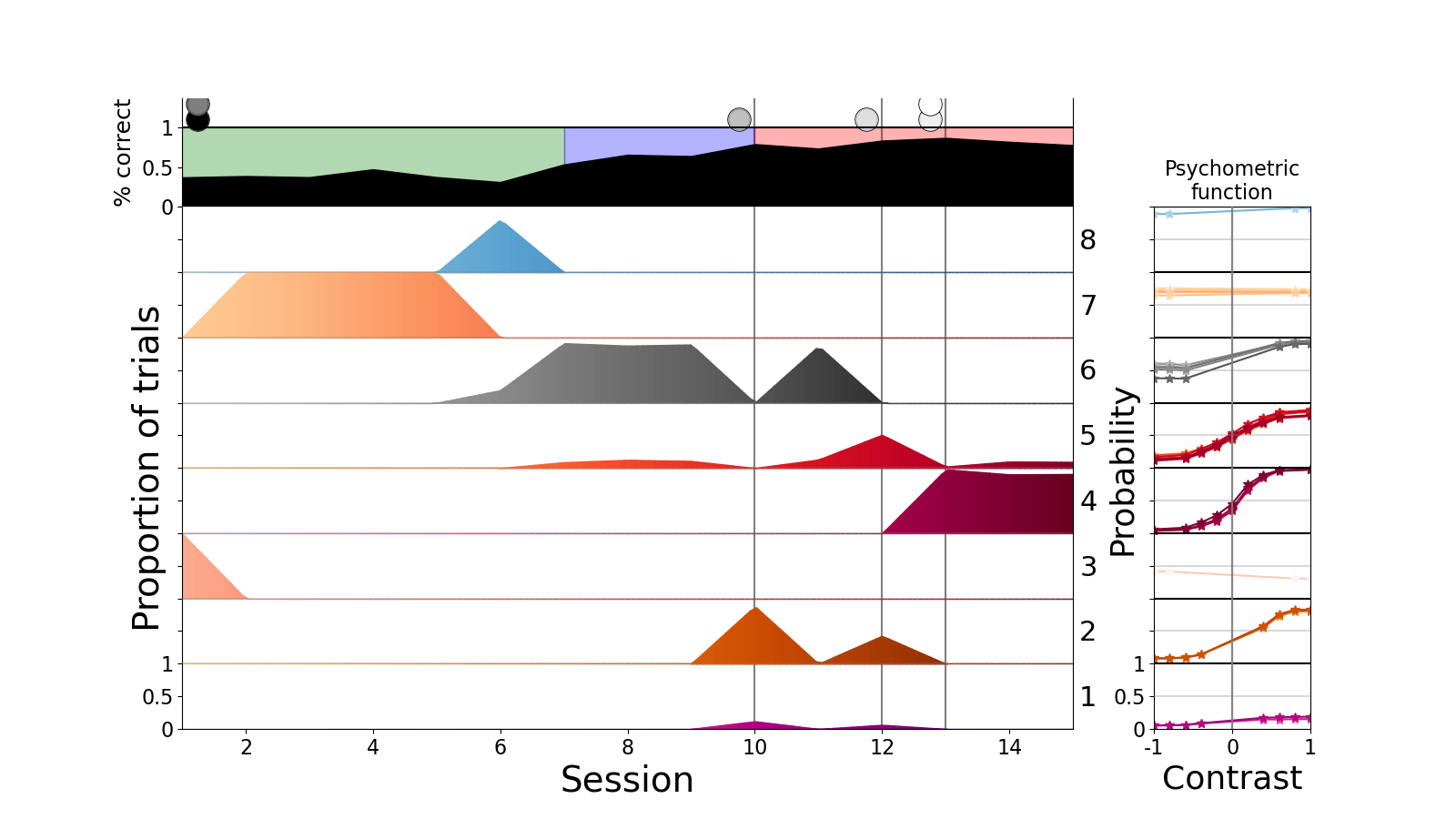
Biography:
Peter Dayan did his PhD in Edinburgh, and postdocs at the Salk Institute and Toronto. He was an assistant professor at MIT, then he helped found the Gatsby Computational Neuroscience Unit in 1998. He was Director of the Unit from 2002-2017, and moved to Tübingen in 2018 to become a Director of the Max Planck Institute for Biological Cybernetics. His interests centre on mathematical and computational models of neural processing, with a particular emphasis on representation, learning and decision making.