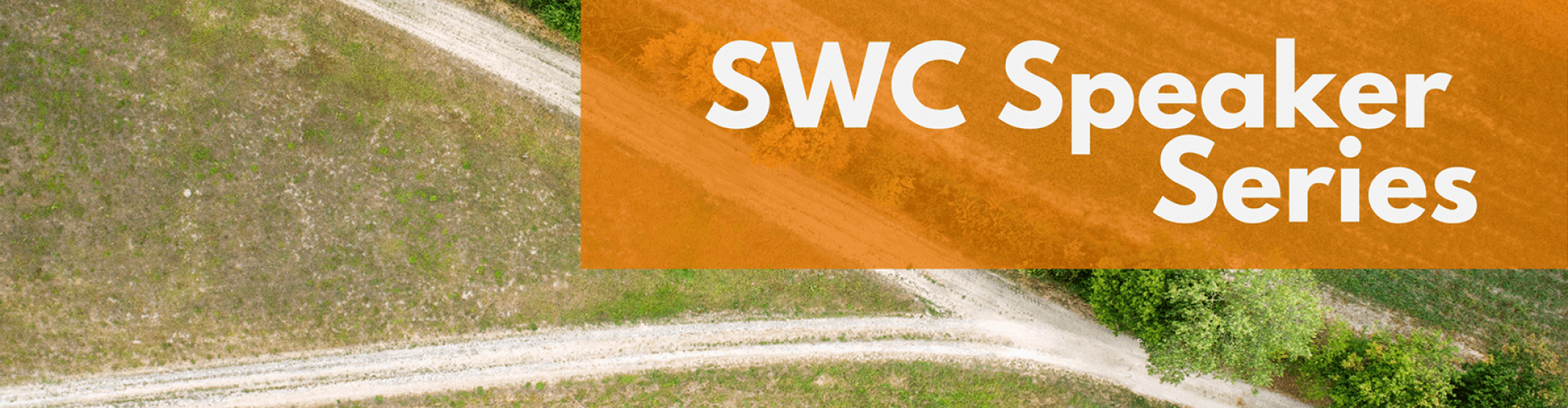
Making sense of flexible decision-making in navigation
An interview with Dr. Shin Kira, Harvard Medical School, conducted by Hyewon Kim
When we are walking to a given location, but then are told to navigate to a new location, we can flexibly change our course and redirect our steps. How does the brain allow us to do this? In the second of this year’s Emerging Neuroscientists Seminar Series, Dr. Shin Kira shared his work on flexible decision-making during navigation. In this Q&A, Dr. Kira elaborates on the population code that the brain uses to produce this flexible behaviour.
What are some examples of flexible decision-making in navigation in real life? Why did you choose to focus on this question for your research?
In our daily life, we often navigate to our destinations. For example, if I were invited to the Sainsbury Wellcome Centre, I would need to remember the destination building in my mind while walking. But this process is remarkably flexible. If I am instructed to go to a different building by email, for example, I can immediately change my destination and redirect my navigation route. This process is not trained by trial and error, but happens very rapidly, flexibly, and proactively.
Our brain can deal with many combinations of memory and sensory stimulus, and very flexibly combine them to link them to an appropriate action. This is an amazing ability of the brain which I wanted to study.
What had been previously known about decision-making in navigation?
Navigation studies tended to focus on the encoding of spatial variables, such as the animal’s location, heading direction, and running velocity. But these studies typically did not investigate the mechanisms of decision-making in which animals must choose a navigational path among alternatives.
In contrast, many studies of decision-making were carried out using perceptual decision tasks that did not involve navigation. In these tasks, a person or animal would receive some sensory stimulus, and need to make a decision on what they perceived. For example, they observe a noisy motion stimulus and if it is moving to the right, they press the right button and if to the left, they press the left button. In a sense, they would report what they perceive. These studies revealed that neural activity can represent ongoing decision-making processes in several brain regions, including parietal and frontal cortices.
Recent approaches have been developed to study navigation and decision-making together. These approaches have shown that sequences of neural activity in the posterior parietal cortex (PPC) correlate with perceptual decisions during navigation. Similar activity has been observed in the retrosplenial cortex (RSC).
