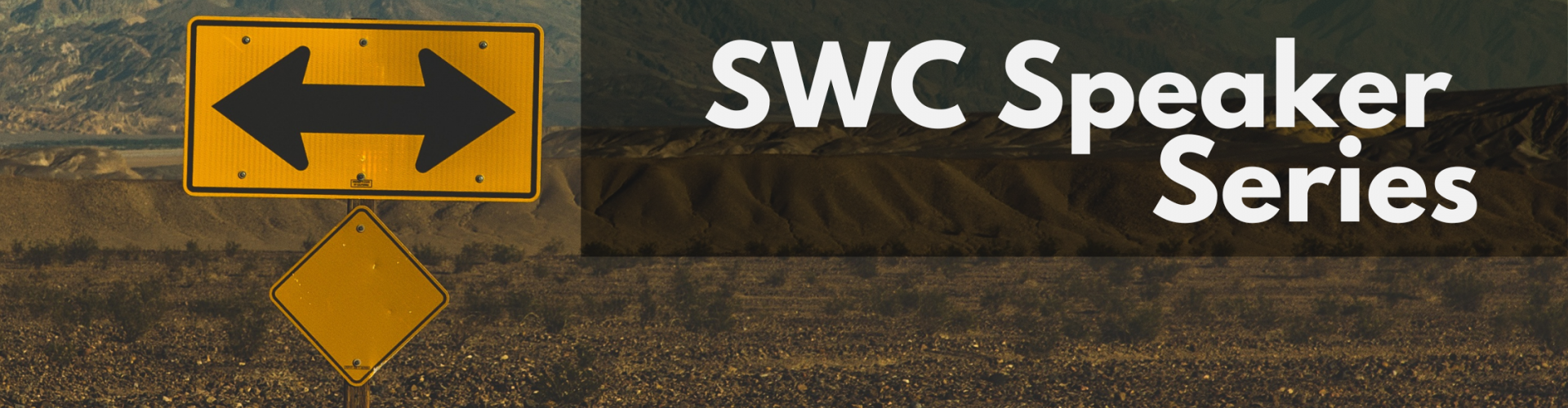
Learning from models of suboptimal learning
An interview with Professor Peter Dayan, Max Planck Institute for Biological Cybernetics, conducted by Hyewon Kim
Modelling the solar system with a grape, orange, and watermelon comes close to representing the relative scale of the planets, but it’s bound to leave out key details. In the same way, modelling a complex behaviour like learning can also leave out some aspects of the data. Professor Peter Dayan recently gave a seminar at SWC where he shared his work on modelling the behaviour of subjects in a couple of well-established experimental tasks. In this Q&A, he goes into more detail about the models he uses and the role each one plays.
What first sparked your interest in modelling the learning and decision making process?
Much of what we do over our entire lives is making decisions over time. To make better decisions, we have to learn – from our successes and failures, and also from just what happens. This is such a broad area that many different fields of science: economics, operations research, control theory, reinforcement learning, AI, and so forth are all answering very similar questions, and so there is much to understand from them all.
Why is it important to understand what happens during the course of learning rather than just at the end?
There are two reasons. One is a very prosaic one, which is that the way subjects perform the task at the so-called asymptote may be informed by the path they took to get there. It's therefore important to understand the path they took to get there because that will help us understand the normal behaviour we see.
The other reason is that much interesting adaptation is happening over the very early part of learning. From when they start, the subjects have to understand what they should pay attention to in the task. How can we understand the way that they understand the rules that govern the environment?
In many environments, you don't know what it is you could learn. So you perform a much more open-ended and richer exploration in order to engage with a new problem. And that is extremely fascinating.
It's a fascinating question also just from a scientific curiosity point of view. We've focused deeply on the micro aspects of learning, which are very important to think about.
How much is known about how humans and animals learn a behavioural task?
On one hand, a lot is known. On the other hand, little is known.
In terms of how a lot is known, we have in animals a wealth of deep knowledge about shaping training protocols. We know quite a lot about training animals in artificial settings to behave in particular ways, for example. People have a lot of intuition about how animals should go about learning.
