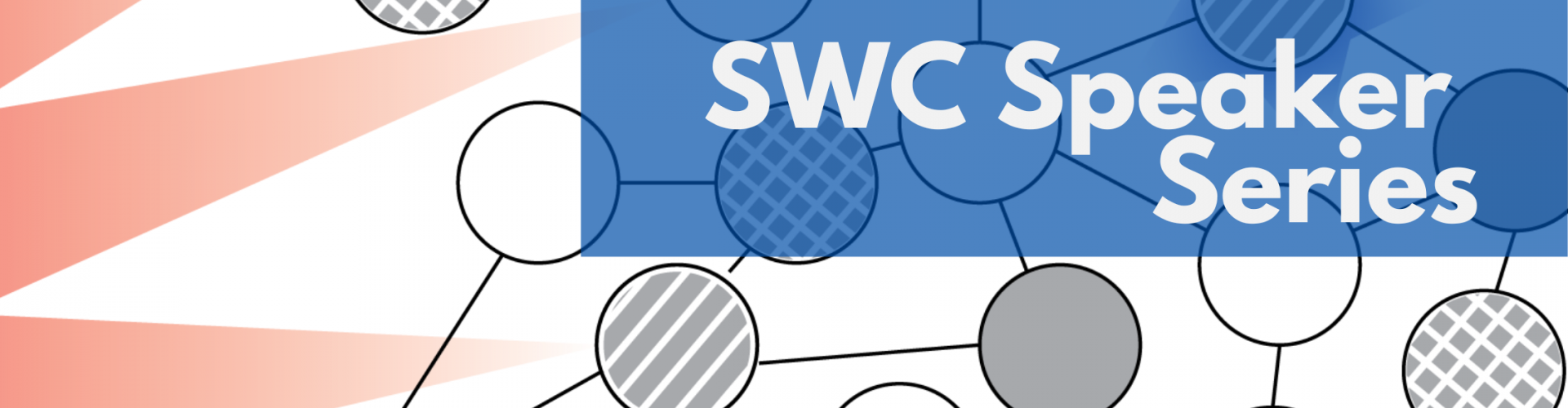
Forcing behaviour in a handful of neurons to understand learning
An interview with Dr Nuria Vendrell-Llopis, University of California, Berkeley, conducted by Hyewon Kim
Can you willingly activate a handful of neurons in the brain? What implications does such neural reinforcement have on the use of brain machine interfaces? In a recent SWC Seminar, Dr Nuria Vendrell Llopis shared her work on neural reinforcement across brain regions and cell-types. In this Q&A, she expands on what motivated her to pursue this line of research, her key findings, and implications of these findings on how we learn.
What made you interested in researching neural reinforcement in the brain?
I had always been interested in it since the moment I transitioned to neuroscience. I am an engineer by training and at some point, I realised that if I wanted to study the most powerful machine, I didn’t have to go to normal computers, I had to study the brain.
It was a switch from thinking, ‘Computers are the best because they can do everything and can learn something that humans can’t’, to realising that is actually not true. Machines have a long way to go. Even deep learning nowadays actually recreates the way the brain learns.
I came to neuroscience with the idea to study how the brain identifies the neurons that are driving a behaviour and how the brain reuses and refines the neuronal patterns that drive that behaviour.
How much has already been known about this topic?
There are two different aspects to what is known about neural reinforcement. One is how long we have been using only a handful of neurons to achieve neural reinforcement and the other is that under each refinement of a behaviour, there is also a refinement of neural patterns.
Starting with the second aspect first, there has been a long road with many small incremental papers showing this over time. Whenever there is refinement of a behaviour, there is also refinement of the neuronal patterns underlying that behavior. In 2016 Peters and colleagues showed that refinement in motor output and in neuronal patterns happen simultaneously. Neural reinforcement had been shown before during neuroprosthetic learning , but this was the first time it was shown specifically in a normal motor behaviour.
