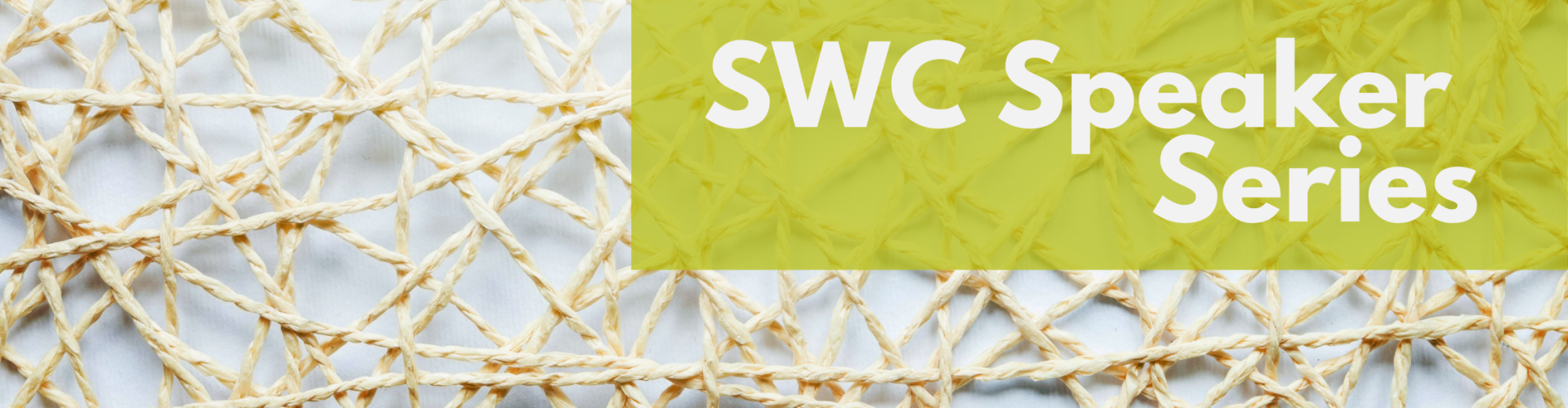
Unlocking the brain’s symphony with neural manifolds
An interview with Dr Juan Alvaro Gallego, Imperial College London, conducted by Hyewon Kim
Neural manifolds have become a cornerstone of neuroscience. Initially explored in locust perception, they now offer profound insights into brain function across species. SWC Seminar speaker Dr Juan Alvaro Gallego’s team have revealed patterns transcending individual boundaries, shedding light on the brain’s inner workings.
What are neural manifolds? How have they been used in the field?
It's a way to understand how our brain, made of billions of neurons connected to each other, functions. It utilises mathematical methods to grasp their collective behaviour and interactions.
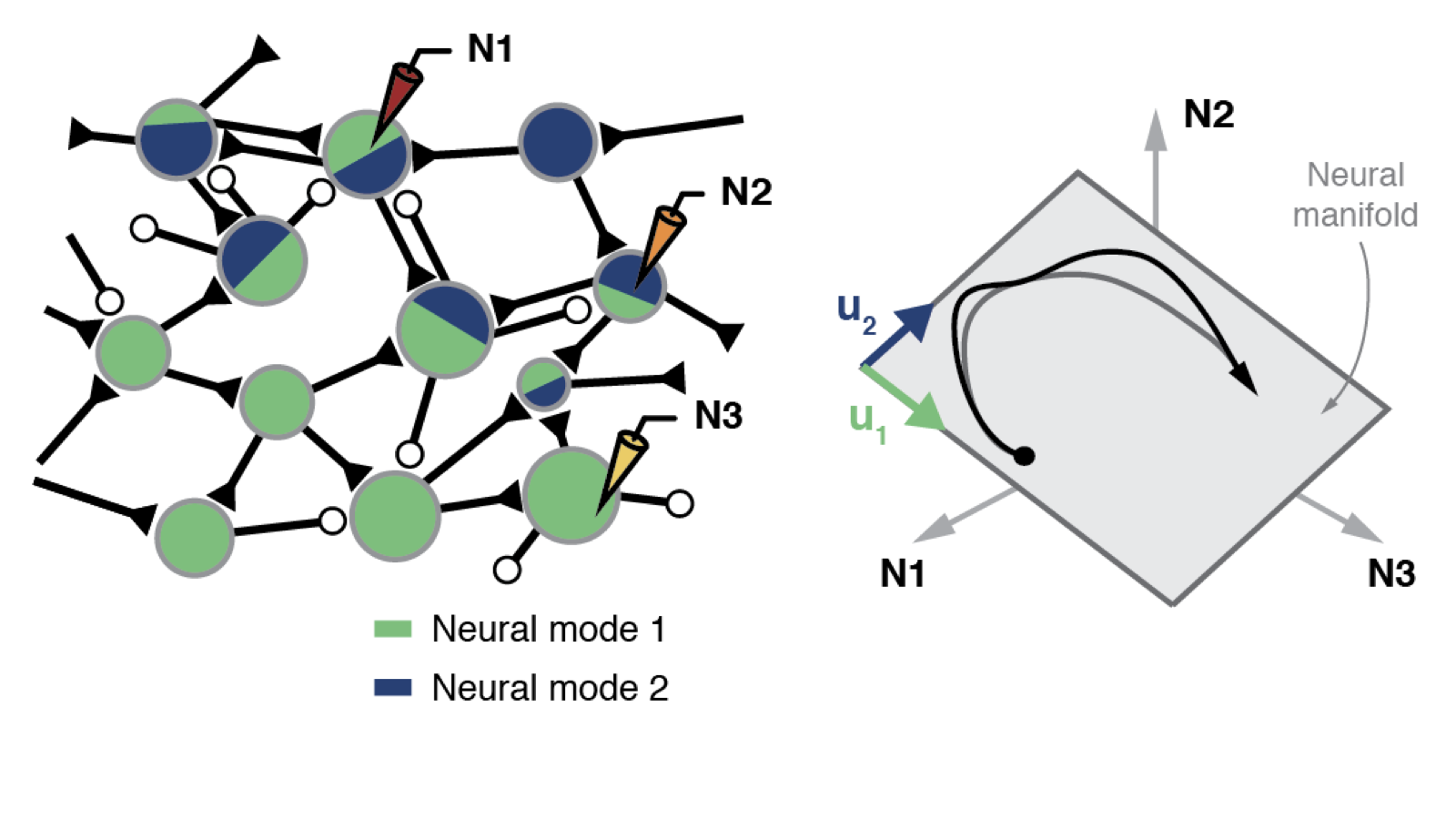
Originally, the term emerged in a 2003 paper, initially aimed at comprehending how locusts perceive stimuli. This research involved presenting various stimuli – like the scent of a banana – to locusts and analysing the resulting neuronal activity. Over time, the concept expanded to studying neuronal activity in mammals and other species, including humans and nonhuman primates. While the idea initially didn't gain significant traction, it gradually evolved, particularly with studies examining how monkeys moved, notably from the late Krishna Shenoy's lab at Stanford. This journey of exploration spanned several years before gaining broader recognition.
How would you say the field has received the idea?
It's funny because I'm not that old, but I've seen in the last less than 10 years a shift in how the community perceives this. I'm an engineer by training and I did my Ph.D. in biomedical engineering, but it was only during my postdoc I’ve made this observation.
At the beginning, a lot of people thought it was obvious or that it wouldn't teach us anything new we didn't already know. Now, the majority of people seem to be more convinced. But still, there are people who are not, which makes it more interesting. Science should be about these discussions that actually help you think.
