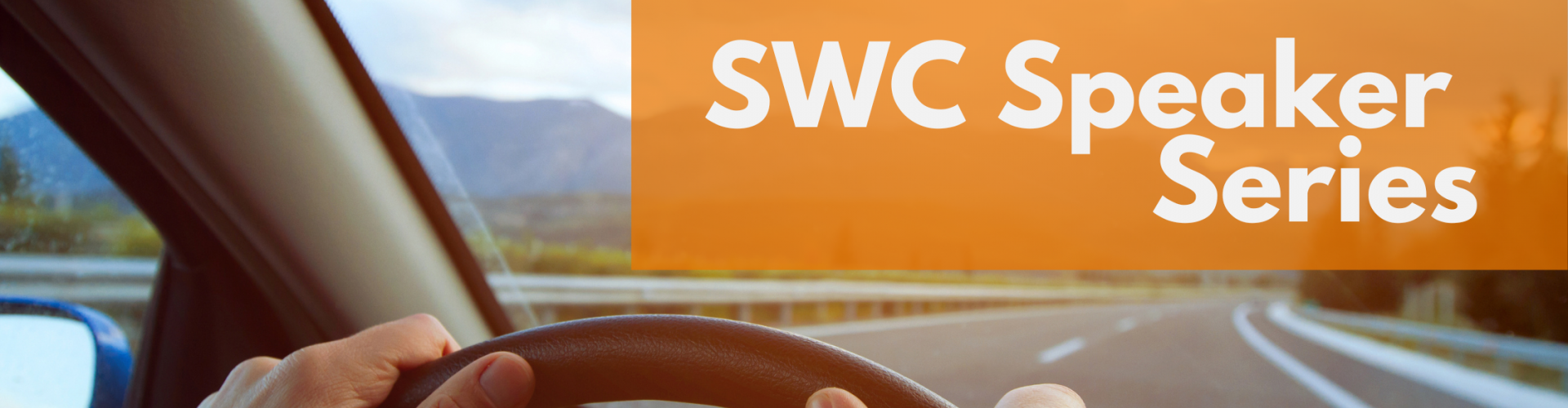
How do neural circuits implement flexible decisions?
An interview with Dr Ning-long Xu, Chinese Academy of Sciences, conducted by April Cashin-Garbutt
When you visit a country that drives on the opposite side of the road than you usually do, you are able to quickly adapt. How does the brain allow us to apply such rule-dependent flexible behaviour?
Dr Ning-long Xu recently gave a seminar at SWC on implementing flexible decision-making in single neurons and brain-wide circuits. In this Q&A, he explains what first sparked his interest in this field and how research in mice is allowing his team to uncover the neural circuit implementation of algorithms underlying rule-inferencing flexible behaviour.
What first sparked your interest in studying flexible decisions?
Context-dependent flexible behaviour is really a hallmark of intelligence in mammalian brains and is one of the building blocks for higher cognition. I wanted to understand the biological substrates underpinning the computations of this capacity.
During my postdoc, I focused on how dendritic nonlinear integration can impact behavioural output. After I established my own lab, I wanted to investigate how neuronal dendrites integrate task context information to facilitate flexible behaviour.
I read a paper from Dr. Anthony Zador’s lab, where they showed mice performing a flexible auditory categorisation task. In order to isolate the top-down contextual information, we modified the task to allow mice to use reward outcome as the feedback to guide their decisions.
This development, combined with our expertise in high-resolution in vivo two-photon imaging and circuit manipulation, allowed us to investigate how the prefrontal cortex provides feedback information to update the representation of current task rule, and how dendrites in single cortical neurons integrate top-down contextual information with sensory information to drive context-dependent behavioural output.
Why do you focus on the mesoscale level?
Over the past century, there has been a revolution in molecular biology and so we know a lot about cellular and molecular cascades, signalling pathways, gene expression and genetic mechanisms.
At the other end of the scale, there is this long tradition of cognitive science and psychology studies focusing on the rules of cognitive functions and psychological processes, and how different brain areas are involved in different cognitive and behavioural processes.
But there is really a great gap between these two different levels and that’s why the mesoscale level investigation is important. Fortunately, many researchers have realised this and so many of us are using all kinds of tools to study mesoscale, neural circuit level processing.
How much is known about the biological mechanisms underlying flexible decision-making?
