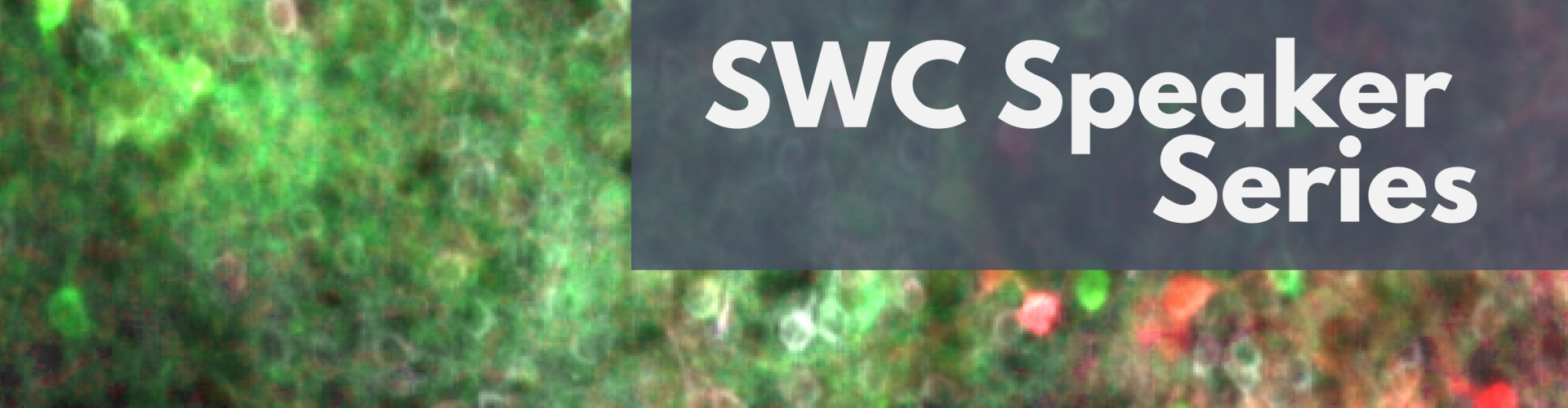
How do internal states shape learning?
An interview with Professor Sami El-Boustani, University of Geneva
Decision-making offers a valuable window into the intricate ways internal states and internal models shape our behaviour. In a recent SWC Seminar, Professor Sami El-Boustani presented findings that elucidate the cognitive mechanisms underlying sensorimotor learning under varying motivational states. In this Q&A, Professor El-Boustani shares his journey from studying synaptic plasticity to exploring the interplay between motivation and learning, and the importance of understanding cross-modal generalisation.
Why is decision-making a particularly insightful process to study when exploring how internal states and representations shape behaviour?
When you're trying to get a sense of how motivated someone is, or what emotion someone is experiencing, there are a lot of visible clues. For instance, if you're afraid, your face might show it. But what I observe might not be exactly what you are experiencing. The relationship between visible behaviour and cognition is a tricky one.
Decision-making is a way to see an animal display certain behaviours that might reflect the cognitive processes that we're interested in. That being said, decision-making might not be the only behaviour that can do this. Classical conditioning, like fear conditioning, are also revealing.
But the more you get into the details of how the brain builds a rich representation of the world, the more you require situations in which animals are facing complex decisions.
How are you unravelling the interplay between motivational states and learning?
Initially, this is not what we were interested in! At the beginning, the lab was trying to understand the nature of synaptic plasticity when one has to learn something new. By something new I mean a sensory-motor association that can be as simple as pressing a button when you see a red light.
I come from a theoretical background, and I studied mechanisms of plasticity during my PhD. The simple tasks we used to train our mice could in principle be learned extremely quickly, even by a simple network model. Yet, when we studied this behaviour in mice, we realised that the learning time was way longer than what we expected. Not only that, but other studies show that depending on the condition and the stressor or the reinforcer, you can observe faster or slower learning.
So that brought us to understand that maybe there's another factor that could affect learning trajectory.
Some scientists refer to this distinction as the difference between apparent learning and proper learning. This means what is really learned versus how it's manifested in behaviour. This is where motivation plays a big role, because we’ve known for quite some time that motivation can completely change the expression of a learned behaviour.