For humans on the other hand, it would be excellent, for instance, to optimise education. But if you look at the sort of educational software that my kids were using when they were young, it seemed that the software is still unable to target individual differences in a very precise way. Can it find out how one particular person is learning? Does it know exactly what information it needs to provide a student at exactly what time? It certainly didn’t seem nearly as good as I hoped.
This is a big, important field in psychology as a whole, but I think we’re far from finding optimal ways of understanding and adjusting to individuals so that we can exploit our knowledge to help people learn things more quickly and effectively. There's still a lot to be discovered.
You focused on the different behavioural paradigms that already exist. For example, the International Brain Laboratory (IBL) task for mice and then the spatial alternation task for rats. Why did you focus on these tasks?
The focus of a task is a mixture of expedience and the intrinsic ability of a task to reflect some phenomenon that you're interested in. So in the case of the IBL task, since it's such a huge consortium which involves many neuroscientists, including investigators at the SWC and Gatsby like Tom Mrsic-Flogel, Sonja Hofer, and Peter Latham, we have an unparalleled opportunity to understand the course of learning at scale. That is, it is one of the few projects where we have data from many different mice who are learning exactly the same task according to exactly the same protocol. So all the issues about reproducible science don’t go very much into the learning at IBL. We have 700 choices per day for individual mice and one learning curve per individual because each mouse only does it once. So if you need to get the sort of data to understand learning, it’s phenomenal to have access to a data set with more than 100 animals doing exactly the same thing.
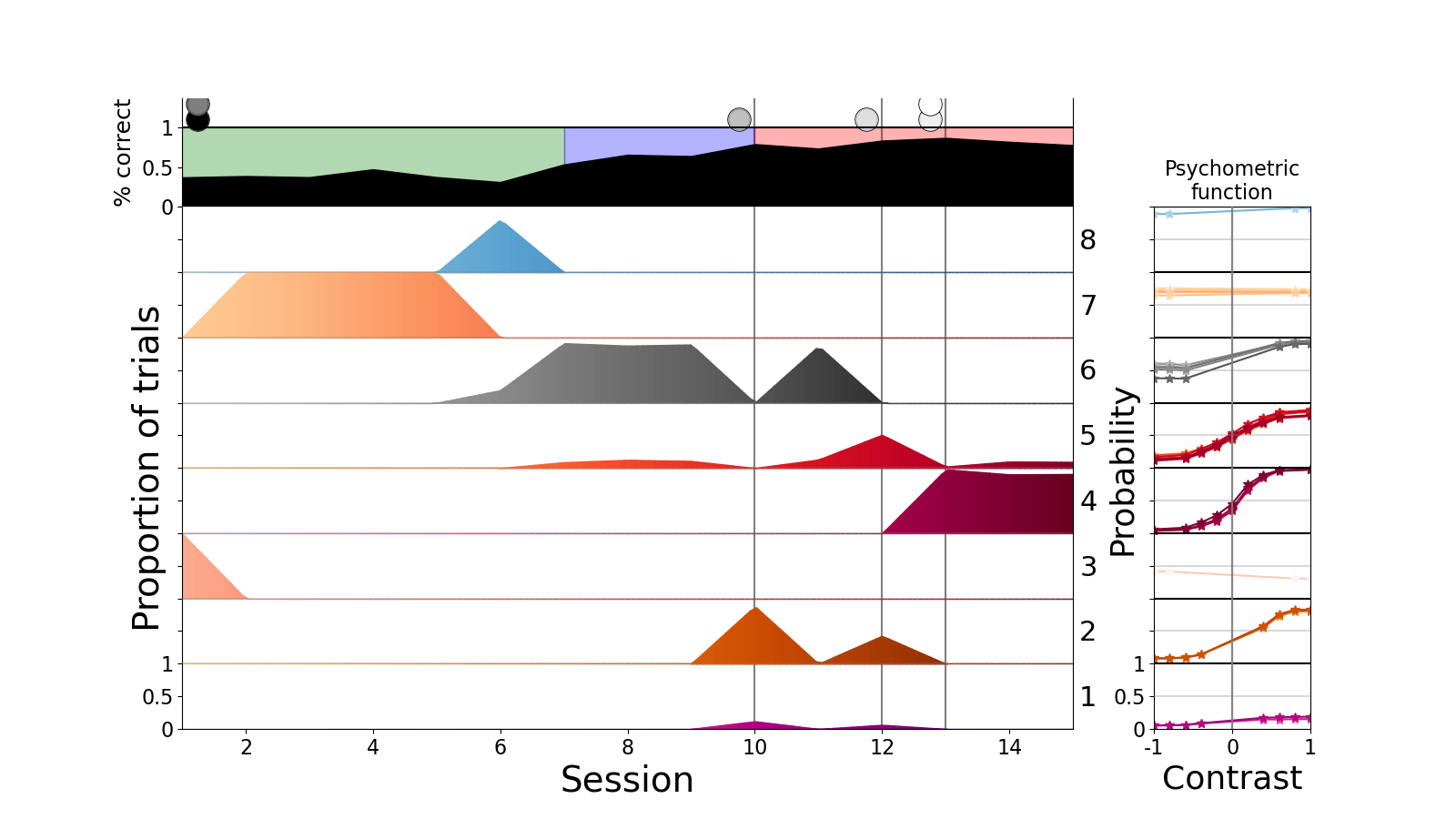
As for the spatial alternation task, Loren Frank and David Kastner had started on this before I was ever involved. This is also a case in which David had collected data from very many animals. In its simpler incarnations, the task had once seemed to involve just simple spatial alternation. However, what David showed very nicely is that this is far from the whole story in his richer and more complex version. The task has duly revealed some really interesting characteristics about learning. One of David’s brilliant innovations was to have an early exploratory phase in which the subjects are rewarded almost no matter what they do. During this almost free-choice phase, the subjects exhibit their intrinsic biases and priors; and we could show how these persist into later, more constrained behaviours. In fact, we didn't know it at the time, but in retrospect the task had many features that were perfectly suited to study the course of learning.
