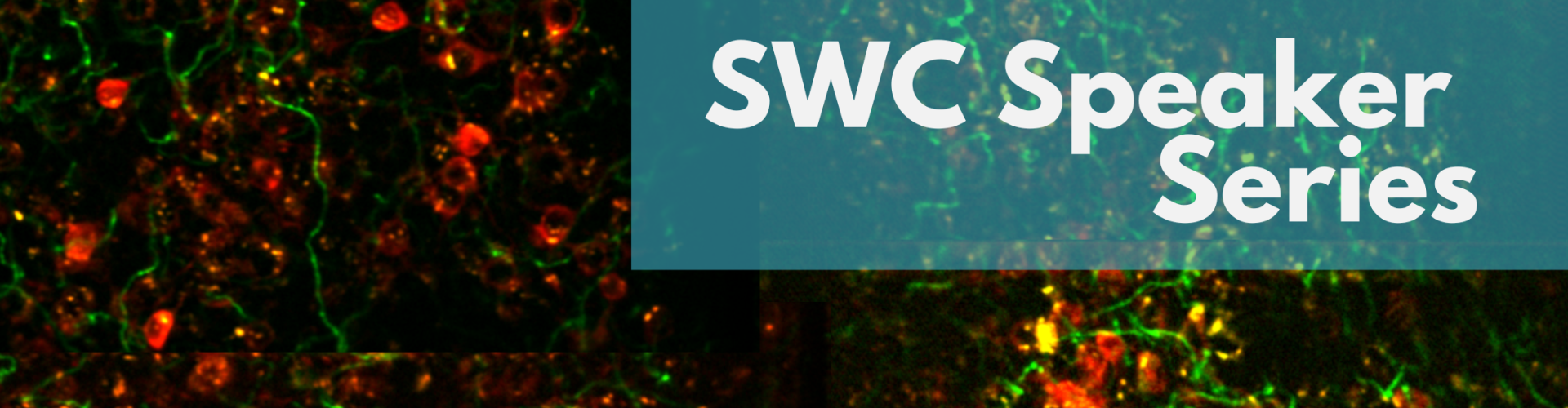
Exploring the gap between knowledge and performance
An interview with Dr Kishore Kuchibhotla, Johns Hopkins University, conducted by Hyewon Kim
What we know in our heads is not always smoothly translated into how we perform. Take learning to drive for example. While you might pass the written theory test one day, it doesn’t necessarily mean you’ll be easily cruising through busy roads the next.
In a recent SWC Seminar, Dr Kishore Kuchibhotla shared his lab’s work investigating the strategies and insights animals may use during sensorimotor learning. In this Q&A, he hints at how such findings could be implied in our own learning, his career trajectory, and how that’s informed his research direction.
How did you first become interested in studying sensorimotor learning?
It goes all the way back to why I got into neuroscience. I was an undergraduate physics major and in my third year I saw a talk by Sebastian Seung, who has just moved to MIT. He was a physicist and gave a great talk about theoretical neuroscience. That made want to explore neuroscience.
From that moment, I wondered about how we learn and remember. To understand learning and memory is why a lot of folks get into neuroscience. How is information able to come in from the outside world and create representations in our brain? How do we use this information whenever we need to? For me this is one of the most amazing things humans and other animals can do. We can learn from the environment around us, store those memories, and use them when needed.
That flexibility and adaptability are critical to everything in our daily lives. Trying to understand their neural mechanisms seemed fun to try.
Why do you look at task contingency signals? What do you mean by task contingency?
Say you’re driving and see a sign on the road that says STOP. You learn that the visual signal and word mean you need to push your foot on the brake pedal. And you’re not born knowing you need to go when you see a green light. You learn that it is a cue or signal from the environment that says it’s time for you to press your foot on the gas pedal. This is what we mean by a contingency in our work and the work of others. There is a sensory cue in the environment that needs to be linked to an action. How does that actually happen? How does that contingency get formed where a sensory or environmental cue can now reliably elicit the appropriate motor action?
Often, that type of learning is achieved with the presence of a reward. Sometimes it can be an explicit reward, like you learning that the sign for a Starbucks indicates the availability of coffee. You go in and get your reward, that’s great. With the traffic light example, the reward is just being able to move forward. This is an internal reward of being able to move according to plan. That’s what we mean by contingency.