There is a century-old law called the Yerkes-Dodson law that describes how performance in different tasks can be completely affected by the level of arousal or motivational drive that an animal is experiencing. For us, this was interesting to probe from a neural circuit point of view, as the tools available today allow us to do that.
Many labs including our use negative reinforcement to train their animals. This comes with a price – it creates motivational drive that can be too high and therefore can lead to apparent learning that does not reveal what the animal really understands of the task. This is another reason why we decided to explore this phenomenon more in detail.
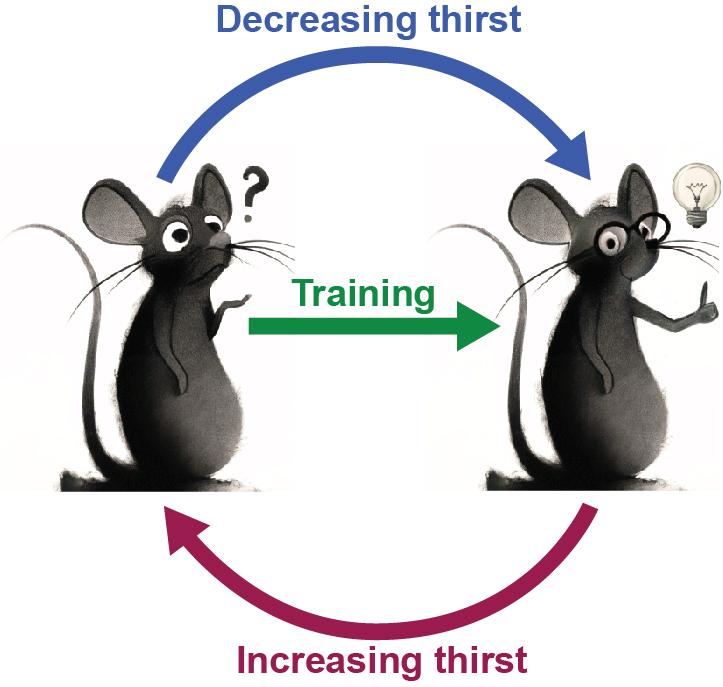
What have been your key findings so far?
Our key findings are that mice are dealing with different forms of learning. On the one hand, they learn sensory-motor associations. The animals don’t have any prior understanding of how the task works and they discover it by trial and error.
On the other hand, they are learning to adapt to situations or contexts that are more stressful for them. With limited access to water, they are in conditions that are not their usual ones, and so they also need to adapt on that level.
We see these two processes acting at the same time. We’ve learnt that we need to pay attention to both and try to factor one out if we want to study the other one.
What we're planning to do now is get rid of the motivational aspect to focus more on the mechanisms underlying pure sensory-motor learning.
Do computational models support your results?
Initially, we tried to explain this phenomena using a relatively simplistic approach. We decided to model it more in-depth to give a clearer understanding of how motivation and sensory-motor learning play together. We can define learning trajectories that are specific to one mechanism or the other, and the model highlights how they interact.
We're planning to push this further and use the model to identify specific behavioural traits, or personality traits, in individual mice. We will also try to adapt our behavioural training strategy to individual mice, as they all react differently to these tasks.