Could you also speak about the alternating serial reaction time (ASRT) task for humans?
The ASRT task is administered to human subjects, who are, at least from our perspective, often lamentably proficient at picking up on contingencies explicitly. So although that's important for learning, it's a very different sort of learning from the sort of acquisition of skilled performance that I was discussing.
However, in the ASRT task, even after thousands of trials, humans lack an explicit understanding of what the actual contingencies are. To explain, in a serial reaction time task, you're presented with a series of let's say, four symbols, each attached to one key or button. Subjects see a sequence of symbols and have to do nothing more than press the associated key. The trick is that there are regularities (and occasional violations thereof) in the sequence. Subjects pick up on those regularities, and are faster and more accurate at pressing when the sequence follows the pattern. What happens in this ASRT task is that alternating symbols are either part of a deterministic pattern (here, with 4 components) or a random symbol between 1 and 4. So let's say it goes: 1, random, 3, random, 2 random, 4, random and then back to 1 , random, etc. The fact you have these random symbols in the middle apparently prevents subjects from gaining an explicit appreciation of the contingency.
Nevertheless, critical facets of subjects’ behaviour get faster, and the way they do so is very interesting. That is, successive random symbols could, by change, follow the same rules as successive deterministic symbols. That is, if you see 1,2,3,4; then it could be that 1*3 is deterministic and 2*4 is random; or 2*4 could be deterministic with 1*3 being random. If 2*4 is random, then it would be unpredictable, and so subjects should be slow. But they aren’t – since 2*4 would have been deterministic if the ‘phase’ of the cycle had been different, subjects are apparently quick. The way that this untoward speed builds up is then a signature of implicit (and in this case, incorrect, or at least unnecessary) learning, and something we can use to build models. My student Noémi Éltető, who constructed a model of this, and I are now extending it to capture structure in other sequences, such as the songs of Bengalese finches.
Looking at the big picture of these three tasks that you described, what were some of your key findings so far?
