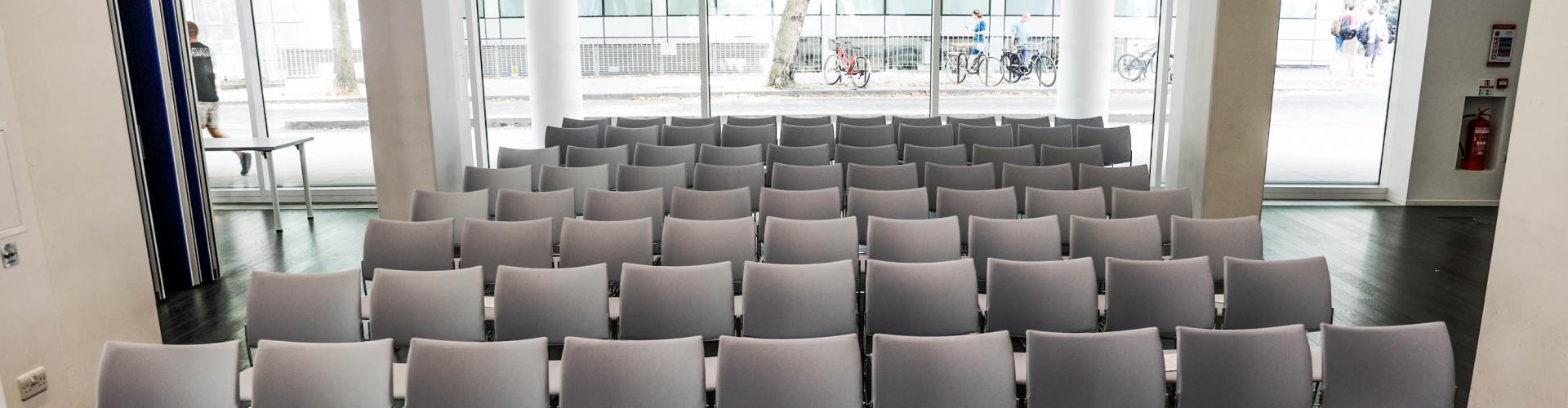
Understanding sensorimotor circuits
An interview with James Fitzgerald, Ph.D., Group Leader at Janelia, conducted by April Cashin-Garbutt, MA (Cantab)
Finding order in the immense complexity of neuronal networks in the brain is one of the central challenges of neuroscience. In the following interview, James Fitzgerald outlines how his lab is tackling this problem to understand sensorimotor circuits implementing zebrafish behaviour.
What general challenges do neuroscientists face in trying to understand the neural circuits underpinning behaviour?
One of the critical challenges about developing a neuroscience of behaviour is that many behaviours engage numerous parts of the brain. In most animal models, we don’t have the opportunity to gain access to everywhere in the brain, therefore we are not able to see all the signals that might be relevant for understanding the behaviour.
Another thing that is challenging about understanding behaviour is that the brain is a multi-scale system. For example, behaviour is something involving the entire body of the animal, however, effects at the level of single cells, or even smaller, can have a consequence on that output.
