Abstract:
The rich repertoire of skilled mammalian behaviour is the product of neural circuits that generate robust and flexible patterns of activity distributed across populations of neurons. Decades of associative studies have linked many behaviours to specific patterns of population activity, but association alone cannot reveal the dynamical mechanisms that shape those patterns. Are local neural circuits high-dimensional dynamical reservoirs able to generate arbitrary superpositions of patterns with appropriate excitation, or might circuit dynamics be shaped in response to behavioural context so as to generate only the low-dimensional patterns needed for the task at hand? Here, we address these questions for primate motor cortex activity associated with rapid reaching movements, by delivering optogenetic and electrical microstimulation perturbations during behaviour. Computational models that capture the dynamical effects of these perturbations show that motor cortical activity during reaching is shaped by a self-contained low-dimensional dynamical system. Combining a novel analytic approach that relates measured activity to dynamical models of excitatory and inhibitory neurons with theoretical analysis of such systems, we find that the dynamical subspace is oriented so as to be robust to strong non-normal amplification within cortical circuits, and that stimulation only perturbs behavior to the extent that it alters activity within this subspace. Our results resolve long-standing questions about the dynamical structure of cortical activity associated with movement, and point the way to the dynamical perturbation studies needed to understand how neural circuits throughout the brain generate complex behaviour.
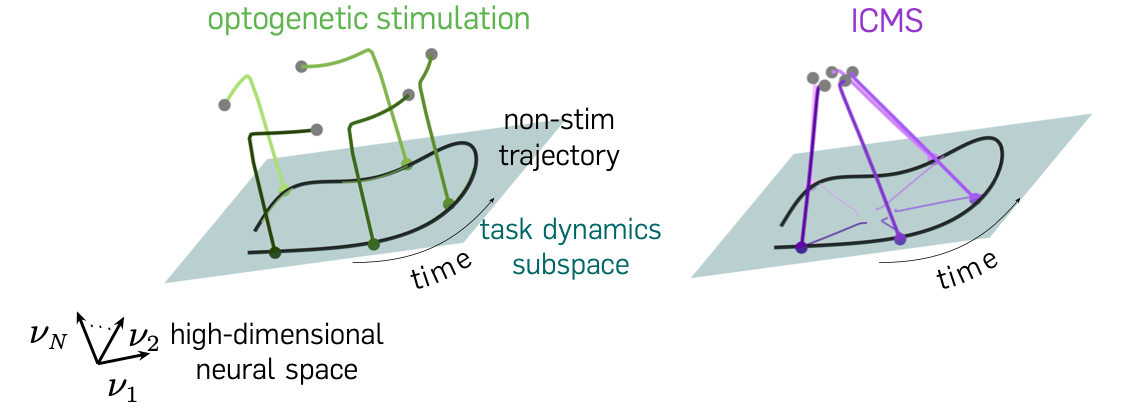
Biography:
Lea Duncker is a postdoctoral researcher at Stanford University, where she works with Krishna Shenoy and Scott Linderman. She has a bachelor of science in natural sciences and a master of science in computational statistics and machine learning, both from University College London. Lea obtained her Ph.D. at the Gatsby Computational Neuroscience Unit, under the supervision of Maneesh Sahani. Her research is centered around characterizing and interpreting population-level structure in neural data, with a focus on dynamical systems and motor control.