The most critical finding is that there's rich structure in the overall course of learning, with both regularities and individual differences. We hope that some of it will generalise across tasks. So we now have a very compelling project in the future of then trying to understand those two things, which are pulling in two different directions. Clearly, we need have generalisation across the 115 mice in our IBL dataset, rather than have 115 separate theories. That would not be a scientific result.
In the three tasks we found three different things. In the IBL task, my student Sebastian Bruijns, found that there were different types of states of task knowledge/performance expressed by the animals over the course of learning. One of these state types, we didn't expect at all. This middle type had an interesting structure where it looks as if the animals are paying attention to only one of two sides of a screen on which a stimulus can occur, and guess randomly when the actual stimulus comes from the other side. We didn't expect that. Then we discovered other things about the number of states and when the states appear in behaviour, and how fast and slow changes in behaviour might work.
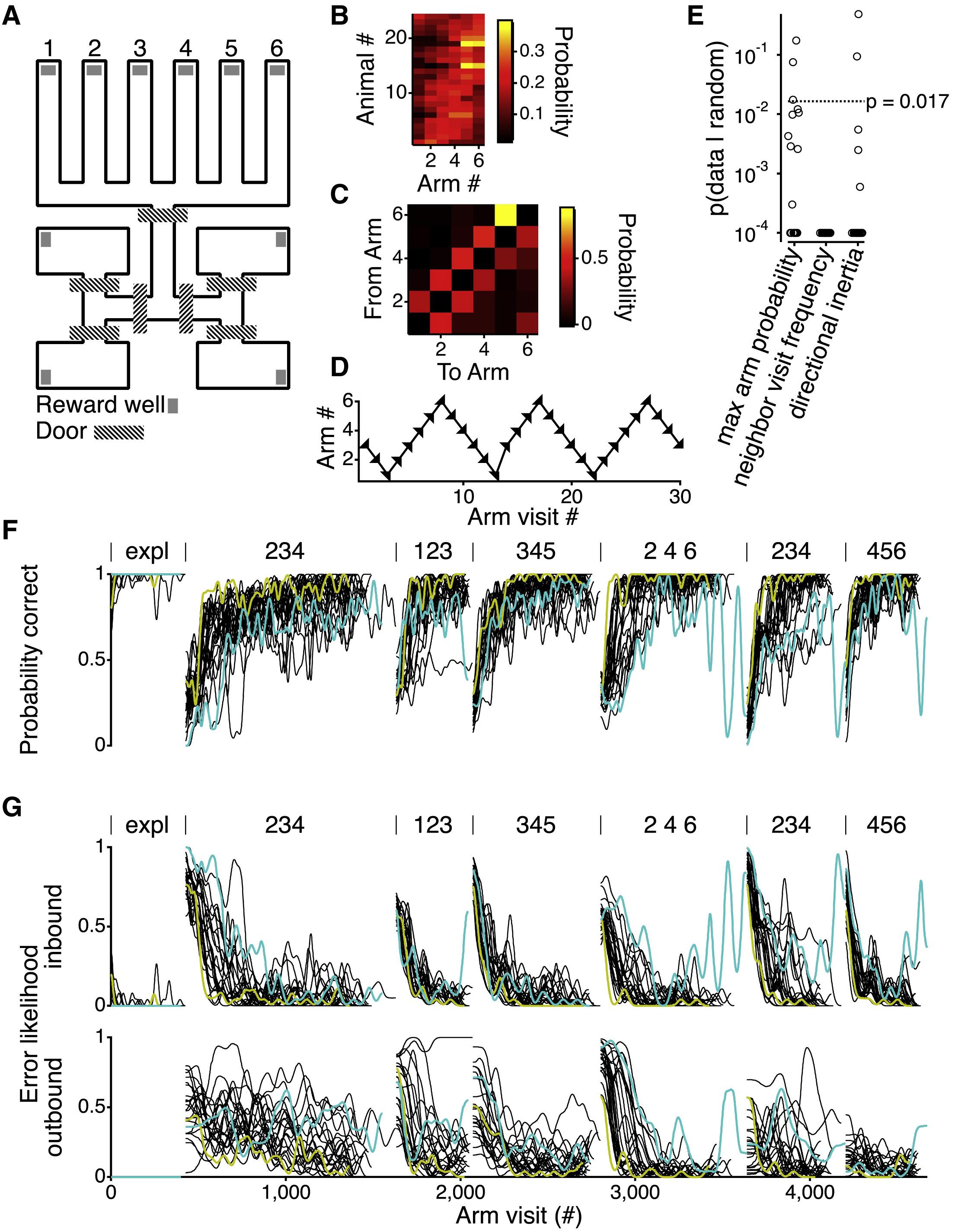
Adapted from Kastner et al. 2022
In the Kastner task, the lessons are a bit harder for us in the sense that our model is only barely fitting the data well enough even to offer insights. In a way, the biggest thing we discovered there is that our modelling remains somewhat incompetent. So there are other things that we need to do, but it nevertheless can be revealing to look at. The things that it took us to fit the model that didn't work so well actually told us quite a bit about the tasks, like some of the long run contingencies and so forth that we know from exploration and other behaviours.