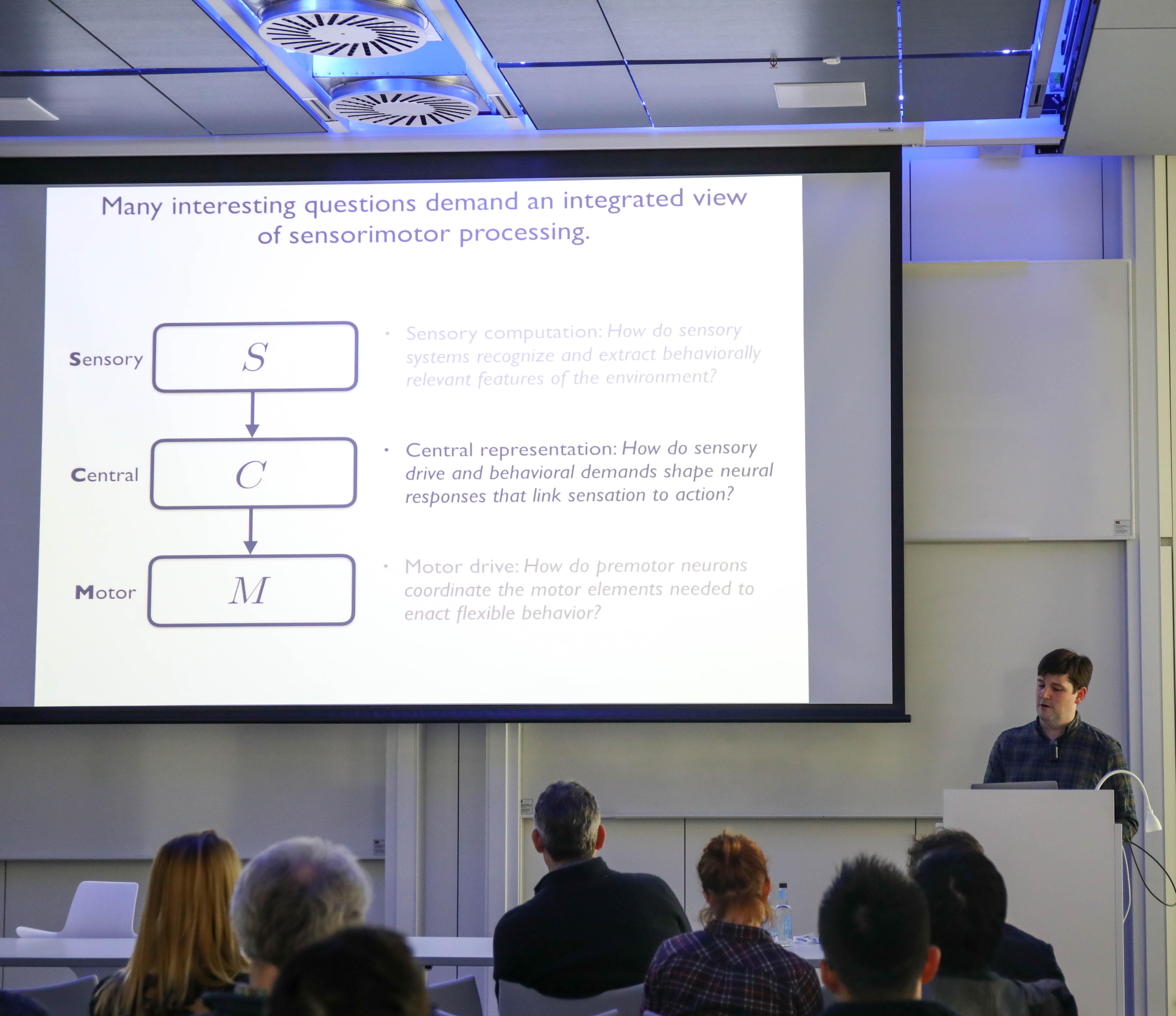
Why do you focus on larval zebrafish in some of your research?
My general approach is to try to find the simplest nervous system I can in order to ask the questions that I’m trying to understand.
One of the things that really attracted me to the larval zebrafish as a system for trying to understand the circuits underlying behaviour is that when it is in the larval stage it is optically translucent, and as a consequence you can do imaging throughout the entire brain.
This property means that you are not, in any way, limited to only looking at certain structures. You can start by doing an unbiased search throughout the entire brain in order to find signals that seem to associate with the stimuli you might be using to drive the behaviour or the motor output of the animal itself.
The other thing that makes the zebrafish an ideal system is that you can get cellular resolution in conjunction with whole brain imaging. In fact, all of the canonical neuroscience techniques that are used to understand brain circuitry in other animals are also accessible in zebrafish.
So, it’s not that whole brain imaging is the only thing that makes zebrafish appealing, but I think that it’s the missing link between small-scale descriptions of the brain, involving cells or molecules or synapses, and large-scale descriptions of the brain, such as behaviour or cognition.
With such a vast neuronal network in the brain, how do you begin to unravel the brain-wide circuitry implementing behaviour?
As a computational neuroscientist, I try to have two different ways of entry into the system. One way is bottom-up, which is a very data-driven way involving looking at what the brain is doing under different types of functional circumstances, and from that data, starting to make some new hypotheses about how the whole system might work.
Because the brain is an extremely complicated place, there’s obviously going to be many different interpretations of the data that are going to be consistent with whatever it is that you’re trying to understand. So, I take an Occam’s razor approach where I try to build the simplest model that I can, in order to develop a model of the behaviour without violating any of the known experimentally derived constraints.
