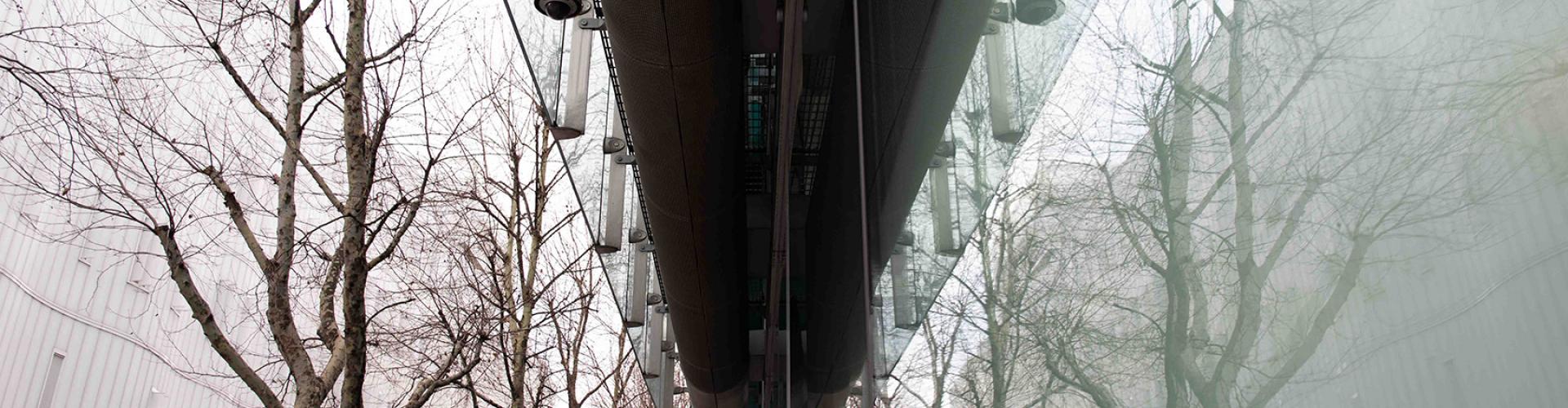
Defining internal tuning-curves for neuronal codes of space
An interview with Dr Alon Rubin conducted by April Cashin-Garbutt, MA (Cantab)
The final Emerging Neuroscientists Seminar Series (ENSS) of 2018 was given by Dr Alon Rubin, a postdoctoral researcher at the Weizmann Institute of Science. I caught up with Dr Rubin to find out more about his research on the internal structure of neuronal codes for space.
What can neuronal tuning curves teach us and what are some of the challenges of interpreting them?
Usually, neuronal tuning curves are used to reveal which aspects of the external world a given brain region cares about. Does it care about visual inputs, the animal’s position, or what point of the body is being touched or moved? In many cases, the importance of a tuning curve is just the question, what is the neuron tuned to? In other cases, it is not only about what, but also about how.
For example, consider hippocampal place cells versus entorhinal grid cells, they both code for space, but they use very different schemes to code for the same property. In this case the internal structure can reveal the differences between the two coding schemes (how), despite the same coded variable (what).
Internal tuning curves do not assume a priori the identity of the encoded variable. For example, if I wanted to ask how stable is the coding of the hippocampus, in the past I would have looked at the tuning curves of the neurons with respect to the position of the animal and looked at how stable they are over time. In order to do so, I had to have the assumption or the knowledge that they care about space.
In contrast, by using the internal structure approach, we can test the stability without making an assumption about what is actually the variable that is coded by recorded neuronal population.
To give an analogy, there is a famous riddle where there is a room with two doors with a guard on each. One door leads to a treasure and the other leads to danger. One guard always tells the truth and the other one is a compulsory liar. You can only ask one of them one question in order to figure out which door is the correct one.
It’s always hard because we tend to assume we need two questions, one to figure out which guard is the truth-teller, and then the second to know which is the correct door. The trick is to ask one of them, “What would the other guard say if I would have asked him?” and then do the opposite.
The interesting point here is that you can find the right answer about the door without actually knowing who is telling the truth – you’re bypassing the need for this knowledge. In the same way, we can bypass the need to know what is the encoded variable and directly test the stability.
