Abstract:
Currently Deep Reinforcement learning is undergoing a revolution of scale, fuelled by jointly running the environment, data collection, and training loop on the GPU, which has resulted in orders of magnitude of speed-up for many tasks.
In this talk I start by presenting examples of our recent work which have been enabled by this revolution, spanning multi-agent RL, meta-learning, and environment discovery.
I will end the talk by outlining failure modes of relying on GPU accelerated environments and possible paradigms for the community to collectively address them, ranging from promising research directions to novel evaluation protocols.
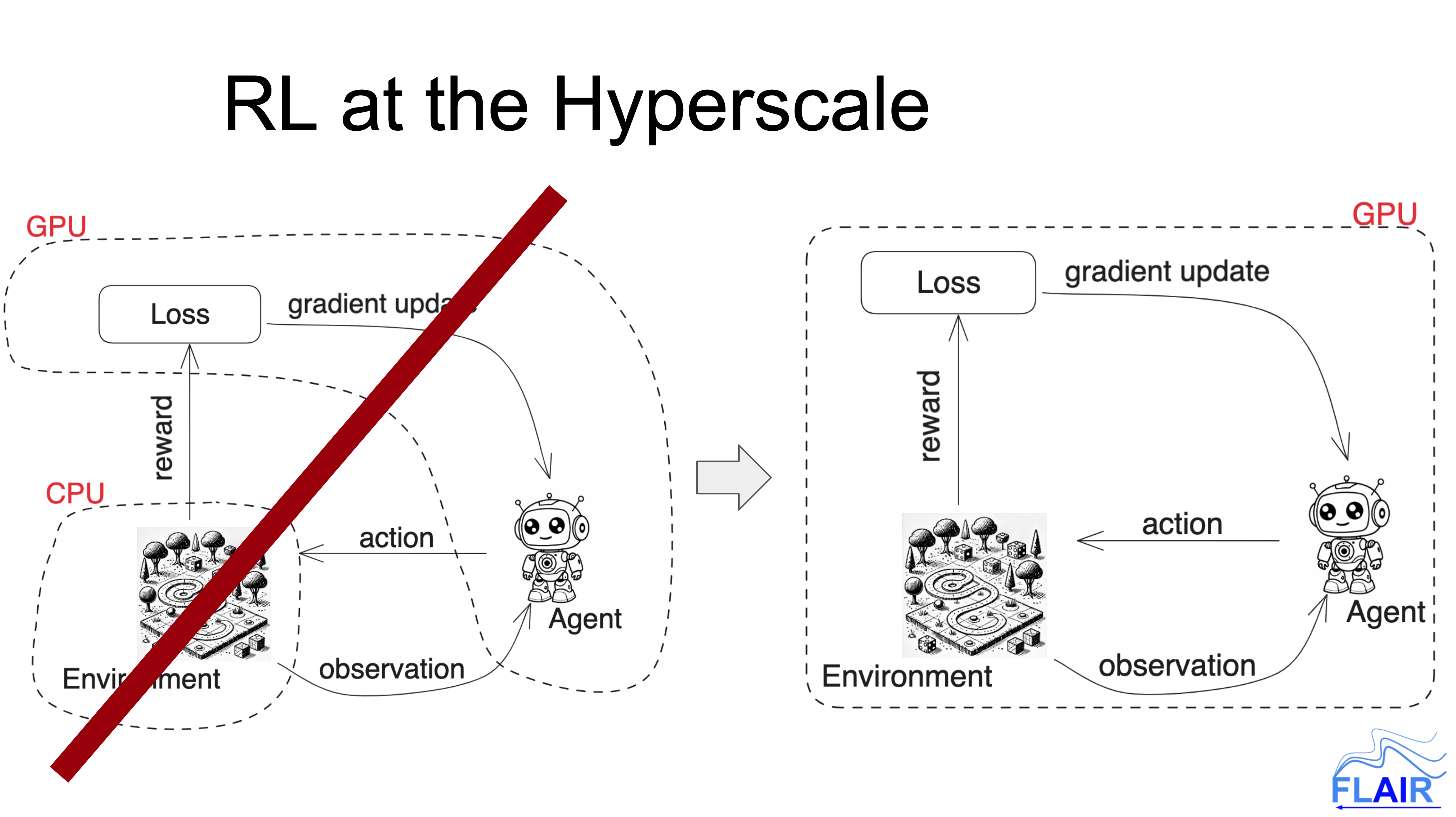
Biography:
Prof Jakob Foerster leads the Foerster Lab for AI Research at the Department of Engineering Science at the University of Oxford. During his PhD at Oxford, he helped bring deep multi-agent reinforcement learning to the forefront of AI research and spent time at Google Brain, OpenAI, and DeepMind. After his PhD he worked as a (Senior) Research Scientist at Facebook AI Research in California, where he developed the zero-shot coordination problem setting, a crucial step towards safe human-AI coordination. He was awarded a prestigious CIFAR AI chair in 2019 and the ERC Starter Grant in 2023. More recently he was awarded the JPMC AI Research Award for his work on AI in finance and the Amazon Research Award for his work on Large Language models. His past work addresses how AI agents can learn to cooperate, coordinate, and communicate with other agents, including humans.
Currently the lab is focused on developing safe and scalable machine learning methods that combine large scale pre-training with RL and search, as well as the foundations of meta-and multi-agent learning. As of September 2024 Prof Foerster returned to FAIR and Meta AI in a part time position to help build out a new team investigating multi-agent approaches to universal intelligence.