As for the first aspect, it was in 1969 that Fetz was able to train a macaque to increase or decrease neural activity in order to obtain reward The advent of brain machine interfaces (BMIs) was in the early 2000s with the work of Nicolelis, Donoghue, etc. But there is this huge gap of 40 years between then and the 60s.
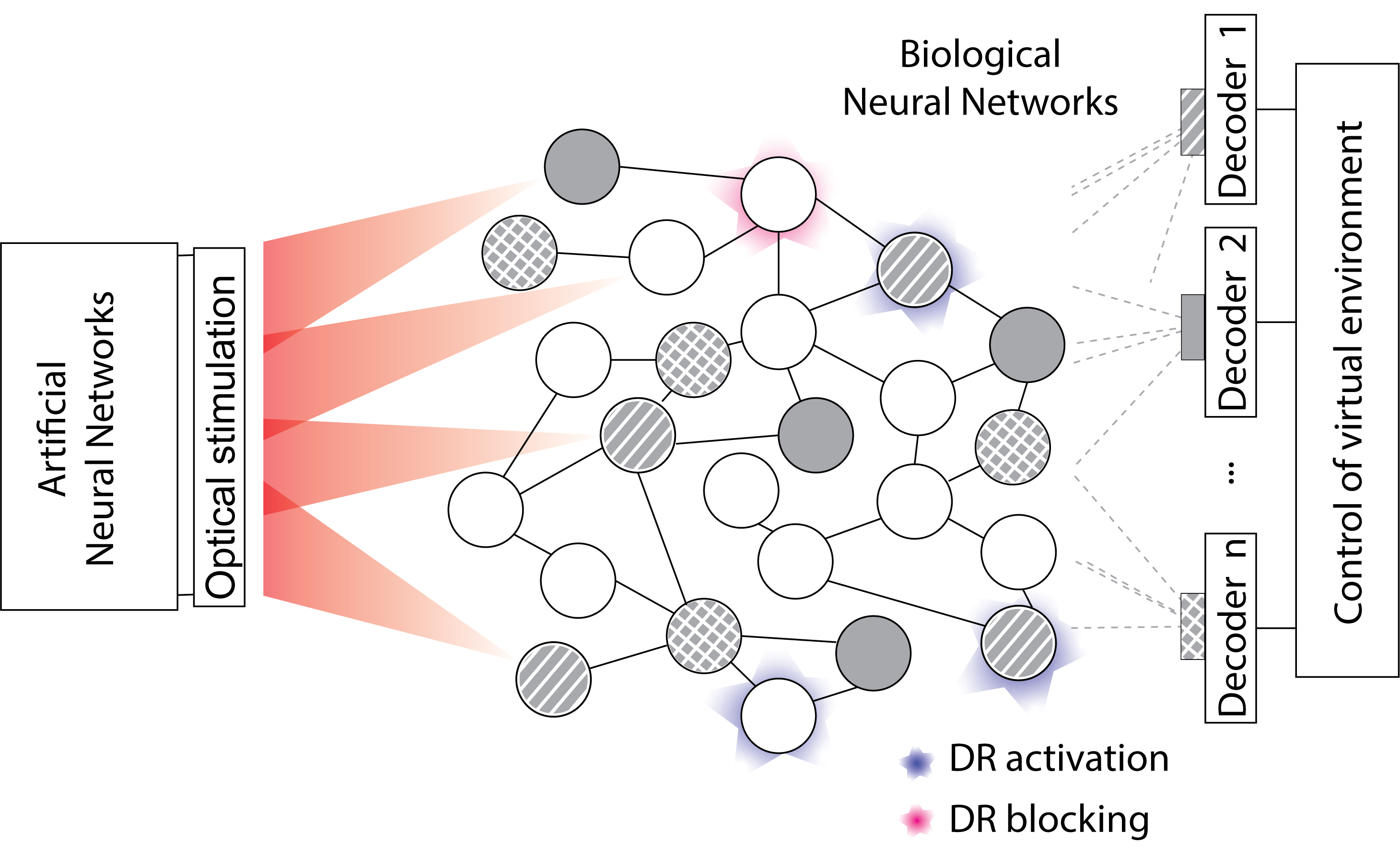
How does your research try to understand the role of cortex and striatum in neural reinforcement?