There is what I call the ‘Anna Karenina problem’: “All happy families are alike; each unhappy family is unhappy in its own way.” Optimal behaviour can be a bit unrevealing, because it’s optimal, and can be realized in many different ways. It’s the nature of suboptimality that tells us about the actual behavioural and neural algorithms and informs the models we need to build.
The ASRT task taught us about the sloth of people’s learning. It's an implicit learning task, so they didn't actually have to learn anything at all. If the model is true, we've quantified aspects of forgetting (one reason why learning is so slow) and aspects of how they make other sorts of inferences about the domain that they're working in.
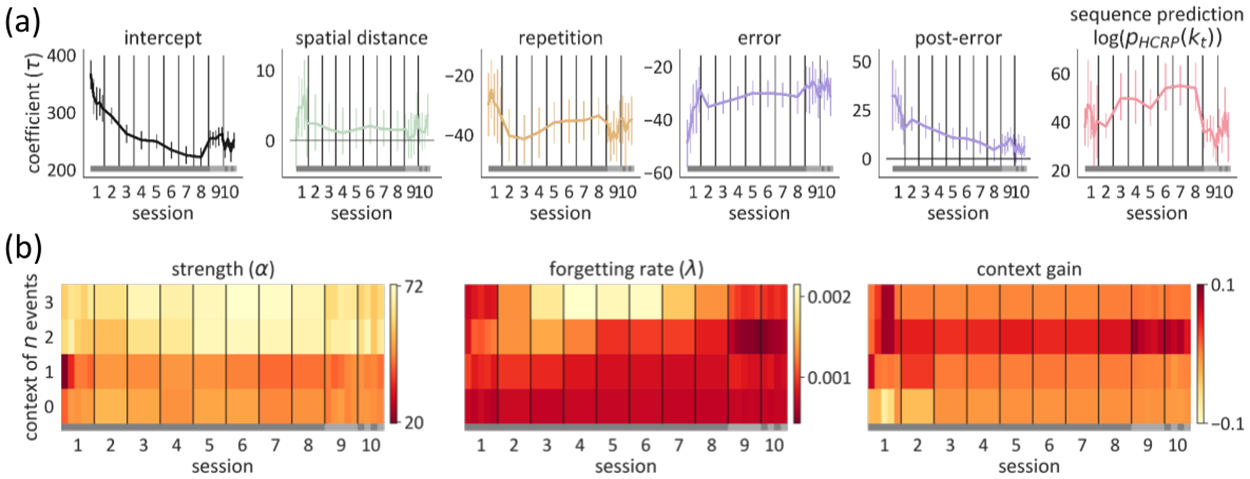
Adapted from Éltető et al. 2022
You said in your talk that some of your models can be more descriptive than mechanistic. What is your take on descriptive compared to mechanistic models?