The association between perception and choice is usually fixed in those perceptual decision tasks with or without navigation. Whereas if a person is instructed to press the opposite button, for example, like pressing left if they see a right motion and vice versa, the person can do that very flexibly. But that kind of flexible association had not been studied so extensively yet. That was the part I wanted to get at and is the focus of this study.
What kinds of methods did you use to address the question of flexible decision-making in navigation?
We trained mice to perform a virtual-reality T-maze task. As the mouse ran through the maze, the mouse sequentially observed two cues separated by a short delay. To receive a reward, the mouse performed flexible decision-making to choose a turn direction by combining the short-term memory of a remembered first cue (sample cue) and the visual information of the second cue (test cue).
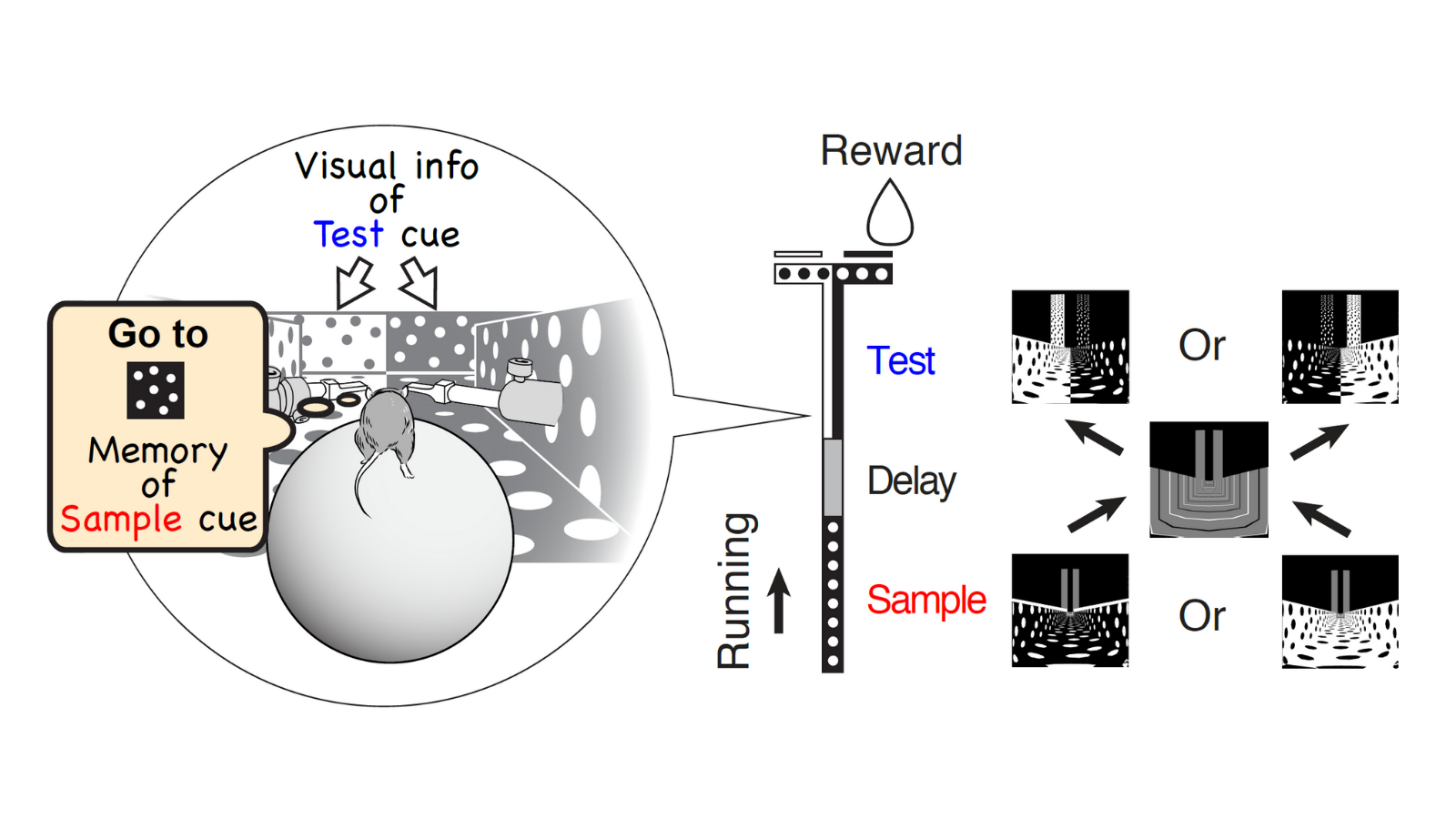
This flexible navigation decision likely involves a lot of brain areas because navigation and decision-making signals can be represented in many parts of the cortex, including the PPC, RSC, frontal cortex, entorhinal cortex, and hippocampus, etc. There are so many candidate areas that are potentially involved in the task.