One of the most complex things about studying the relationship between cortex and striatum is that there are many neurons in both areas. Those neurons may be doing many different things that are unrelated with the behaviour we are interested in.
Whenever we take any action, like grabbing something or driving, there are neurons that are generating that behaviour. However, when you move your hand, you see your hand moving. This visual information also comes into your brain and acts as feedback. It’s difficult to separate which neurons are bringing in sensory information, which neurons are bringing in motor information, and which neurons are driving the behaviour.
The way I go about figuring this out is not to try and find those neurons among all the millions of neurons that have behaviour related activity. Instead, I choose a small group of neurons and force a behaviour in them. I link a behaviour to the activity of those neurons. For example, If those neurons increase their activity, the cursor (artificial movement) moves accordingly. So, that way, I can limit the circuit to study – instead of having millions of neurons with trillions of connections with each other, I have a small number of neurons that I can identify because I have selected them. That allows me to have a small circuit, a circuit that I can control.
How did you build your model and in what ways did your model inform how the data explained your hypothesis?
The ultimate thing we wanted to study was how each of the different variables that can interfere with or impact learning, were impacting the model. The problem we were having was that it was very difficult in experiments to separate different variables that affect the same output. For example, I am a woman and because I am a woman, I am shorter than the average man It is going to be difficult to study how sex or height affects a third variable because you cannot separate me being a woman from me being not as tall as the average man.
To separate clearly dependent variables and see how they would interfere with learning, we used a machine learning model to recreate how the brain may be interpreting each of those features that were impacting the learning result. Now that we had that model recreating the learning performance, hopefully with the same influence of each of those variables, we could try to explain how each of those variables impacted the model and by doing that, explain how those variables impacted learning.
What have been your key findings so far? How do your results in cortex compare to those in striatum?
Our key finding is that there are different populations of neurons in cortex and those populations experience neural reinforcement differently. It is much easier for the brain to reuse and refine the neuronal patterns from a particular cortical population – pyramidal tract (PT) neurons – than it is to reuse and refine activity of the intratelencephalic (IT) neuronal population. There is a difference in the cortex between these neural populations and in how the brain can control them.
We know that in learning any task that is goal-directed or reward-motivated, there is a connection between cortex and striatum. Striatum is key to finding which neurons are being reinforced and how to reactivate those neuronal patterns, reuse and refine them. But we didn’t know exactly how. So we looked at how the activity of the cortical neurons influenced the activity of the striatal neurons. What was the function of those striatal units?
What we found is that striatum takes the cortical activity that we know is moving the artificial cursor and gives it a value, an internal representation of this cortical activity, indicating whether I am far away from or close to the reward. This is the information the animal wants to get because that is what will get the reward. We believe the striatum is doing that – making an internal association between what cortex is doing with what the animal is getting externally.