In the end, the best models are essentially mechanistic models. In science we're trying to build mechanistic models at multiple scales. But you build a model at one level out of pieces at lower levels. Sometimes those pieces are mechanistic models themselves; but often, we don’t know enough to specify how those lower level models work, but only what they do. These are then descriptive place-holders for future scientific discoveries. So it’s inevitable that some aspects of mechanistic models lie in the eye of the beholder.
In this instance, the mechanistic models should be of the course of the reinforcement learning that takes the animal from ignorance to mastery. And the trouble with the model of the IBL task is that at the moment it doesn't reflect anything about how the animal might come to acquire these states. We see a state, but how did the animal develop it and what did it learn about the task such that we can then see how it instantiates that state in some neural circuitry? Why did it choose to use that state there? What was the switching process, stochastic or targeted in some way based on experience?
That's why that sort of descriptive model is, at some level, unsatisfying. But maybe it provides us with enough constraints to go look at the neural data. If we really believe in this state as being a realized entity, maybe we can find some evidence of it in the neural data. We can then understand what the state means in terms of what the brain does when it prevails, how that state came to be, and even what the neurons were doing before. So we hope that the state description, even as a mere description, will help us essentially unpick aspects of the neural data and thereby tell us about algorithm which led to those states themselves.
Then in the Kastner task, we have a mechanistic model, but we don't believe the mechanistic model completely. It makes some systematic errors. So there's another case where having a mechanistic model that isn't right can still be extremely useful. But is it more right than a descriptive model, which does a better job of describing behaviour? It’s not clear. Models all have a purpose. There’s an (in)famous saying (from Box) that goes, ‘All models are wrong. Some models are useful.’ Descriptive and mechanistic models fit into these classes.
What are some of the main implications of your findings?
The main implication is that there needs to be more work! That’s typically the case in science. For the IBL project, the main implications are that we now have correlates and neural data we can use to build mechanistic models. They hopefully will help us understand these states, which I think a lot of people are interested in. If the animal really is switching states at various times, then we need to understand what the neural behaviour is like.
For the Kastner task, the implications of the work are about this very extreme past dependency. There are also some technical implications to do with what is known in the machine learning literature as behavioural cloning. Our mechanistic models attempt to recapitulate what the animals themselves do – generating choices, receiving rewards or non-rewards, updating internal parameters associated with the policy, and then generating new choices. Normally, when we fit the models, we feed in the choices that the animals made and the rewards they received up to some time, and then attempt to predict as well as possible their next choice. But consider what happens if we run our model on the task by itself. Does fitting the next choices well guarantee that the model will perform the task to a similar degree of (im)perfection as the animals? Actually not – since small errors in the way we fit the choices can feed on themselves, so that the model generates successive choices that become quite unlike (in this instance, much worse than) the animals’ choices, and so can completely fail to solve the task. There are ways around this – for instance, we can run the model based only on its own choices, and then attempt to match summary statistics of the animals’ performance (such as reward rates, or the prevalence of particular sorts of error).
This problem turned out to be quite revealing about a critical feature of the task. In it, we change the rules a number of times, going from one contingency to the next. The animals learn new contingencies quickly. That means, though, that our fitting process experiences very few choices that tell us how the animals accomplish this feat. Worse, we know it is also influenced by other inductive biases that the animals have. So when we fit our models in the normal way, accounting for every single choice the animal makes, they give up on characterizing those critical trials well, perhaps because there are not enough of them, and because there may be some randomness in the exploration that the model can never exactly predict. The trials are also anomalous – all sorts of things happen during those exploration trials.
This comes to show that even if you have a model class that is good, the way you fit the model actually gives you parameters which don't work very well. So you have to fit it the second way, which is to see if the behaviour the model produces looks in some way like the behaviour that it is trying to get in the first place. You can generate different parameters and the model still fits the data reasonably well. But we know that they're missing aspects of the data themselves. And so this is a case where model mismatch has a big effect on our ability to characterise the data properly. There are some important technical lessons from that modelling.
Could you explain the difference between parametric and non-parametric models that you use?