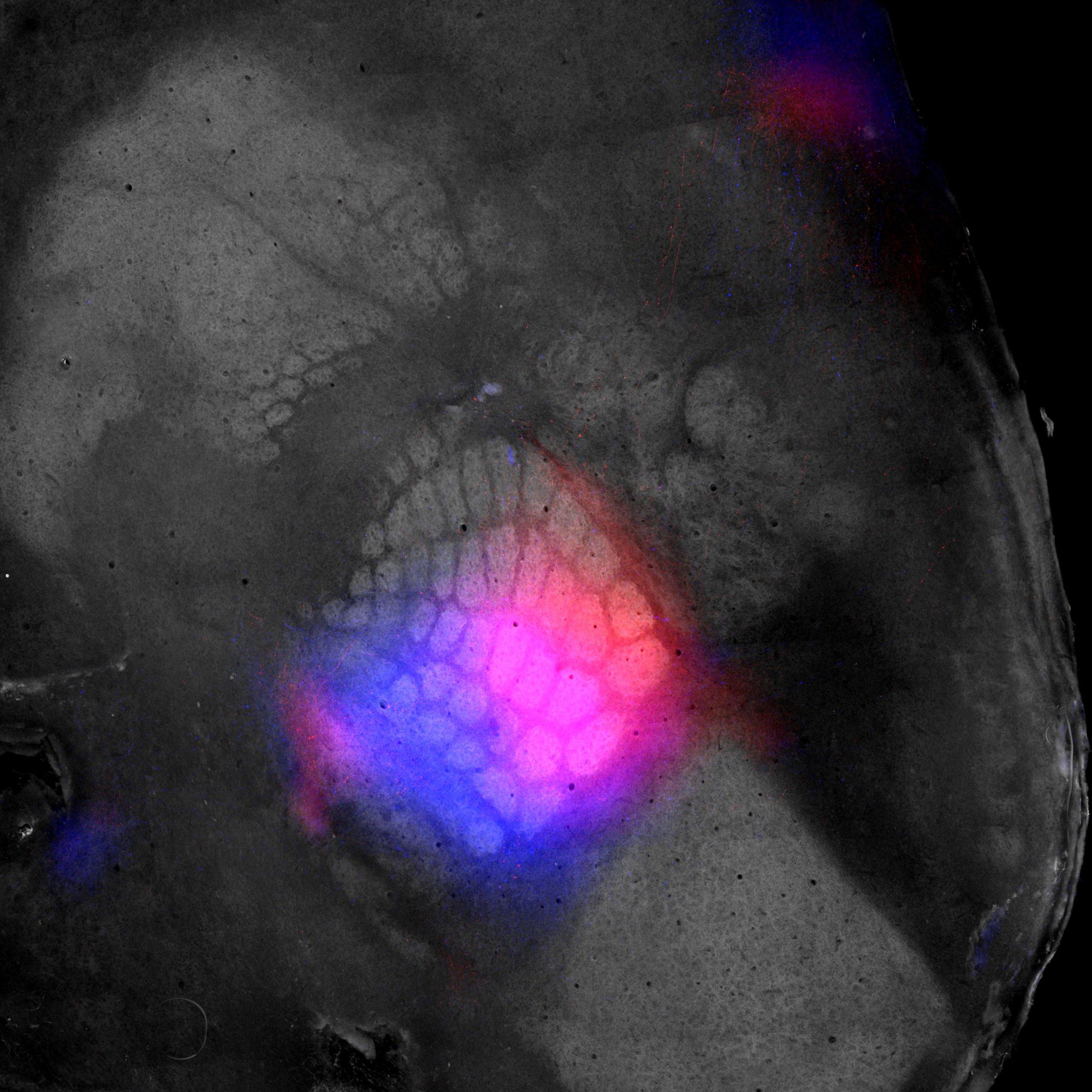
Reconstructed cortical surface obtained post-mortem using a flat-brain preparation. The primary visual and somatosensory cortices are highlighted via M2 muscarinic acetylcholine receptor immunostaining. Fluorescent proteins, shown in blue and red, are expressed in distinct regions of the barrel cortex, along with their axonal projections, which preserve the somatotopic organization across the cortical sheet. Credit: Sami El-Boustani.
Could you tell me more about how you're exploring cross-modal generalisation?
As many neuroscientists, we are interested in understanding how our senses communicate with each other. We are often confronted to situations where we need to adapt to a dramatic change in sensory conditions. For instance, you're looking for your remote control and you see that it's under the couch, but you can't get it easily while you’re still looking at it.
So, you reach under the couch with your hand and, once you grab the object, you immediately recognise whether it's a remote control or something else. This is all done within a few seconds as you're going from purely visual information to purely tactile information. You're able to transfer that information across sensory modalities without even realising it.
The question that sparked our interest was: how does the brain construct an abstract representation of the world, independent of any single sensory organ? This is what brought us to cross-modal generalisation.
Thinking about peri-personal space – the space within reach around your body – an object in that space can evoke a stimulation that is sensed by both your eyes and your hand. But your brain knows that it's the same object in that same position in space. We see that mice do this generalisation in the same way.