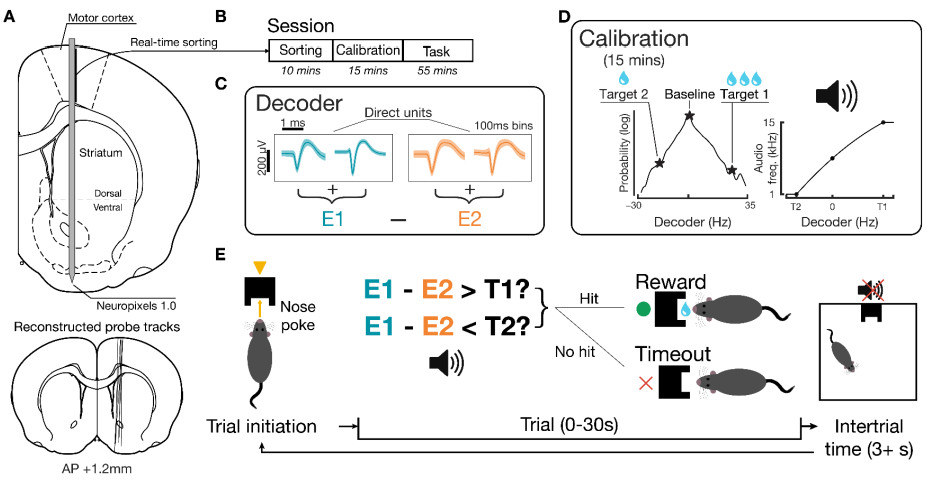
Rats learn a neuroprosthetic task driven by motor cortex units via an increasingly consistent neural strategy. (A) Targeted chronic implant trajectory, Neuropixels 1.0 probes in rats (n=7). Bottom: probe tracks via histological imaging confirming desired positioning. (B) Phases of daily training. (C) Example mean waveforms (shading ± 1 SD) of direct units and decoder calculation. (D) Decoder targets and its mapping to auditory tone according to calibration period. (E) Task structure. Source
Were you surprised to find that the striatum gives value to the cortical activity to indicate proximity to reward?
In a sense, yes. We were expecting to see a shift in the striatum, not only taking information from cortex, but also from the influence that the cortical activity had on the environment. When I move my hand and touch something, there is an effect of my actions on the environment. What we expected to see was that the ventral striatum would first represent that effect on the environment, then slowly change to only represent the cortical activity that created those changes.
And it turns out that is not what is happening. From the very beginning, the striatum did not care about what was happening in the environment, it only cared about what was happening in cortex.
What implications do your findings have for humans?
I think the general implication is for understanding how we learn. At the basic neuronal level, even though the human has 100s of millions of neurons, the concept is still the same. It is the way we learn a new action, or any kind of behaviour that leads to reward, like learning how to play Donkey Kong or any video game. You just do it because it’s fun! You want to keep doing it so that you get better at it. The cortical patterns that control the joystick, or how fast you type on the keyboard if you are playing on a computer, all arise from starting slowly at first with your movements being very sharp and unrefined. Eventually, you get better at moving the joystick or mouse.
It’s a general basic understanding of how we learn multiple different behaviours that, ethologically speaking from an evolutionary point of view, made us who we are today. If we didn’t learn to find the fruit that gives us reward, or didn’t escape the dangerous animal, we wouldn’t have evolved the way that we have.
I also want to apply these findings to BMIs, which we can use to help people with motor deficiencies. If we can learn how the brain learns, we will be better able to help people that use these BMIs to learn tasks like moving a motor wheelchair just by the activity of a handful of neurons.
What will the work in your lab focus on?
BMIs use the concept of neural reinforcement, a small amount of neurons controlling an abstract neural cursor or a physical object like a robotic arm. In the same way, I believe we can use BMIs to help people who suffer from cognitive dysfunction. It’s as simple as better understanding how the brain learns so that we can use those same strategies to help people with cognitive dysfunction learn better.
What I envision in my lab is to discover how the brain learns, and use that knowledge to create better BMIs and help a broader population, including people with cognitive deficiencies.
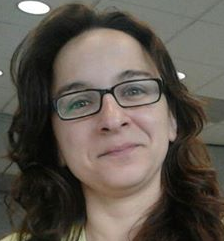
About Dr Nuria Vendrell-Llopis
Nuria Vendrell-Llopis is a postdoc fellow at UC. Berkeley who studies the mechanisms underlying neural reinforcement. She received the equivalent to a B.S. and M.S. degrees in Telecommunication engineering from the Polytechnic University of Valencia (Spain) in 2010. Following those, she received a Ph.D. degree in Biomedical Science from the KU Leuven (Belgium) in 2015 and was the recipient of a long term EMBO fellowship from 2015 to 2017. Her ultimate goal is to understand how neural reinforcement drives learning and to leverage this knowledge to establish a new experimental protocol that could artificially enhance or imprint new skills.