Non-parametric is a term of art that comes from the statistics community, which is essentially a terrible word because unfortunately our non-parametric models are full of parameters. What they mean by non-parametric is that we don't want to start off with a particular number of parameters to characterise the behaviour of a subject, for example, but rather have an extensible model that can accommodate the amount of complexity that there is in the data.
One of the inspirations for our work is the GLM-HMM model, which I discovered a lot of people at SWC/Gatsby Unit are using. There, you typically have a certain number – say three – states, each defined by certain number - say seven – parameters, so let’s say you have 21 parameters. Then, you decide the best value of those 21 parameters inside the context of this model. That’s very parametric.
In our case we don't know whether there are three, four, five, six, or even seven states. Some people fit a model with these different numbers of states and see which fits the best, taking care to correct for the fact that models with more parameters can over-fit the data – characterizing noise rather than systematic structure. But a more holistic approach is to start from a different perspective. The world is very complicated. The learning curve is very complicated. Maybe there are really hundreds of states. However, we do know that we cannot have evidence about more states than we have trials, for instance – since states only reveal themselves on trials. So instead, we define a statistical structure that allows for the possibility that there are just lots of different states, without us having to pin down what that unbounded number. So even this so-called non-parametric model has parameters. What makes it non-parametric is the fact that we're not being individually specific about the amount of structure there is to start with.
That's important for our applications because we simply have no idea how many states an individual goes through. One individual might be very different from another. Indeed, that is exactly what we found – that different animals have different numbers of states.
You mentioned that some of your models are don’t yet capture the data perfectly. What are some of the next pieces of the puzzle that you are excited to work on?
For the ASRT task, I'm excited to use the same model class for other problems. One is for the song of Bengalese finches, in which there are what is known as branching points. At one of these branching points, how many of the past syllables influence the choice of a branch? Is it just stochastic where it makes some immediate choice, or does it look a couple steps back? What is the structure of the song?
For the IBL task, we have a lot of different directions. The most important thing now is to first fit the model on all the data we have we collected so far, and then integrate it with the experimental work on learning.
On the Kastner task, one direction is to look at data that David has on rats with mutations in genes associated with autism in humans. We want to understand what's different about their behaviour. David also has a much more ambitious project in which he seeks to develop a behavioural rhyme of the genome-wide association study (GWAS). In this method (with the slightly fishy name of choice-wide behavioural association study, or CBAS), he is looking at the combinatorial number of behavioural sequences involving a modest number of choices, and asking whether their statistics differ between two or more groups – in the same way that in a GWAS, one looks for the potentially few genes out of a large number that differ between people with and without a specific disease, for instance. We are excited to see whether we can use this bottom-up or data-driven method to learn more about what differs between our groups of animals.
Having helped found the Gatsby Computational Neuroscience Unit, how do you envision the collaboration between Gatsby and SWC?
Of course, Geoffrey Hinton founded the Gatsby Unit and I helped as co-founder together with Li Zhaoping. SWC having opened much later than Gatsby, the issue of how they should interact is something to be designed afresh based on what they are currently like. I'm obviously delighted to see the interactions that are in the building. When I left Gatsby, they were still just starting to build SWC and the research groups. Now, it’s great to see more new faculty at SWC who are themselves very much interested in the interface between theoretical and experimental approaches. Tim Behrens on the SWC side would fit as perfectly into Gatsby as the SWC, for example, and Andrew Saxe also lives between the two.
I think the opportunities for close interactions are very much there because of the set of questions that everybody is interested in. What I haven't had a chance to find out about in this short visit is how the educational programme has changed since my time – whether or not the eagerness of the experimenters for more theoretical additions to their programme is satisfied, whether it's theorists who are doing rotations in experimental labs in a way which actually embeds the collaboration. But I'm sure this is what will be happening.