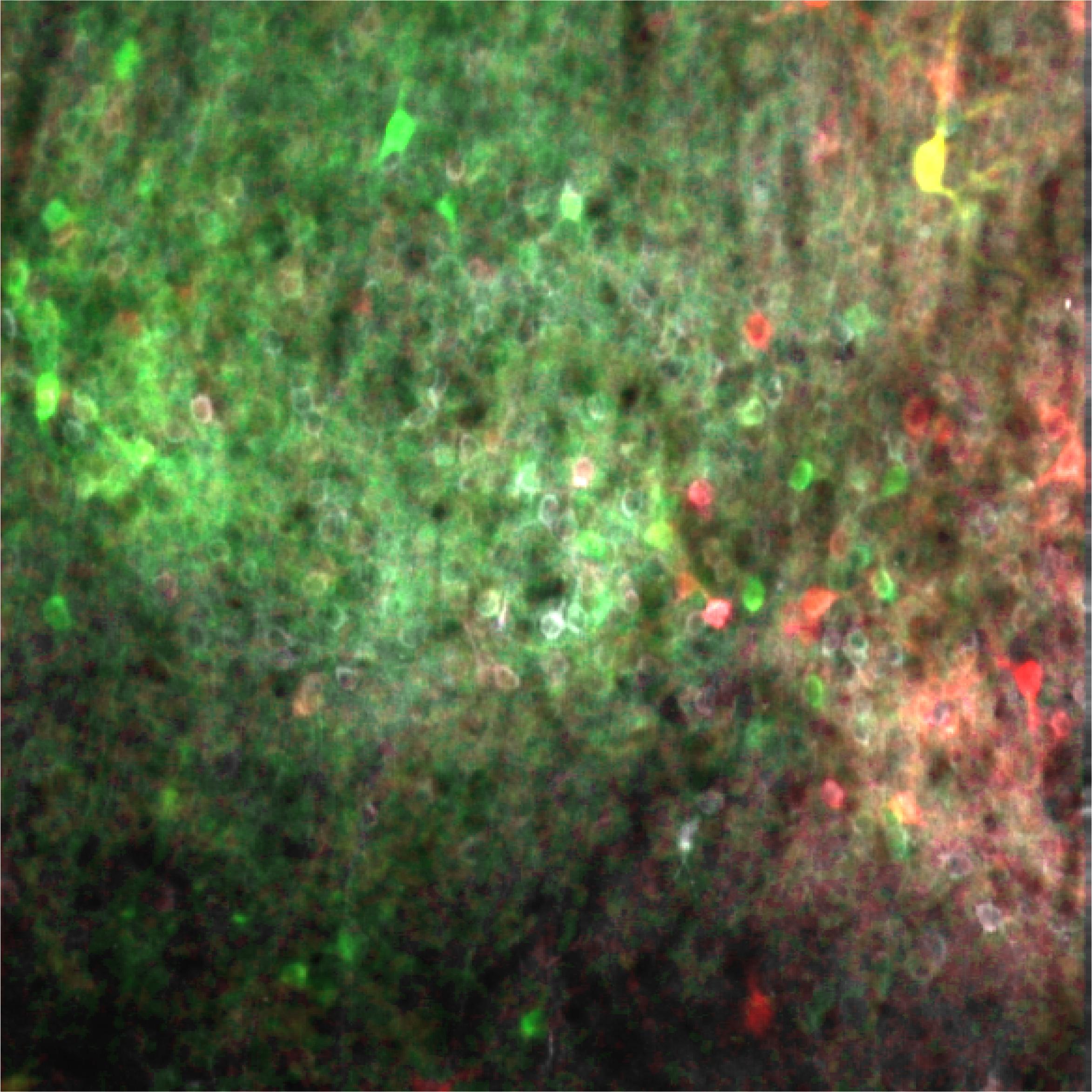
Two-photon calcium imaging across cortical layers of the mouse whisker primary somatosensory cortex during a two-whisker discrimination task. The colour code indicates whisker preference: green neurons respond predominantly to the C2 whisker, red neurons to the B2 whisker, and yellow neurons to both. The spatial segregation of neurons with different preferences highlights the somatotopic organization of the barrel cortex. Credit: Sami El-Boustani
Why is it so important to understand how the brain integrates and generalises information across the different modalities?
It is a mechanism by which we can adapt our behaviour to complex or changing situations. If everything you learn was attached to the specific situation where you learn it, you wouldn't get very far.