Although internal tuning curves eliminate the need for prior assumptions regarding the coded variable, while interpreting the classical external tuning curve is usually straightforward, it is much harder to look at an internal tuning curve and understand what it is the neuron tuned to. In a way, it is impossible to infer the semantics of the code just from the code itself. For that you have to look at its correlation to the external variables.
However, the internal structure may provide you with some hints for the features of the encoded variable. For example, say, I don’t know what is encoded here, but I see that it is a circular variable that moves continuously in a timescale of hundreds of milliseconds. This could suggest head direction and may give you a hint next time you record from this brain region of what external variable to look at.
Why does measuring neuronal tuning curves require a priori assumptions?
You have to choose what is the external variable you are contrasting or correlating with the neural activity. First, you have to have a knowledge or a guess about that, and second, sometimes, it might be tricky to interpret the data in this way if you use it too naïvely.
For example, consider neuronal synonyms: different neuronal activity patterns that have the same meaning. If you interpret too naïvely, you just average over the two synonyms and get something that is neither of the two. If you look at the internal structure and see that you have two different descriptive states, then you can see that they have the same meaning or semantics.
How did you apply unsupervised learning to uncover the internal structure of neuronal codes for space?
I used unsupervised learning, specifically a method called Laplacian eigenmaps, which is a nonlinear dimensionality reduction.
In many cases, people use linear dimensionality reduction, which may work in very specific cases, such as when the tuning curves are wide. But when things are narrower, it is much harder to understand. When we have this structure in the reduced space, you can study the dimensions, the topology, or the temporal trajectory of the state of the network within this set structure.
This kind of analysis is unsupervised, because you don’t have any labels. You don’t have a teacher that tells you “All these are A, and all those are B.”, instead you just look at the data itself and say, “Okay, I see there are two clusters, two states.”
What did this study reveal about place-tuning and head-direction tuning and how did you expose a previously unknown variable?
We used the head-direction tuning and place tuning as a proof of concept, that a known feature of the neural data can be revealed using this approach. However, when we looked at the structure of the data, we could see the data itself is clustered or segmented to different neural states. This, in a way, released us from the need to predefine the different behavioural states and to identify the transitions between them.
For example, we didn’t have to set a threshold and say that the mouse is running if and only if its velocity is above the threshold. Instead, since some of the neuronal states seem to be associated with running, we can base the behavioural labelling on the neuronal population activity.
The motivation for the data analysis of more frontal areas, specifically, the area called the ACC, was to demonstrate how we use this method even in cases where no canonical variable is associated with the brain region of interest. I think this method is mostly useful when studying a brain region where it is not clear what code it supports.
Was the internal structure conserved across mice?
Yes, at least in some cases. We showed it in two mice that were trained to do the same task and performed the same task. This allowed us to learn something about the code from one mouse and apply it to another mouse, a task which is trivial in the case of creatures like C. Elegans, and maybe flies, where there is one-to-one mapping between neurons of different individuals.
In the case of mammals, and specifically for their hippocampus, it is impossible, because there is no one-to-one mapping, and even no organization that we have to do with anything within the hippocampus. Here, if the structure is similar, we can learn what is the meaning of different parts of the structure, and based on the similarity to the structure of another one, we can export this understanding to another individual and understand the meaning of each state in that individual.
What is the next piece of the puzzle you are trying to understand?
The main goal is using this method to understand the coding of additional brain regions.
In general, I think there is a need for a shift from defining the functionality of a brain region through the identity of the variables it is coding to viewing it through the computational process it performs.
It may be that under different conditions a given brain region would use the same type of neuronal computation to process different types of information. Thus, maybe the relevant question is not which variables are represented, but what is the computational process the circuit is performing? In this sense, the internal structure may be a better fingerprint of the functionality than the external correlation, which may differ across setups or contexts.
For example, a couple of years ago, researchers from Princeton demonstrated that the hippocampus, a brain area that is known to be associated with navigation and to code position in space, is tuned to the tone of the auditory signal during a task of navigating in the auditory space. In this case, the hippocampus coded a different external variable (tone instead of position), but the coding scheme was similar and may underlie a realization of the same computation process.