Was it a surprise that the auditory cortex network formed a contingency well before you thought it did?
One of the things we’re excited about in our lab is that the brain, in any given moment, has a lot of what we call latent knowledge. We’re focused on how latent knowledge can help underlie the learning process. One way to think about it is, you can study for an exam and you feel like you know the answers at home, but when you go and take the test for some reason you may not be able to demonstrate that. So there’s a difference between what you know and how you perform. That example is maladaptive. Ideally, you’d be able to access all the knowledge that you have.
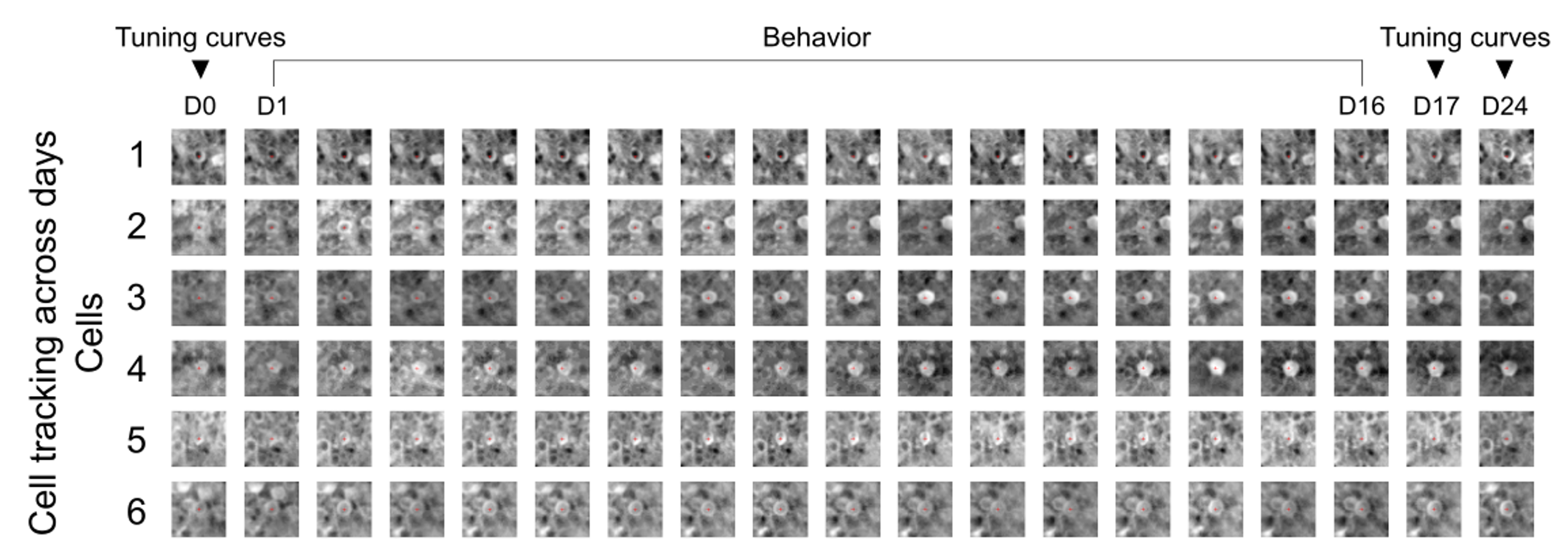
An alternative is that when animals are learning, they may know that water is in one particular location – say a babbling brook. But they may continue to explore because they want to see if there are other sources of water, maybe because they hear another noise. If you were just observing the mouse or fox from the outside, you’d want the animal to go the water source you know of. But they’re exploring because, while they know the water is there, they want to see if there’s something elsewhere.
We think that a lot of this is reflected in the brain activity and the auditory cortex may be important for auditory related tasks. Our idea is that there are two different learning processes:
- One is about learning the content. These would be the core contingencies. The sound of the water means there is water available.
- The other is about learning to optimise behavioural performance. In the rodents we studied using our particular task, the reason there may be a difference between what an animal knows and how it performs is not because it doesn’t know it, but actually because they’re actively exploring. They’re purposefully making mistakes to gain knowledge about the environment.
We think both of those computations are present in the auditory cortex and part of a broader distributive circuit that’s involved in these types of learning.