Being able to observe and recognise certain patterns and map them together whether they are learned, abstracted, or they are anchored in the way your brain has developed is fundamental. It allows us to be able to change or produce the right behaviour, even if the conditions are not exactly the same.
What's the next piece of the puzzle that you're hoping to solve?
That's going to be the question we were initially trying to answer! We really want to understand where, when, and how synaptic plasticity occurs during sensory-motor learning. There are many different areas between cortical and striatal structures that presumably are experiencing forms of plasticity.
The hope is to deploy a set of new techniques that allow us to identify exactly where this is happening via brain wide mapping of plasticity. We are also trying to understand the precise time window when proper learning is taking place. The goal is to understand the neuronal underpinning of goal-directed sensory-motor learning.
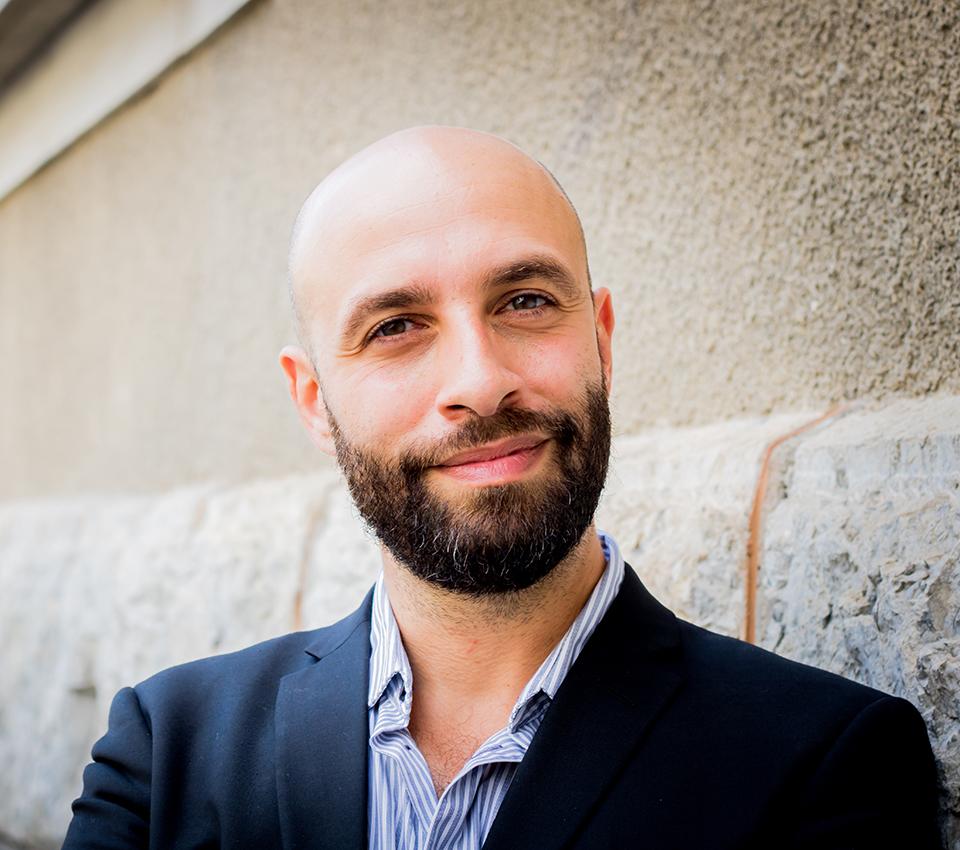
About Professor Sami El-Boustani
Sami El-Boustani is an Assistant Professor in the Department of Basic Neurosciences at the University of Geneva. After earning a Master degree in theoretical physics from EPFL (Switzerland), he pursued a second Master's in Cognitive Science from l’Ecole Normal Supérieure in Paris (France). He then completed his Ph.D. in neuroscience at Sorbonne University, focusing on sensory processing and plasticity in cortical networks. Following his Ph.D., Sami conducted postdoctoral research at MIT (USA), studying interneurons and dendritic plasticity, and later at EPFL, exploring brain circuits in the mouse whisker system. In 2019, he opened his lab at University of Geneva after being awarded a SNSF Eccellenza fellowship.