
Space and time in the entorhinal cortex
2014 Nobel Laureate Professor Edvard Moser recently gave a lecture at Sainsbury Wellcome Centre where he focused on three main topics: the integration of grid maps in merged environments; a new type of cell called object-vector cells and time-coding in the lateral entorhinal cortex.
History of hippocampal spatial recording
Firstly, Moser began by giving a brief history of hippocampal spatial recording starting with Professor John O’Keefe’s work in 1971 recording from the hippocampus in freely-moving rats exploring open spaces and mazes of different kinds, where he found a large population of cells in the hippocampus that respond to the animal’s location called ‘place’ cells. Based on these observations in the 1970’s, Professor O’Keefe suggested that ‘place’ cells were the basis of a cognitive map of space and other aspects of the animal’s experience.
Many years later in 2005, Professors Edvard and May-Britt Moser found another type of cell in the medial entorhinal cortex called ‘grid’ cells. These cells fire at many different locations that form a very regular pattern across the space available to the animal, hence the name ‘grid’ cells. Grid cells, Moser explained, have several dimensions of variation, the three major ones are:
- Phase – in the xy position of the firing fields
- Scale – one may have wider spacing and field size than another
- Orientation – rotational position relative to neighbouring representations
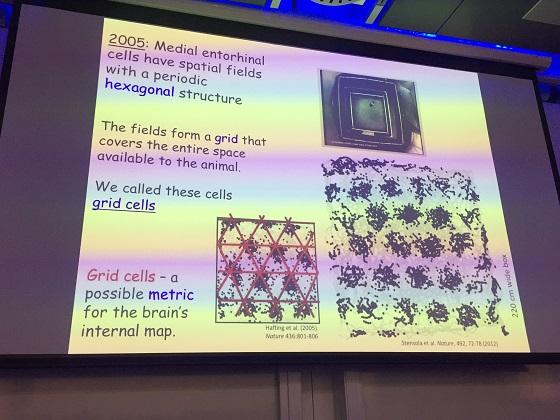
All of these variations are found among grid cells in the entorhinal cortex, but there are some patterns in how they are distributed in this brain area. Scale of the grid appears to be organized topographically, varying systematically from dorsal (top) to ventral (bottom) of the medial entorhinal cortex. Typically at the top you have only grid cells with high frequency or close grid fields with small scale and at the bottom you have more grid cells with the large scale.
This change Moser first thought was gradual, but the data from studies in London and Trondheim showed that there are discrete jumps, where you have small scales mostly at the top and then you may have nothing in between, then you get new levels. These levels do overlap in space to some extent but there is a tendency for clustering into modules, which is why the grid code is often described as modular. There are usually at least 4 or 5 modules, sometimes more.
In contrast to scale, for phase there is apparently no large-scale topography. This means that neighbouring cells can have very different grid phases – they may be together or completely apart. In any given local anatomical region of entorhinal cortex you have all phases present.
One of the most important properties of grid cells that Moser emphasized was that unlike place cells, grid cells are very rigid in the sense that the pattern of grid cells in terms of which are active at any location repeats itself from one environment to another so that you can often perceive it as a single coherent map that can be used over and over in different environments.
They also wanted to know whether the very rigid map is expressed when animals are not exploring the environment, for example when they are sleeping. Moser described how Richard Gardner, a post-doc in his lab recorded grid cells in the awake state when rats were walking around in an environment and then found pairs that were either in phase or out of phase. He found that those cells that fire together in the awake state also fire together in sleep state, in slow-wave or REM, and those that do not fire together e.g. out of phase in the awake state are still out of phase in sleep. This applies for the whole population and suggests that it is one pattern for cross-correlation and presumably we can infer that it is still the same ‘map’ that is expressed even in the sleep state.
Moser also outlined other types of cells including head-direction cells, that don’t have any particular spatial pattern but do have strong-directional patterns that fire when the rat’s head is in a certain direction; border cells, that fire along particular borders of the local environments; and cells that fire in relation to the speed of the animal, many of which are interneurons.
There are also a subset of cells that are spatially modulated but don’t appear to have any clear grid pattern at all. They are still quite reliable so they fire at the same locations and there seem to be a fair amount of these cells in the entorhinal cortex. They are spatially-modulated but aperiodic in the sense that they have no observable grid patterns.