It’s fascinating that mice engage in rudimentary forms of hypothesis testing. How could this be manifested in the wild or ethologically-relevant environments?
Most of our work is in controlled laboratory environments, but there is a rich behavioural literature that looks at how animals behave under more ethological conditions. One can imagine multiple scenarios in which active or directed forms of exploration are really valuable. We know from studies in non-human primates – in apes, chimpanzees, bonobos – and other animals including birds like corvids that they do actively seem to have their own hypotheses and test them. It’s always an interpretation with behavioural data.
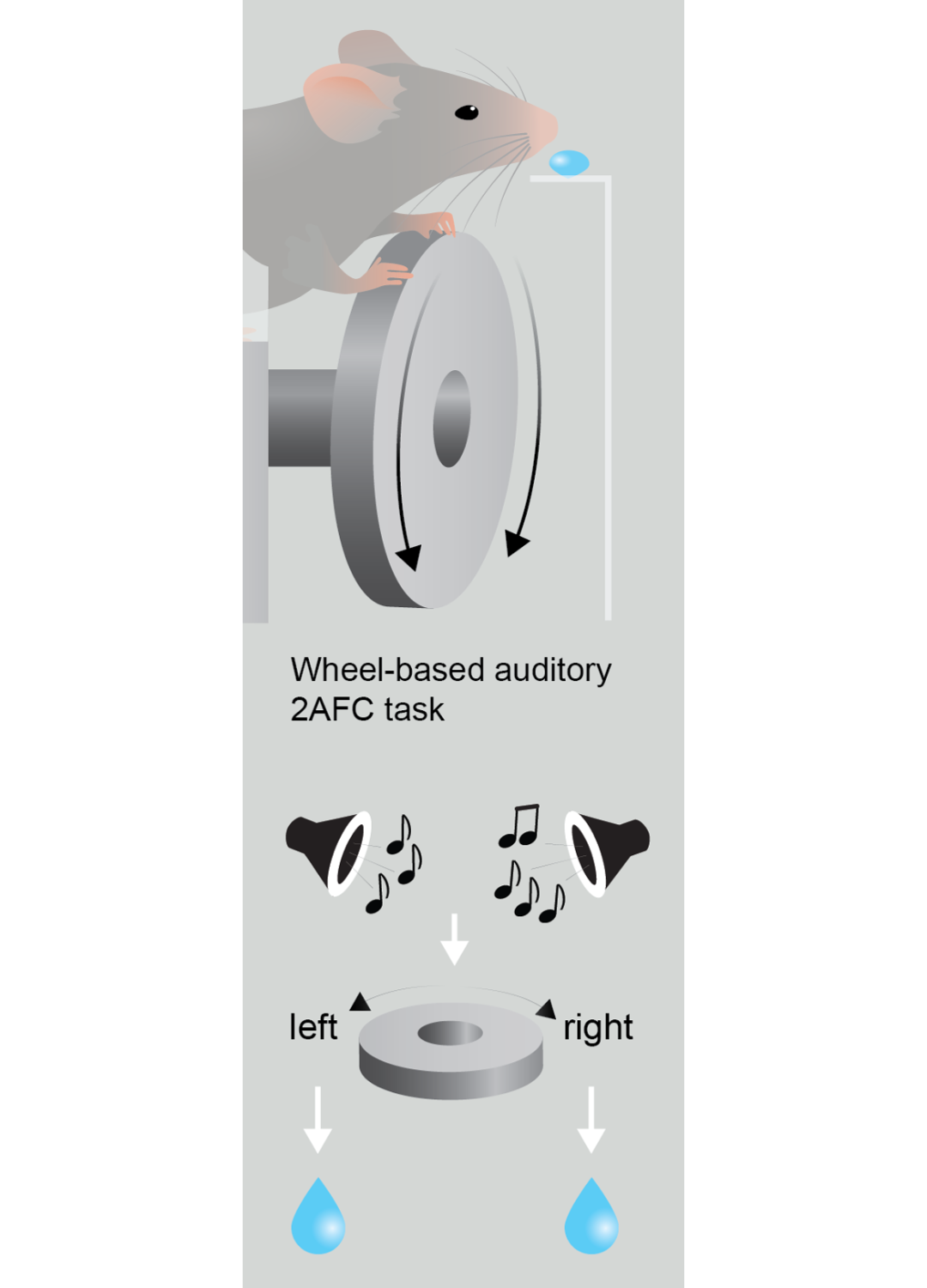
It’s a stretch to say it’s definitively hypothesis testing that these mice do, but we feel comfortable saying it’s a directed form of exploration. That could be very valuable for the animal. That means there is an internal model of where the water is or where the predator might be and they’re constantly evaluating whether that model is appropriate. They can do that through exploratory processes. The underlying idea is that there is an adaptive value to exploration. And if that exploration is directed, that means they are testing different ideas.