The typical strategy in the field is to pick the best guess and just focus on that one area and look at the activity there. If you find a behaviourally relevant activity, that is a good result. But we wanted to be a little more systematic in our approach because of the wide range of candidate areas.
We first did a systematic screen by optogenetic inactivation. We inhibited each part of the cortex and found that the posterior side of the brain is more involved in the task, particularly the visual cortex, PPC, and RSC.
Then, we further narrowed the focus into that area and looked at the neural activity more closely. By comparing the visual cortex, PPC, and RSC, we found that the RSC is most informative for solving the task by signalling, or shows the most conjunctive encoding of visual stimulus and memory.
We could easily focus our attention on the PPC, for example, which has been implicated in decision-making in previous studies. But actually, the PPC contained less information about the reward direction than the RSC. So in a sense, just focusing on a specific area with one’s own belief could be potentially misleading. You still see some signals, but it may not be a majorly involved area. By using a systematic approach and comparing regions, you can be more confident that a certain area is likely essential for a particular function.
What does it mean for neurons to nonlinearly mix contextual information from short-term memory and visual information?
Nonlinear mixing has been studied extensively by many neuroscientists, including Dr. Stefano Fusi and his colleagues. One argument is that nonlinear mixing will increase the ways you can classify those stimulus combinations. To use a more technical term, that will increase the dimensionality of the stimulus representation. If there is no such nonlinear mixing, then the brain cannot generate reward direction signals in our task, because that requires a specific computation called “exclusive OR (XOR)”.
What is a population code in the context of the brain and why is it important for navigation?
In our study, we found a very specific type of nonlinear mixing: conjunction, also known as the AND computation between sensory and memory signals. That results in the selectivity to a specific trial type (or maze) in our task.
In relation to the previous navigation literature, the navigation system is set up so that it allows the mouse brain to signal the specific location in a maze. Toward the end of my talk, I discussed these combinations of sample and test cues being interpreted as different mazes. This conjunction could be a signal pointing to a specific location, and that could be how the mouse solves the task in this case, figuring out what type of maze it is in. Then, the mouse can learn which direction to turn for each maze.
Were you surprised to find this visual-parietal-retrosplenial network to be involved in flexible decision-making and navigation?
The PPC has been shown to be important for navigation decisions in previous studies, including those of my advisor, Dr. Chris Harvey. My first speculation was, maybe PPC is primarily involved in the task. There are many people out there who are interested in the RSC in the context of navigation, but for the decision-making task, I did not initially pay much attention to the RSC. Then, the optogenetics studies suggested that the RSC may be also involved, prompting us to look at that area more carefully. Then we found a good mixture of memory and visual information in the RSC. That part was quite surprising.
But it makes sense retrospectively because that involvement seems to build on top of the navigation system that has been studied by many neuroscientists, including Dr. Troy Margrie and his colleagues. Previous studies have shown a lot of conjunctive coding in RSC during navigation, so our results can be interpreted as one type of conjunctive coding that combines visual and memory signals to create mixed selectivity.
How do you think these findings can be translated to other animals or humans?
We don’t have much knowledge about how the human brain represents the combination of stimulus conditions. But we know that in the hippocampus, for example, cells can respond to a specific person with distinguishable features, such as Marilyn Monroe. We can think of that as one type of mixed selectivity – the concept of a person consists of many features: how she looks, sings, dresses, and so on.