However, because the way the brain is responding is heavily influenced by the sensory conditions and motor conditions you put it in, even if you get a very good model about how the brain works in that circumstance, it is not clear that your model will generalise very well to naturalistic behaviours that might involve a variety of different types of sensory inputs, interacting modalities, and lots of flexibility in behaviour.
The other approach I take is a top-down, principles-based view, which is that once you get some ideas about how things might be working, we try to come up with some candidate theories and hypotheses and principles that we can then use to try to make new experimental predictions. The hope is that this will push the experiments into a regime that will start probing phenomenology that’s interesting from a viewpoint of what we already know, but also tries to push us into new frontiers.
Overall, I try to keep models as simple as possible. One of the challenges in understanding the brain is its immense complexity, and if you replace an immensely complex biological system with an immensely complex model, it is not clear how much you gain. But if you replace a very complicated brain by a very simple model, you can often understand that model completely and that can give rise new to ways of thinking about experiments and existing data.
How do you make sure your models are as simple as possible?
I would say that it depends on the question that you are trying to ask.
One way that you can find a simple model is to hypothesise that systems evolve to optimally achieve goals. Once you’ve made that hypothesis, then you can analyse the system from a purely theoretical point of view, and if that gives good predictions for the biology, then you have a very simple description where you know how to think about its various pieces.
For example, in my lab we are trying to understand the sensorimotor circuit that generates swimming and turning behaviour from moving visual stimuli, which is called the optomotor response.
It is hypothesised that the optomotor response is an innate behaviour in the fish, because by turning in response to motion and swimming with respect to the motion, the fish can stabilise itself in a moving environment.
If you think of a fish in a stream, it has no way of knowing that it is moving based on a direct velocity sensor. What the fish needs to do is use its other senses to infer its velocity. Many animals, including fish, as well as flies and humans, detect motion by looking at patterns of light as they change over time. By processing how the visual scene is changing in a spatiotemporally correlated way, the animal can get some sense that it is moving.
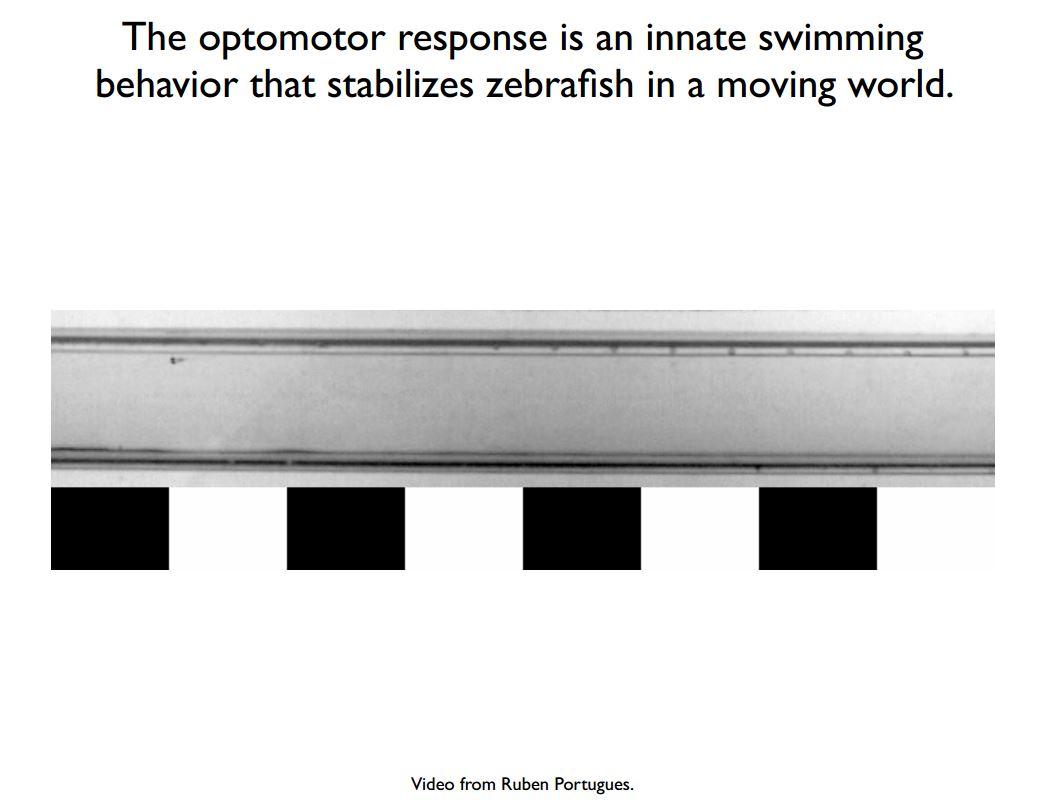
One way we might start to think about the earliest processing, which would be in the retina, is to think about the entire goal of the system. The goal of the system is to accurately estimate the velocity of motion. But, it’s not the velocity of any motion, it’s the velocity of motions of naturalistic environments.