More broadly, what we are trying to understand is whether the common model of understanding rodent behaviour – a cumulative number of pairings between the sensory stimulus and the rewarded-reinforced action – is really all you need to learn, or do animals have higher order things going on?
Are there insights? Are there strategies that animals take? These are popular words and they sound cool, like a ‘Eureka’ moment, and we’re not saying that is what’s happening. Strategy also sounds very high level. It’s always hard to interpret behaviour to say there are these higher order processes going on. But a lot of our data, and those of other labs, suggest that these animals that we tend to think of as just blank slates slowly learning something actually have these moments where their learning curve is not linear. There is a nonlinear aspect to it. So there do appear to be types of strategies and insights.
Your team found that habits form within a few trials, through an abrupt transition. What could this mean?
We were surprised by that. It wasn’t even why we started that project. Dr Sharlen Moore, a very talented postdoctoral colleague, was very interested in developing new ways to motivate animals for tasks so that we don’t need to do as much water or food restriction. In doing so, we looked at the data and were surprised by what was happening at a certain time point. We saw an abrupt change from what we think is goal-directed to habitual behaviour.
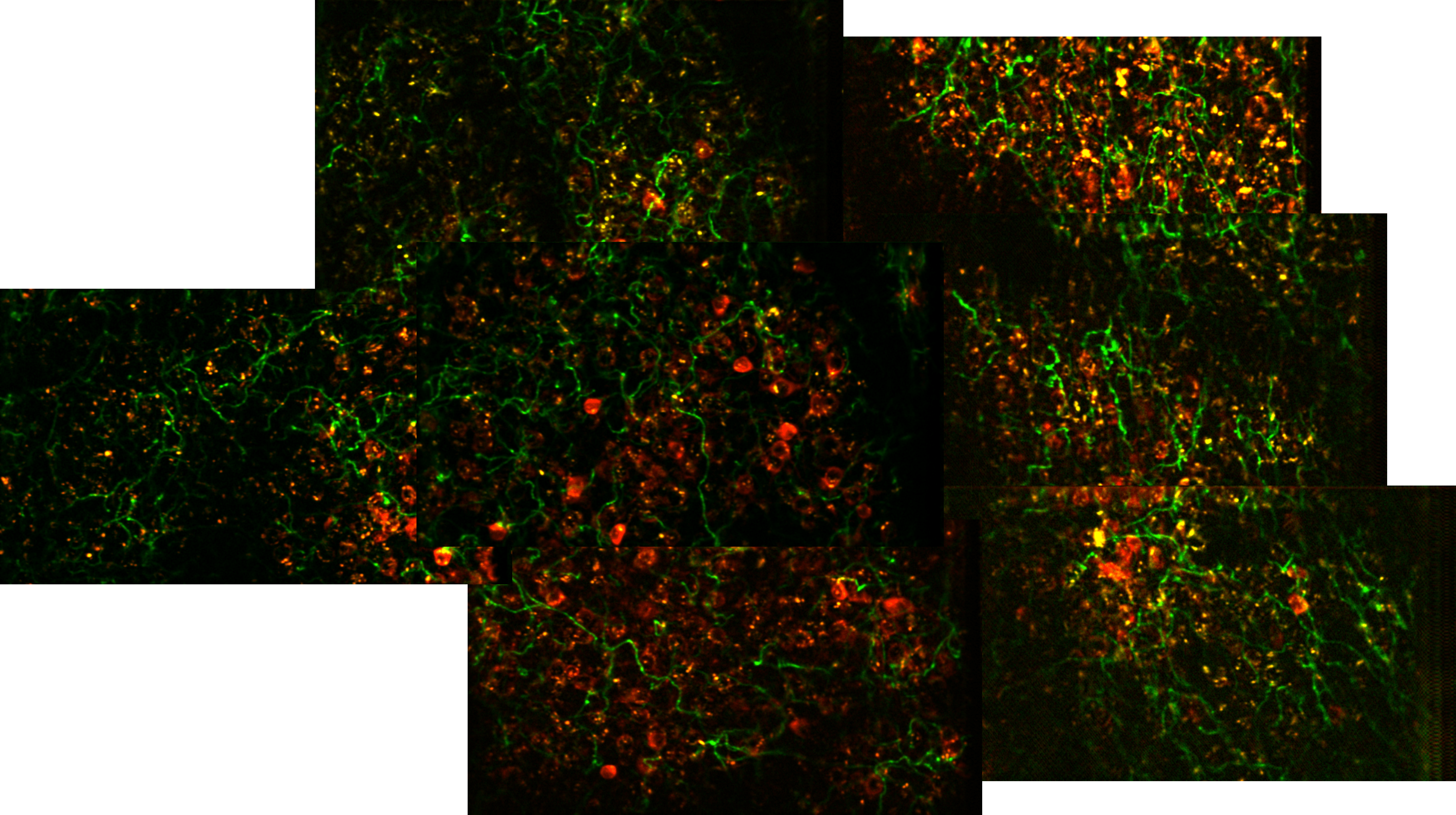
Our current model is not that the habitual circuit goes from nothing to something in five trials. It’s that the habitual circuit is also dormant. It’s being developed over the course of the goal-directed period, but for whatever reason it’s not used until this particular moment in time. Why does the behaviour shift to a habitual form of decision-making at that precise moment? Is it an active choice or a passive network shift? We don’t know. But it does appear there is some version of controller. That controller could be intrinsic to the decision-making brain area, for example the striatum. Or there could be a higher order area that impinges on it and lets the system know it’s wasting a lot of effort and energy deciding every trial whether to do it. The controller directs the system to perform the behaviour in an automatic way because that would be less effortful or metabolically expensive.
But those are just ideas that we don’t know for sure yet. We think there is a dormant habitual controller that develops alongside the goal-directed controller, and at a precise moment, the network for some reason decides to use the habitual controller. And that could be for a number of reasons – the two we’re interested in is because the network or the animal has realised the environmental uncertainty is low and they can shift to this habitual controller, and secondly because it can reduce metabolic energy usage and cognitive effort.