While there have been plenty of psychological and behavioural level studies on flexible decision-making, very little is known about the underlying neuronal mechanisms.
Previous studies have identified roughly which brain areas are involved. For instance, lesion studies in non-human primates showed that the prefrontal areas, especially the orbitofrontal cortex, are involved in reversal learning, a type of adaptive behaviour. Human patients with prefrontal damage showed deficits in performing flexible categorisation tasks, such as the Wisconsin Card Sorting Test, which requires cognitive flexibility based on rule-inference.
But we really know very little about the biological basis at a cellular and circuit level. The main reason is that to study cellular and circuit level mechanisms you need to use animal models like rodents, where powerful tools are available to reveal single-neuron and circuit level operations. But it is very difficult to study higher-order, cognitive level functions in rodents.
Our strength is that we are good at training mice to do complex behaviour using our efficient behaviour control system allowing us to examine cognitive-level functions in rodents, and meanwhile we also have the expertise in the state-of-the-art circuit analysis technologies, such as two-photon imaging and optogenetics.
What are rule-dependent flexible decisions and how widespread do you think they are in mammals?
I think this is a fundamental cognitive function widely existing in highly evolved species such as mammals. The simplest kind of decision-making involves executing proper motor actions based on certain sensory input. For example, we see a red light we stop and we see a green light we go.
Rule-dependence involves being able to be flexible to an arbitrary change in the rule. Humans can do this immediately. For example, in China we drive on the right side whereas in the UK the reverse is true as people drive on the left. I can quickly adapt to the new rule when I am visiting London.
We are able to train mice to perform rule-dependent flexible decision-making tasks. But I believe other highly evolved species, such as birds, should also be able to produce such kind of behaviours. For example, scientists have been training pigeons to do very complex categorisation tasks.
Such behavioural flexibility allows animals to solve problems, make decisions, and adjust their actions based on the context they find themselves in. It is an important aspect of cognition and is thought to be a key feature that distinguishes more intelligent species from others.
Please can you outline the flexible decision-making task that you have developed?
Essentially, we trained head-fixed mice to make distinct motor choices (by licking left or right) based on the frequency of tones they heard. For instance, when presented with high-frequency tones, the mice needed to lick left; conversely, for low-frequency tones, they licked right.
However, the boundary defining tones as high- or low-frequency categories alternated between higher and lower frequencies. As a result, some tones situated between the two boundaries had to be flexibly categorised as either high or low, depending on the task rules. Therefore, mice needed to make different choices for these tones under varying task rules or contexts.
Remarkably, mice were capable of learning this demanding task. Moreover, after well-trained in the task, mice displayed behavioural indicators of rule inference, as they could swiftly alter their choices after encountering just one error trial following each rule switch. This behaviour allows us to investigate neuronal circuit mechanisms underlying inference-based flexible sensory-motor decision-making.
What techniques did you use to elucidate the underlying neuronal mechanisms?
We use two-photon imaging, optogenetics and circuit tracing tools. We are good at performing high-resolution two-photon imaging in behaving animals, which allows us to examine how populations of neurons represents task variables, and how cross-region circuits compute task-rule inference, and how single cortical neurons compute context-dependent flexible sensory-motor transformation.
We employ state-of-the-art tools including two-photon imaging, optogenetics, and circuit tracing. We are particularly good at high-resolution two-photon imaging in behaving animals, which enables us to investigate the representation of task variables by neuronal populations, the computation of task-rule inference by cross-region circuits, and the context-dependent flexible sensory-motor transformation computed by individual cortical neurons.
What have been your key findings so far?
We have made several important discoveries. First, we found that auditory cortical neurons could represent a hidden cognitive variable, namely the subjective auditory categorisation boundary.
Second, we identified a prefrontal-sensory cortical circuit that implements a novel learning algorithm for rule inference, which updates the subjective categorisation boundary.
More interestingly, we have recently obtained direct evidence supporting dendritic integration of context and sensory information to generate context-dependent flexible decision signals, a long-sought single-neuron computational mechanisms that could contribute to cognitive functions.
