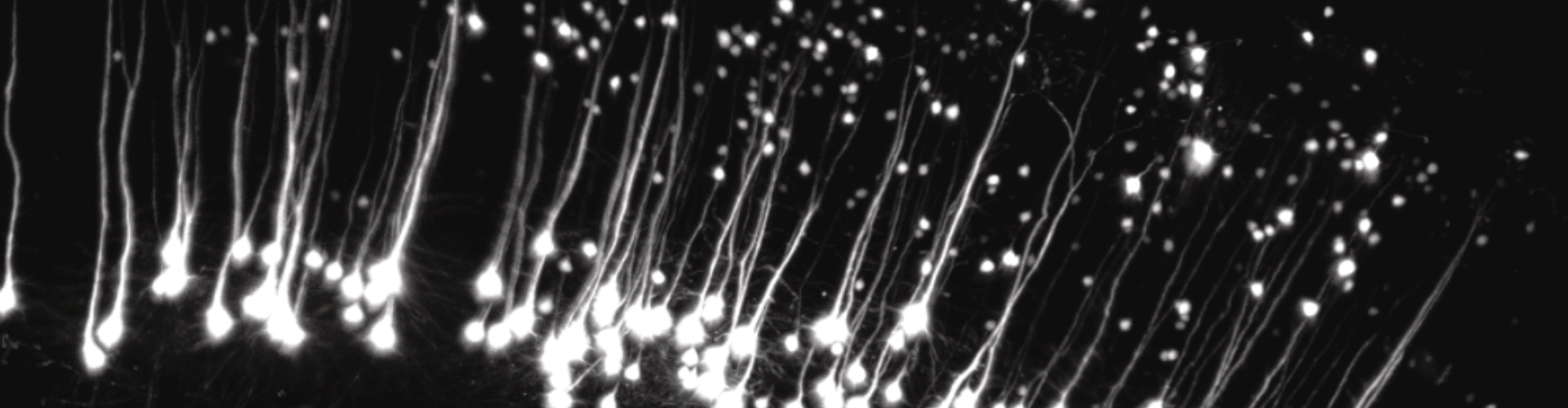
Neural computation of flexible behaviours
Behrens Lab
Research Area
Human behaviour is flexible. Whilst we can act by simply repeating behaviours from the past, we typically use experiences that are only loosely related. We can even imagine the consequences of entirely novel choices. Our research focuses on the neural mechanisms that support this flexible goal-directed behaviour. In doing so, we build new bridges between human and animal neuroscience, between biological and artificial intelligence, and new methods for integrating across scales of neural activity.
Research Topics
How does neural activity represent models of the world to facilitate flexible behaviour?
These internal models underlie human ability to understand the relationships between events and predict the consequences of actions. We know broadly which parts of the brain support these relational world-models, but we have limited understanding of the computations involved or how they are performed by neurons.
How are these representations generalised to new scenarios to facilitate rapid learning and inference?
This generalisation enables humans to make flexible decisions without direct experience. It is fundamental to human cognition. Patterns of cellular activity are abstracted from sensory experience and applied to new situations, but it is unclear how this abstract knowledge is represented or generalised.
Structuring complex behaviour.
How do we build rich plans about the future? The state of the art in neuroscience considers learning and planning over sequences of low-level actions, but humans create, represent and execute complex, heterarchical, plans. Our notion of a foreign holiday includes a plan to fly and rent a car. These representational plans, and our ability to compose them into hierarchies, are central to our rich behaviour, but there is currently no mechanistic neuroscience in this domain.
Building representations for flexible cognition.
How do these representations arise? One critical factor is likely to be rest. Rest is important for consolidating learning, but critically also for new insights. There is currently no mechanistic theory of how this happens. How can knowledge representations be reconfigured in rest to generate new insights and abstractions? How can different abstractions be combined?
Lab members
- Tim Behrens
- Diksha Gupta
- Mathias Sable Meyer
- Will Dorrell
- Jo Warren
- Chongyu (Xiao) Qin
- Lauren Bennett
- Avital Hahamy
- James Whittington
- Jacob Bakermans
- Beatriz Godinho
- Mohamady El Gaby
- Anna Shpektor
- Alon Baram
- Peter Doohan
- Svenja Kuchenoff
- Jiali Zhang
- Adam Harris
- Arya Bhomick
- Emma Muller-Seydlitz
- Daniel Shani