And so, we start to focus on the central statistical problem and ask: if that environment was moving with some velocity, what are the patterns of change that would hit the eye, and what strategies might the brain use in order to infer such naturalistic movements
An entirely different way that one can find simplicity is by using a very bottom-up, data-driven approach to create a model. Once you have a model, you can try to apply model reduction, which is to say that even though a model has many parameters in it, there might only be a limited number of those parameters that significantly affect the output.
For example, we used this approach on the zebrafish brain, and we identified different parts of the brain that seemed to be involved. From those response patterns, we put together a whole-brain model about how sensory information might be processed by the retina and eventually affect the motor outcome of the animal. Again, once you have that model, even if it has come from a data-driven method, you can ask, how sensitive is the output of the model to different types of parameters. This can sometimes enable you to realise that even though biologically there is a lot of complexity in the model, functionally, there is only a small amount of it working together that is required in order to generate the output.
As we’re going towards very large-scale pictures of behaviour in neuroscience, I think that there are going to be many biological details that don’t matter for specific functions, but I think that the brain is not just doing that as a waste of energy. What this means is that we need to think about other functions that the brain might be performing so we start to understand why that complexity might be there.
If you are trying to explain something that turns out to be simple, you shouldn’t force that complexity into the model. You should look for more complicated behaviour or more complicated cognitive function that would enable you to understand the origins of that complexity.
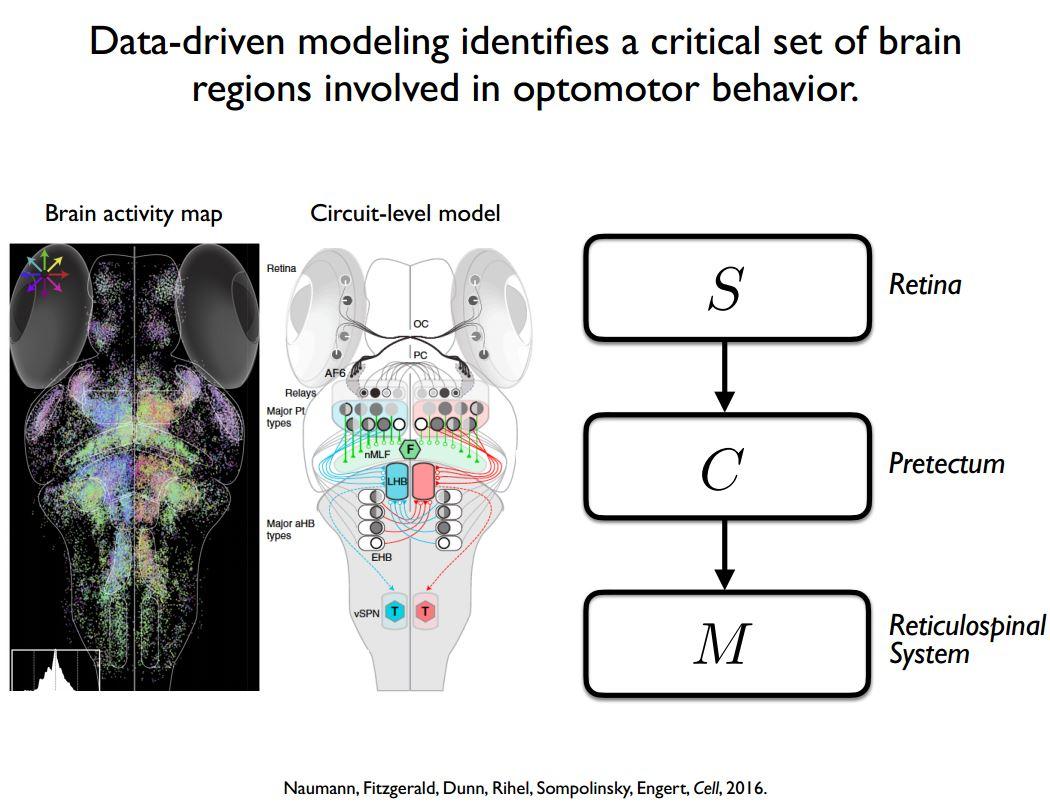
Does your research on visual processing have any implications for humans?