What implications do your findings on acquisition versus expression have for our own learning in daily life?
Extrapolation is always challenging because we are running experiments in reduced preparations. But there is a long history in cognitive science and linguistics thinking about the difference between knowledge or competence and performance. I think that’s an extremely important framework for human behaviour. Competence or knowledge is about acquiring the content. Performance is about how you use that content. The assumption that competence and performance are the same thing underlies many things in our society about how we evaluate people. One is trying to think about evaluations as whether we are trying to understand how someone performs or understand what someone knows. That’s an important question.
The second is connected to why there could be a difference between what we perceive as someone’s knowledge versus their performance. It may not always be negative. Our work suggests that a lot of those differences at least during learning processes could be adaptive forms of exploration. In our own lives, we may constantly be evaluating – should we take a chance and try something new or not? Rather than seeing that as risky behaviour, that could actually be very valuable. A lot of scholars have thought about this trade-off between exploration and exploitation.
Why do you think reinforcement can paradoxically mask underlying knowledge?
We have a very narrow answer in our study, which is that because the reinforcer is available, the animal is trying to explore more. Because it’s largely a deterministic task, the first time we omit reward, that surprises the mouse. The mouse thinks it did the right thing, even though it’s in this exploratory mode and making a lot of errors, and we omit the reward in the correct trials. We think this violation of expectation causes the animal to make sure what it knows is actually true. This is when the animal reinvigorates its contingency knowledge.
What that means is, paradoxically when the animal is actually being reinforced, which is when most of us tend to measure behaviour, it’s a mix of factors – what the animal knows about the contingencies, but also how the animal is trying to learn more about the environment by exploring. There could also be maladaptive factors such as impulsivity – the animal is thirsty and it doesn’t care about performance. There are a number of factors that exist when the animal is being reinforced that may not be as prevalent in these short blocks of non-reinforced trials.
