Abstract:
Dr Rajan and her lab design neural network models based on experimental data, and reverse-engineer them to figure out how brain circuits function in health and disease. They have developed a powerful framework for tracing neural paths across multiple brain regions— called Current-Based Decomposition (CURBD). This new approach enables the computation of excitatory and inhibitory input currents that drive a given neuron, aiding in the discovery of how entire populations of neurons behave across multiple interacting brain regions. Dr Rajan’s team has applied this method to studying the neural underpinnings of behavior. As an example, when CURBD was applied to data gathered from an animal model often used to study depression- and anxiety-like behaviors (i.e., learned helplessness) the underlying biology driving adaptive and maladaptive behaviours in the face of stress was revealed. With this framework Dr Rajan's team probes for mechanisms at work across brain regions that support both healthy and disease states - as well as identify key divergences from multiple different nervous systems, including zebrafish, mice, non-human primates, and humans.
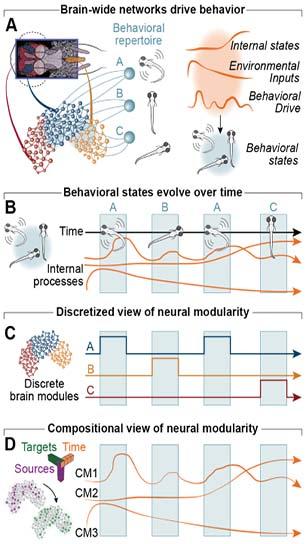
Biography:
Kanaka Rajan, Ph.D. is a Computational Neuroscientist and Associate Professor at the Friedman Brain Institute at the Icahn School of Medicine at Mount Sinai in New York. Her research seeks to understand how important cognitive functions — such as learning, remembering, and deciding — emerge from the cooperative activity of multi-scale neural processes. Using data from neuroscience experiments, Kanaka applies computational frameworks derived from machine learning and statistical physics to uncover integrative theories about the brain that bridge neurobiology and artificial intelligence.