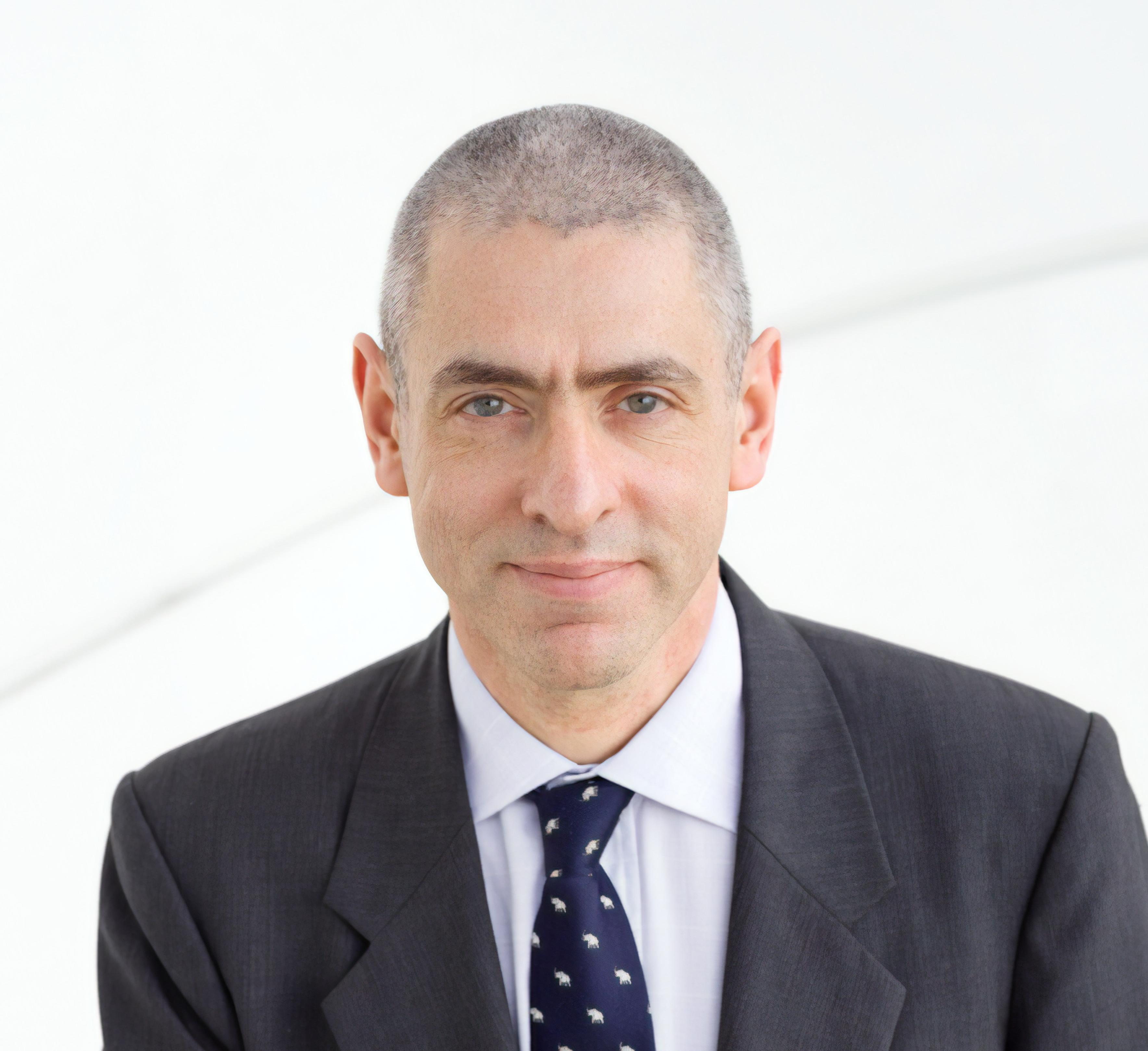
About Peter Dayan
Peter Dayan did his PhD in Edinburgh, and postdocs at the Salk Institute and Toronto. He was an assistant professor at MIT, then he helped found the Gatsby Computational Neuroscience Unit in 1998. He was Director of the Unit from 2002-2017, and moved to Tübingen in 2018 to become a Director of the Max Planck Institute for Biological Cybernetics. His interests centre on mathematical and computational models of neural processing, with a particular emphasis on representation, learning and decision making.