Could you share details on the lifelong continual learning in a multitask playground project and your other future directions?
We’re really excited about this project. As I mentioned my lab is fascinated by learning processes and one of the things that humans and animals do really well is that they learn across their lifetime. You can learn how to do something when you are 20, not do it for five or ten years, then you can come back and still do it. In addition, you can use what you learned previously to build on it. If you know how to play tennis, you can pick up squash more quickly (though there may also be interference because squash and tennis techniques are really different!) You can take things from the past and build on them to learn something new. This is one reason we’re interested in that question.
The second is that most of neuroscience has focused on neural mechanisms of learning single tasks or maybe even two tasks. But the reality is that our brains are not there just to learn one thing. We put these animals in situations where they need to learn one thing, then we look at the representations and dynamics in relevant brain regions. But what happens when you get a mouse to learn 5, 10, or 15 tasks? How does that information get split up in modular ways in relevant brain regions? Or does it get combined? When we guide an animal to learn as much as it can in a relatively short period of time, track that over time and build new tasks in, that’s what we’re fascinated by.
This is what a talented graduate student in the lab, Aneesh Bal, is doing. The insight was to let animals live in a home cage, then get access to multiple behavioural arenas where they can perform different tasks. We can take advantage of the fact that animals may find this more fun, they’re socially housed, their stress would be lower, and it’s volitional – they only run through a tube, which they love. When they want something, that wanting could be for water or for playing a game. We don’t know which.
What we’re finding in that situation is that we can get mice to learn 8 different tasks in less than 30 or 40 days! These are quite different tasks – go/no-go’s, two alternative forced choices, changing perceptual dimensions, etc. We think this is going to open up a major opportunity to understand continual learning or multitask learning. It also allows us to better understand what the brain is there for, which is not being able to do one thing, but to flexibly and adaptively do many things based on the context.
How does your career trajectory inform your research efforts today?
I’ve had a lot of different interests over my life and I still do. I started as an undergraduate studying physics, then added brain and cognitive science, and along the way got interested in politics so ended up minoring in political science. I spent a year in DC working at a thinktank doing India-Pakistan nuclear security policy work and was missing science, so came back and did my PhD more related to Alzheimer’s. Then President Obama won the 2008 election and I was very excited about that. I wanted to be back in DC. I decided to work at a consulting firm that had a public sector practice. I did that for about two-and-a-half years. I really enjoyed it, but during that period also realised I missed being at the lab bench. It was bit of a process to get back in, but I eventually decided to apply for postdoc positions and to work on something I really want, to go down the path of running my own lab someday.
One of the things I’ve come to realise is that it’s okay to make somewhat irrational decisions, but when you do so, execute them rationally. On some level, this flipping and flopping back and forth may seem like it slowed my career trajectory down, but for me it was what I wanted to do. I think it’s important to follow that.
As for how this influences the research in the lab, most importantly it gives me some peace that this is what I truly want to do. And I love working with smart, talented people. At this stage of my career rather than always questioning that choice, given that I’ve had other experiences, I feel comfortable with this choice. I think that’s nice.
The second is that given the varied interest I’ve had in the past, I feel comfortable trying new stuff. Maybe it won’t work – most things fail. But I think it’s important to do that so that you can then learn something else. I would say that one of the features or bugs of our lab, is that we have projects on learning, on Alzheimer’s, both in animal models and humans, on echolocation sound processing and ethological behaviours in echolocating bats in collaboration with Dr Cynthia Moss, as well as on human learning. And now we’re developing an app and ecosystem to track cognitive fluctuations during lucid intervals in Alzheimer’s patients. There’s a lot of stuff going on, which can sometimes be challenging. But I made the decision that if we could get funding for these projects, it would be such a great opportunity, so let’s just have some fun. And maybe someday it could be useful.