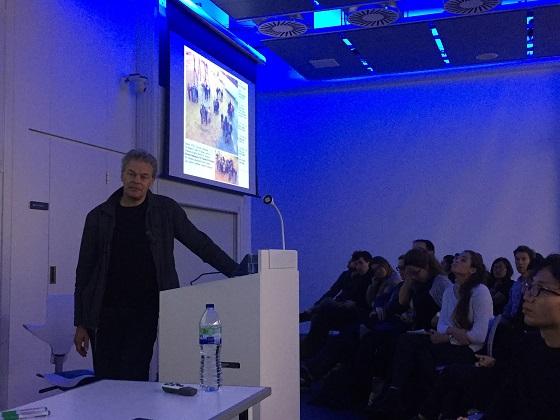
Integration of grid maps in merged environments
Following the overview of place cells in the hippocampus and grid cells in the medial entorhinal cortex, Professor Moser explained that most data to date has been collected in environments that are very simple and to some extent what we have seen has been a consequence of the conditions we have recorded in. He asked the question: would things look differently if we recorded in larger, more complex environments?
One of the PhD students in Moser’s lab focused on this question and found that the grid pattern is not perfect in most environments but distorted by the environment, especially along the walls. There is often a break in the middle where one part has a certain orientation and another has another orientation that leads us to ask whether different parts of the grid pattern as associated with different boundaries of the environment? If that’s the case, how are these fragments brought together?
To find the answer, rats were trained in two rectangular environments, A and B, next to one another and then one day the partition wall was removed so that it became one big environment. The researchers then looked into whether the grids from rectangle A and B somehow changed when the partition wall was removed. There were several potential results: the grid patterns could have been kept for A and B; one map could extend and take over the other; or there may be some sort of integration in the middle; or a new map entirely!
By comparing the maps in the A and B environments with the open AB environment and correlating spots in the environments, it was found that correlation was highly maintained along the outer walls and in the middle where the wall was removed there was a lot of change in the open environment.
Could it be that the pattern in the middle evolves towards a locally coherent grid pattern? Moser explained how this couldn’t be measured with a standard grid measure because that requires the measurement of a large space. Instead they asked for each field, what is the variation among the distances to the neighbouring fields.
As expected, it was found that before the wall was removed, there was high variation among the distances to the neighbouring fields if you allow to cross the wall as the two maps are independent. That variation disappeared after the wall was removed and the distances get more regular, implying a more locally coherent map in the middle.
It seems as though when you remove the wall, the two maps that have been independently anchored are still independently anchored to the periphery but meet in the middle and make themselves as locally coherent as possible. This is quite a fast process that happens within seconds or minutes.
Moser highlighted that the change in the centre of the box happens coherently among pairs of cells. If you record pairs of cells and correlate the maps before and after wall removal and make a cross-correlation, you can see the shifts in the map.
Object-vector cells
To understand the behaviour of cells in natural environments further, Moser described how a PhD student in his lab studied whether the cells in the medial entorhinal cortex respond to the presence of a discrete object or landmark within the environment. Most of the recordings that have previously been made have been from open boxes with nothing inside.
Towers were placed in the environment and it was found that quite a lot of the cells in the medial entorhinal cortex layer 2/3 responded with quite clear firing fields, not at the location of the object but at some distance away from it in a certain direction.
How are the tuning preferences distributed? All orientations were more or less represented. There was some multiplicity of around 90 degrees, which corresponds to the walls. There was also a distribution of distances from the object, which were found to be as much as 45 cm in an environment of up to 1 m. They are always in the same direction regardless of where the object is. Then Moser talked about how they tried different shapes and textures to determine whether it depends on the nature or identity of the object, but they found it didn’t seem to matter.
So what is it about the objects that makes the cells fire? Perhaps it is because they obstruct the mouse’s trajectory as they are walking around? This was found not to be the case as the researchers used hanging objects that were well above what the animal could reach so that they did not obstruct the mouse’s path in any way. Nonetheless, those cells that had object-vector responses to standing objects, also fired similarly to the hanging object, so the same response was seen regardless of whether the object blocked the animal’s trajectory or not.
That raises the question: how much do these object-vector cells overlap with other areas of the entorhinal cortex? Are they a subset of other classes of neurons, or are they distinct? Moser showed analyses of the cells to determine whether they had similar properties to grid cells or border cells, but they were found to be different.
It is particularly important to ask whether or not these object-vector cells are a subset of border cells. The object-vector cells were not found to fire along the peripheral walls of the environment at all. But how about the opposite: do border cells have vector responses when you put an object into the environment? Moser explained how the answer is both yes and no. For border cells quite often, when you put an object into the environment and the cell has a border field along the periphery, they maintain that field even if it is a small object. In other situations that is not the case.