Another example is a device that was developed at the Hebrew University to aid blind people. The key idea behind this device is translating visual signal to an auditory signal. Interestingly brain activity of patients who used this device suggested that the auditory signal is processed in the visual area.
This result could be interpreted as the visual area does not simply process the information coming from photons hitting the retina; instead it processes information with some type of structure, regardless of where it comes from.
Maybe we should move as a field from asking, “What variable?” to asking, “What computational process?”
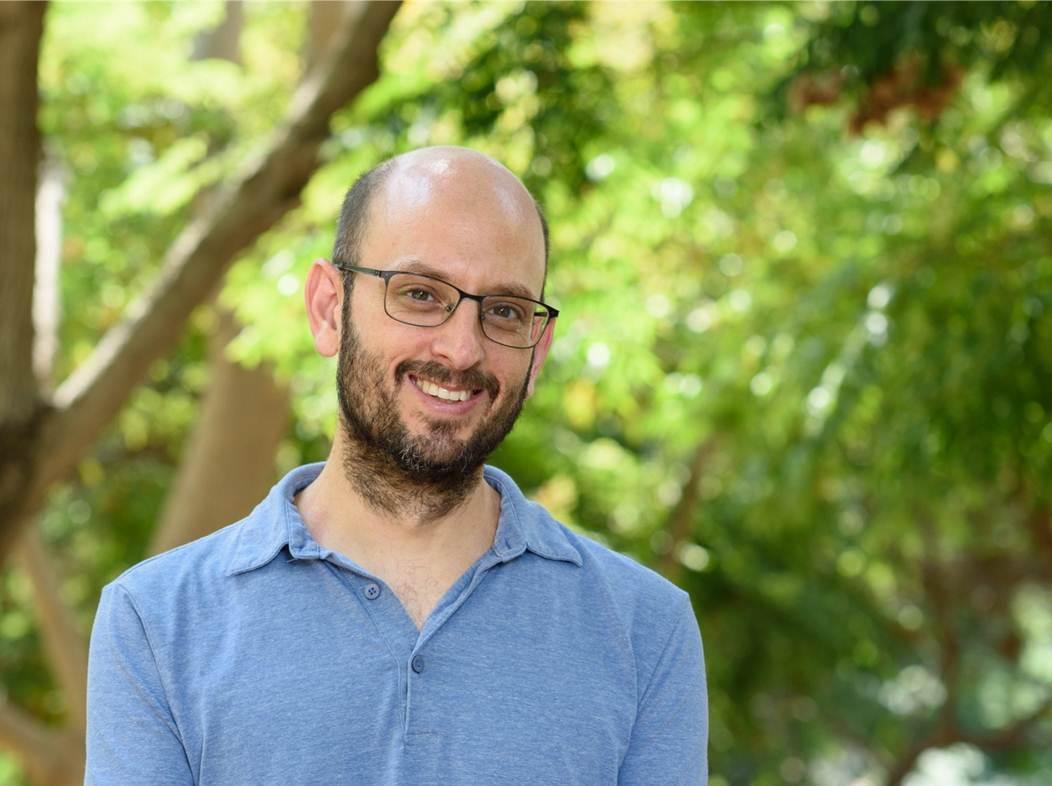
About Dr Alon Rubin
I did my undergraduate studies at the Hebrew University of Jerusalem in physics and cognitive science. I then studied at the Weizmann Institute of Science, where I completed my MSc under the supervision of Prof. Misha Tsodyks and PhD under the joint supervision of Prof. Misha Tsodyks and Prof. Nachum Ulavovsky.
Throughout my research I applied both theoretical modelling and electrophysiological experiments in behaving bats, to study the neuronal code within the hippocampal formation. I then joined the new laboratory of Dr. Yaniv Ziv, where we use novel optical imaging methods which allow longitudinal recording of neuronal activity from large neuronal populations in freely behaving mice. I am particularly interested in advanced analytical paradigms that are now applicable due to the constant up-scaling in numbers of simultaneously recorded neurons.