Abstract:
Recent advancements in reinforcement learning (RL) algorithms have enabled artificial agents to perform in an array of complex tasks at superhuman levels. One of such algorithms is distributional RL, in which a diversity in reward prediction error enables the system to learn the entire distribution of reward magnitudes, instead of the average as done in conventional RL frameworks. In our previous study, we showed that the activity of dopamine neurons is consistent with distributional RL for reward magnitudes (Dabney et al., 2020). A theoretical study showed that distributional RL can be extended to another dimension, temporal horizons of rewards (Tano et al., 2020), yet empirical support for this idea is missing. In this talk, I will present our recent work supporting distributional RL across temporal horizons and discuss its implications.
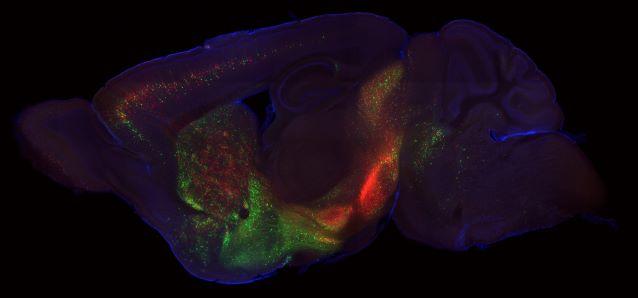
Biography:
Naoshige Uchida is a professor at the Center for Brain Science and Department of Molecular and Cellular Biology at Harvard University. He received his Ph.D. from Kyoto University in Japan, where he worked on the molecular mechanism of synaptic adhesions in Masatoshi Takeichi’s laboratory. He then studied olfactory coding in Kensaku Mori’s laboratory at the Brain Science Institute, RIKEN, Japan. He then joined Zachary F. Mainen’s laboratory at Cold Spring Harbor Laboratory, New York, where he developed psychophysical olfactory decision tasks in rodents. His current research focuses on the neurobiology of decision-making and learning, including neural computation in the midbrain dopamine system, functions of the cortico-basal ganglia circuit, foraging decisions and motor learning. His research combines quantitative rodent behaviors with multi-neuronal recordings, computational modeling, and modern tools such as optogenetics and viral neural circuit tracing.