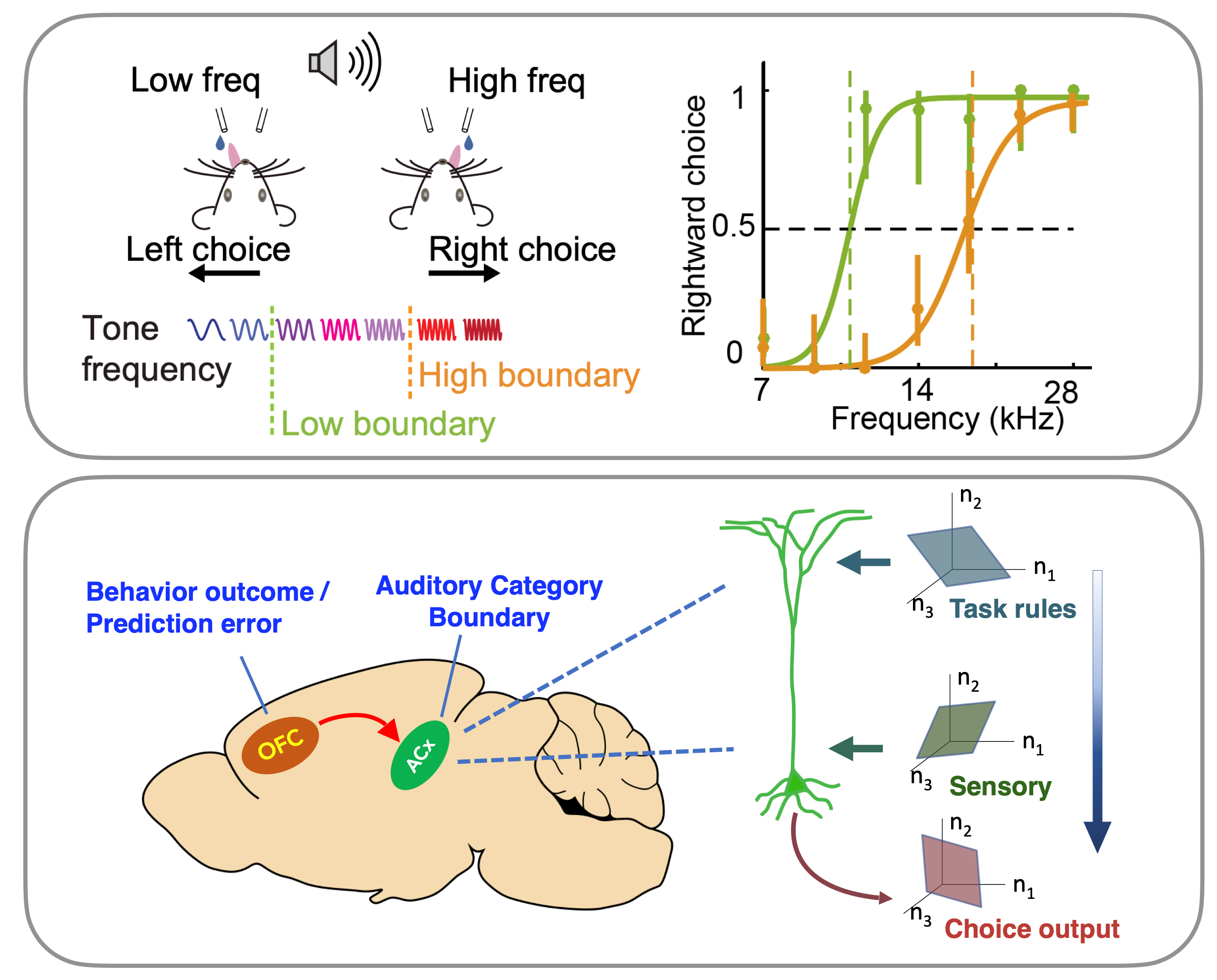
What are the main implications of your research?
We provide one of the first lines of evidence supporting cellular and circuit level implementation of a well-defined computation underlying flexible behaviour. In doing so, we have achieved David Marr's three-level understanding of the neuronal computational mechanism for flexible decision-making.
What’s the next piece of the puzzle your research is going to focus on?
In our auditory-guided flexible decision behaviour, mice use choice outcome to infer the current task state to quickly switch their choices. This can represent a general operational algorithm during context-dependent flexible behaviour. There are two key components of which the underlying neuronal mechanisms need to be dissected.