Similarly, in the temporal cortex of monkeys and humans, we can find face-selective cells that respond to the combination of face parts. For example, when a single eye is presented, these cells don’t respond. But when the eyes, nose, and mouth are presented together, the cells start to show a robust response. That is one type of mixed selectivity in the temporal lobe of the brain. And interestingly, lesion to that area leads to face blindness called prosopagnosia, where one can still recognise each part of the face, but cannot recognise the face as a whole or discriminate between faces across people. So mixed selectivity can be important for that – recognition of familiar but complex objects.
This might also extend to other modalities such as audition. When you hear somebody speaking with an accent, you can still accommodate the accent while comprehending. You don’t really listen to the word phoneme by phoneme, but you can perceive it as a word or sentence. Or when we listen to jazz music, the music can be heavily arranged, or improvised, but we can still enjoy it and recognise the music. So I think mixed selectivity might be necessary for such holistic recognition across modalities.
What is the next piece of the puzzle your research is going to focus on?
I call the behaviour we employed in our work ‘flexible navigation,’ but the flexibility is very limited in the sense that there are only two types of sample cues and test cues. The brain can create cells with mixed selectivity for each combination because there are only four combinations that are important for solving the task. But one can easily increase the number of sample and test cues, which will significantly increase the number of combinations.
And even more drastically, we have to deal with a far greater number of stimuli and their combinations in the real world. The number of features is not limited to two, but unlimited. So it basically creates an infinite number of stimuli and their combinations, but somehow the brain has to link each combination to an appropriate action. This seems like a very difficult problem, but the brain manages it!
So my ultimate interest for the future is how the brain deals with numerous combinations of how the world can appear by linking these combinations to appropriate behaviours. For the brain to achieve this, it is essential to make an educated guess in a novel situation based on their similarity to your past experiences or rules. I like to call this generalisation.
I want to focus on two cognitive abilities that are likely important for generalisation. The first ability is to categorize related stimuli based on their similarity, which would allow you to deal with a novel situation by assigning each of its features to an existing category and making a decision based on the categorical combination. The second ability is to simulate how a situation would evolve over time, which would also allow you to make inferences for a novel situation in the future before experiencing it. In addition, I want to find methods to improve these abilities when they are impaired by brain lesions or cognitive disorders.
These are the directions I want to go in the future: understanding and improving how the brain enables generalisation and make decisions efficiently in novel situations.
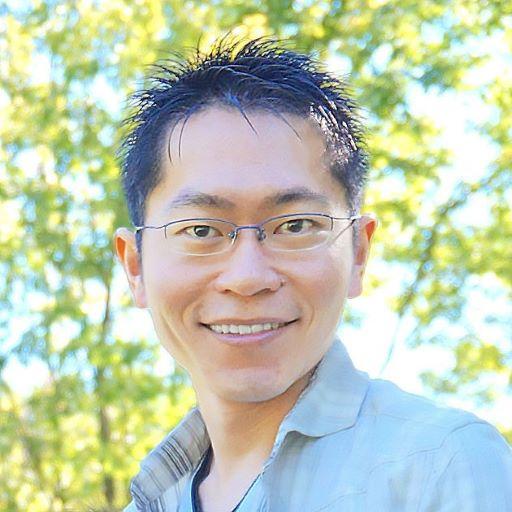
About Dr. Shin Kira
Shin Kira is a postdoctoral fellow in the Harvey Lab at Harvard Medical School. He received his MD from Tokyo Medical and Dental University in Japan, and completed his Ph.D. in the Shadlen lab at the University of Washington and Columbia University. For his thesis projects, he studied decision-making in monkeys and humans by using electrophysiology, psychophysics, and computational modelling. Now as a postdoctoral fellow in the Harvey lab, he studies flexible decision-making in mice by using calcium imaging and optogenetics. His primary interest is in how the brain forms generalisations of past experiences to make rational and flexible decisions in novel situations. He envisions to investigate neural circuit mechanisms underlying generalisable intelligence and its recovery from impairment by advanced neural recording and manipulation techniques in mice.