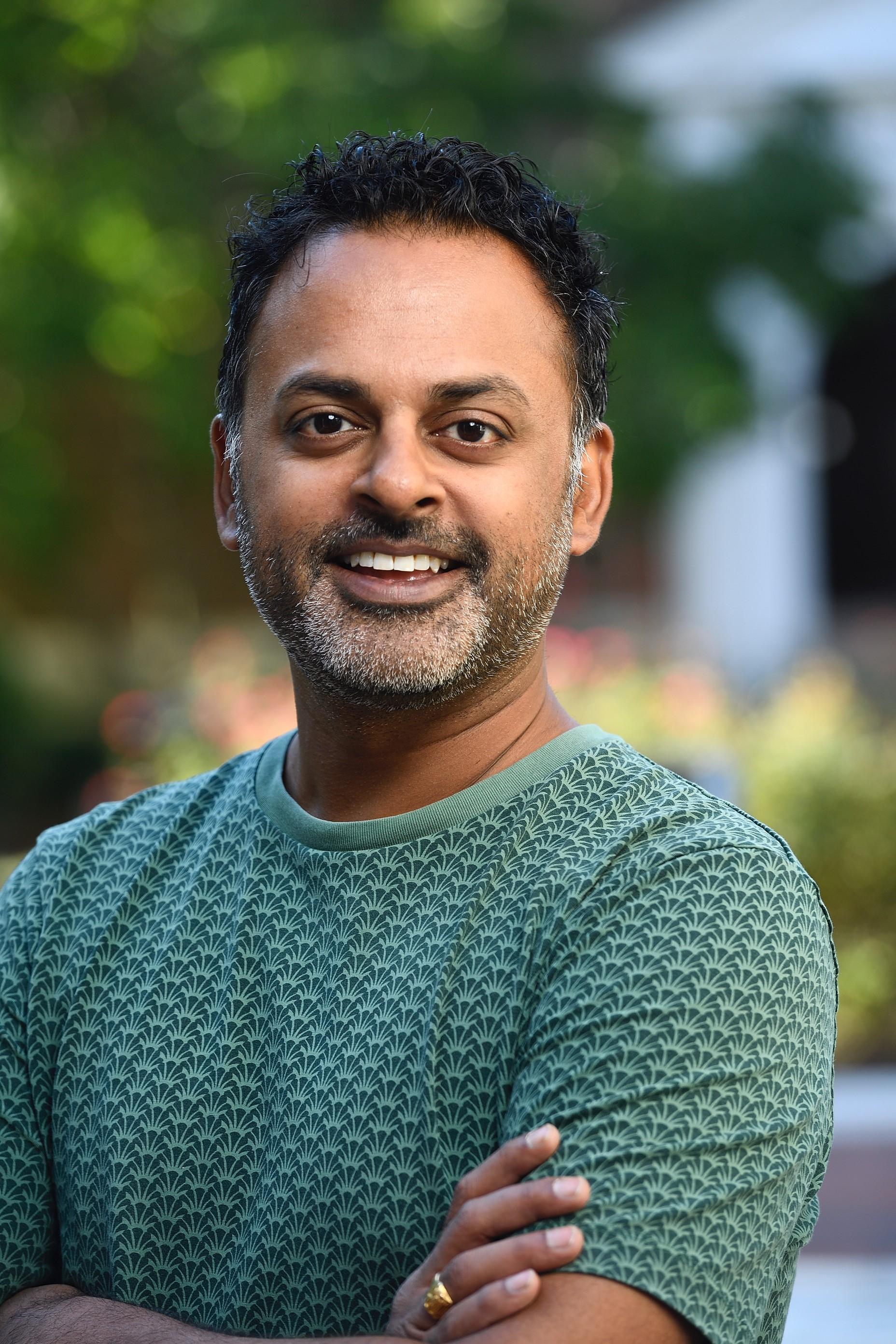
About Kishore Kuchibhotla
Dr Kishore Kuchibhotla is an Assistant Professor of Psychology and Brain Sciences, Neuroscience and Biomedical Engineering at Johns Hopkins University. His lab is interested in the neural circuits and dynamics that enable learning with a particular emphasis on single and multi-task learning, neuromodulation and cortico-subcortical interactions. He received his undergraduate degrees in Physics and Brain/Cognitive Science at MIT and a PhD in Biophysics at Harvard University where he studied Alzheimer’s disease. He performed his postdoctoral work with Prof. Robert Froemke at NYU where his interests in the auditory system, perception and cognition began to develop. In addition to his scientific interests, Kishore made multiple detours in his career to pursue his other interest, policy-making and government. After undergrad, he spent one year at a think tank working on India-Pakistan nuclear security policy. After his PhD, he spent 2.5 years working in the Public Sector practice of McKinsey and Company.