First, the animal needs to use task structural knowledge to make inference. How the brain represents task structural knowledge? What kind of circuit operations allow such representation to take effect?
Second, agents perform inference of current task state using external cues as feedback. The representation of inferred task states and ongoing updates potentially involve many cortical and subcortical circuits.
Therefore, our next steps are to conduct recordings and perturbations in many different brain areas to investigate the brain-wide neural dynamics and circuit logic that represent task structural knowledge and implement state inference computation.
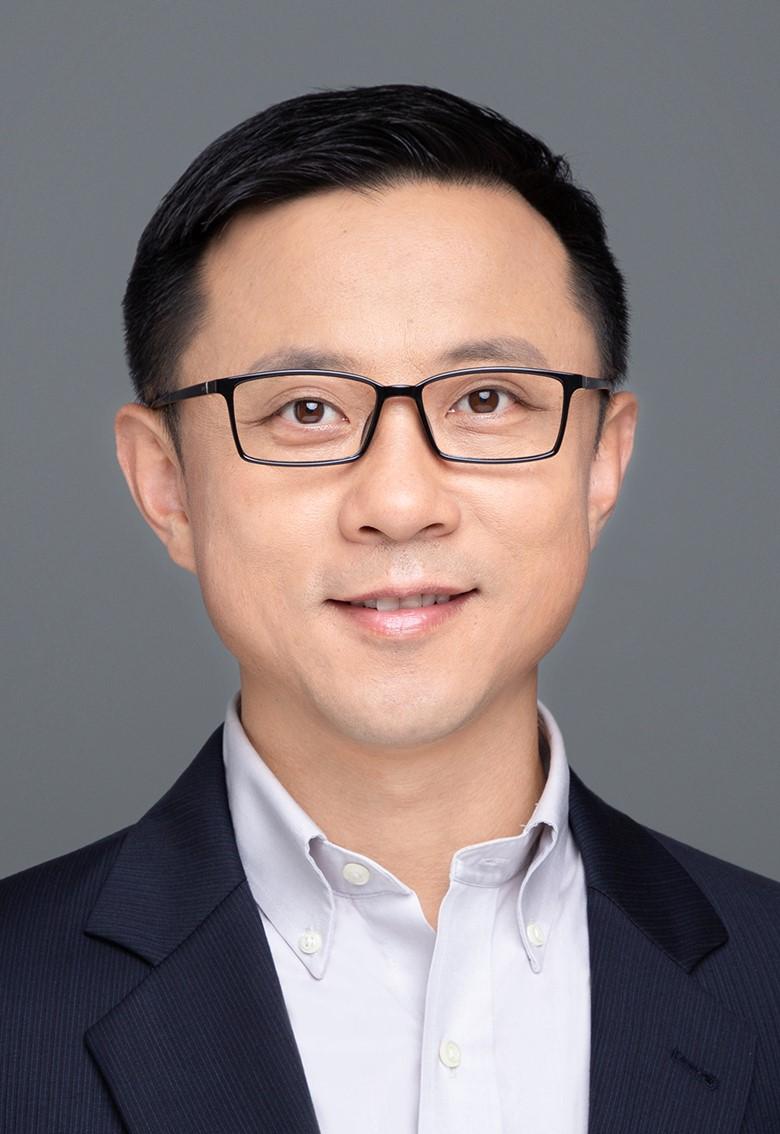
About Dr Ning-long Xu
Dr Ning-long Xu is Senior Investigator and Lab Head at the Institute of Neuroscience/CEBSIT, Chinese Academy of Sciences. Dr Xu received his Ph.D. in 2006 from the Institute of Neuroscience, Chinese Academy of Sciences, with Dr. Mu-ming Poo, and subsequently conducted his postdoctoral research at Cold Spring Harbor Laboratory with Dr. Zach Mainen and at HHMI Janelia Research Campus with Dr. Jeff Magee.
The laboratory of Dr. Xu combines complex and highly controlled cognitive behavioural tasks in head-fixed mice and marmosets with high-resolution in vivo two-photon imaging and circuit analysis tools to investigate how neuronal circuits in mammalian brain implement computational algorithms that give rise to perception and cognition.
Dr. Xu is the awardee of the National Natural Science Funds for Distinguished Young Scholars, and is leading several major national funding projects in China, including the decision-making project in the National Science and Technology Innovation 2030 Major Program.