There seems to be a lot of commonality across the animal kingdom in visual processing mechanisms that we have studied in fish and flies. For example, this research predicts an optical illusion that also applies to humans. I can show you a movie where each pixel is black or white, and it is changing over time, and if I take every pixel that was white and I make it black, and I take every pixel that was black and I make it white, you’ll see it move in the opposite direction.
We can relate this optical illusion to light/dark asymmetries in natural environments. Natural environments do not have equal distributions of light and dark. Because light and dark are not the same in terms of our environment, they’re also not perceptually the same. These movies are designed to see whether or not these so-called illusions, which actually help processing in natural environments, are perceptually relevant, and whether we can we predict illusions from the statistics of the movies in their natural environment.
Can you please outline your theory for how sensory drive, behavioural demands and neurobiological constraints together shape neuronal responses in the pretectum?
My lab is looking in a central part of the brain called the pretectum. The pretectum gets direct retinal inputs, and we think drives a couple specific types of motion-guided behaviours. This doesn’t occur directly as there is another relay station in the brain before it goes down the spinal cord, but we think that the logic of the pretectum is to respond to visual stimuli in a way that allows it to drive these visually-guided behaviours.
What I find to be quite interesting about the pretectum is that, even though it is a visual area that’s entirely being driven by the retina, the heterogeneity of neuronal responses we saw within pretectum was much easier to understand in terms of the behaviours being generated.
One of the models that I presented during my seminar at the Sainsbury Wellcome Centre was a very simple model that says: what if the goal of the pretectum was to generate these behaviours, subject to a couple different types of constraints? Because this is a simple model, we can then analyse how both the behaviour being generated and the constraints that we put on the system together generate the pattern of the responses we see in the pretectum.
We find that there are very large response components within this pretectum model that have no functional output at all, but they’re needed to satisfy the constraints. This is interesting because one thing we had realised, even back when we published the whole-brain model from the data-driven approach, is that there seemed be some responses in the pretectum that, as far as we could tell, didn’t have any functional impact. It wasn’t clear at that time why that would be so. What this model shows is that some of that is actually required in order to satisfy the constraints that we put onto the network.
That picture doesn’t fully account for the pretectal response patterns, but now we can see what component of the activity is required to generate the behaviour, what component is required to satisfy the constraints, and what component is left as a residual. This gives us part of the response that we can use to form new hypotheses about what other parts of behaviour or cognitive function might be relevant, or what other constraints might be on the system.
Are your observations likely to apply to other sensory areas in the brain?
I hope so. When you are way out in the sensory periphery, you have a lot of information coming in that you need to transmit to the brain, so there’s a big pressure to keep as much information as you can. Once you are in the central brain, that pressure switches, as what you want to do is not necessarily keep information. You actually want to discard information that is irrelevant for the types of cognitive or behavioural processes in play.
What I’m hoping is that after we understand the pretectum in terms of a few specific motion-guided behaviours, we will gain general insights into how brains should represent sensory information in order to drive different types of behaviours. This is part of the motivation for the collaboration I have with Isaac Bianco’s lab here at UCL. What we’re doing there is trying to understand, as fully as we can, the way that the fish’s behavioural output is generated by descending commands from the central brain. I’m hoping this will help us to better understand representation of sensory information in other parts of the central brain.
What are the next pieces of the puzzle your research is going to focus on?
Right now, the two pieces of the puzzle are to get a more complete understanding about zebrafish behaviour as it relates to the premotor coding, and that’s in collaboration with Isaac Bianco’s lab in UCL. And then the other part of it is to try to see to what degree this theoretical perspective I’m bringing to pretectal coding can be applied to other motion-guided behaviours that depend upon the pretectum.
About James Fitzgerald
James Fitzgerald is a Group Leader at the Janelia Research Campus of the Howard Hughes Medical Institute. He is broadly interested in combining first-principles theory and data-driven modelling to develop predictive frameworks for neuroscience. He previously studied physics and mathematics at the University of Chicago, received a Ph.D. in physics from Stanford University, and was a Swartz Fellow in Theoretical Neuroscience at Harvard University.