Moser emphasised that the firing location of object-vector cells is not defined by the animal’s own movement so they are different to goal-orientation cells. They are also different from object-cells, which fire at specific locations of objects. However, they are more similar to an apparently quite rare population of cells called lambda-vector cells in the hippocampus, which fire at a certain distance and direction from objects but not from all objects.
There are several differences and the relationship between the cells in CA1 and those in the medial entorhinal cortex is not clear but they seem to be much more frequently present in the medial entorhinal cortex where they are at least as frequent as border cells.
Time-coding in the lateral entorhinal cortex
In the final part of his talk, Moser asked is there any encoding of time in the entorhinal cortex? For many decades, it has been argued that you would expect encoding of time in the hippocampus, especially because the hippocampus has such an important role in episodic memories for which it may be just as important to encode time as well as space.
“Time” cells have already been reported in the hippocampus and they are known to fire at certain times when the animal is doing a steady behaviour. In an experiment where animals ran on a figure of eight task and then on a running wheel, it was found that when they were running in the wheel you get sequential activity, so there was apparently some encoding of time during those trials.
This has also been shown in the entorhinal cortex where mice were trained in a virtual environment to run on a ball until they came to an invisible barrier, where they had to wait. Interestingly, the cells that record space, such as grid cells, are thought to be different to those that record time.
So there is time in the system, but these ‘time’ cells are for short periods in the order of a few seconds. Moser asked: is there time-coding over more extended intervals? He showed work demonstrating that the decorrelation of activity, or the distance between population vectors when rats do a sniffing task increases with time. And in other work, especially in CA1 and even more in CA2, the population vectors get more different the longer the time is between recordings. This correlation decreases much more in CA2 than in CA1 or CA3.
But if there is temporal information in this, where does it come from because the data from the medial entorhinal cortex, at least the ones that have been recorded, seemed like the intervals were too short? This motivated researchers to record in lateral entorhinal cortex, which Moser described.
Finally, Moser explained how if you change the task, you see different kinds of coding in the lateral entorhinal cortex. For example, he compared different types of structured tasks: a figure of eight task where the reward was found in the same place and a circle task where they had to learn. The discrimination was found to not be that good. The decoding accuracy was reduced in the figure of eight and circle task compared with a task involving foraging in an open environment. However, when comparing decoding of trial time, i.e. time within the trial, they saw that the prediction for decoding accuracy was higher than it was in the open field during foraging, whereas decoding accuracy for trial identity, i.e. whether trial 1, 2, 3 or 4, was reduced.
With training in a structured task you get better coding of time since the start of the trial, but less coding of time within the sequence of trials, which might suggest that as a consequence of learning the lateral entorhinal cortex changes the way it represents time and goes from a more general ‘absolute’ time code to a coding of the progression of time through the individual trials that the animals perform over and over again.
Object-vector cells versus boundary cells
Following Moser’s talk a number of questions were posed from the audience at Sainsbury Wellcome Centre, which sparked a lively debate on whether you would get the same response from a barrier as a new object in the object-vector cells and thereby whether these cells are actually just novelty-modulated boundary vector cells.
Professor John O’Keefe asked whether size was important and if you started changing the size would it morph into a boundary vector cell? Moser explained how they haven’t done that but it is something they could test.
Moser was also asked whether grid cells are modulated by introduction of rewards into the environment. He responded by saying perhaps there may be small changes in the firing rates of the grid cells but nothing that they have seen. However, they have very little data on this and there could potentially be rate changes that they haven’t picked up or changes in the population pattern.
Another question from Professor Neil Burgess focused on the anchoring of grid cells and whether, in addition to the boundaries, the cells were anchored to objects in the environment. Their experiment, Moser explained, didn’t investigate that, however, given the existence of such cells that respond to discrete objects, he responded that he wouldn’t be surprised if location relative to object is encoded.
Professor Tom Otis, Chief Scientific Officer, Sainsbury Wellcome Centre, highlighted that given the low dimensionality in the understanding of what the cells encode there seems to be an opportunity to do an experiment where you optogenetically remove activity in space and grid cells and look at the behavioural consequences.
Moser explained that at the moment they don’t have any way to specifically target grid cells over other cells, but hopefully one day they will find a gene that grid cells express that others don’t, which may then make it possible to turn off those cells, but they are not there yet.