Would you say the pushback comes from people trying to understand how to make the connection between single cell findings and population-level activity?
That sums up perfectly the tension in the field. And this happens a lot in the sciences.
A popular science book that I enjoyed reading is called A Different Universe by Nobel Prize in Physics Laureate Robert Laughlin. He talks about how hard it is to discuss many things working together and to convince people that looking at these interconnected elements can teach you more than examining each one individually.
So apparently, this is a tension in physics and chemistry, where a lot of these ideas came from. I think in neuroscience, where we're starting to think this way, it was only natural.
How did you become interested in studying neural manifolds and latent dynamics in the context of movement?
I was working on a movement project in a neuroscience nonhuman primate lab. And I found it very hard because it seems neurons have similarities, but each of them seems very different. I wondered, do we really need to understand all of them individually. Then there were a few papers coming out, and one of them made me believe that neural manifolds are not merely a way of looking at the same thing. Rather, the paper revealed that there was actually something more to them, suggesting that considering all these neurons together was more profound than merely considering each of them separately.
This paper showed that if you have an animal and record activity from, say, 100 neurons, and you find this neural manifold using simple tools from maths, it was easy for the animal to learn a task and generate different activity that lived in the same manifold. But it was very hard to generate activity that lived outside the manifold. This makes sense to me because if this manifold captures something about how these neurons work together, obviously making them work in a different way should be harder. Yet, simultaneously, it suggested to me that perhaps manifolds transcended maths; they captured something intrinsic to our brain.
Could you give a brief premise of your experiments and how you analysed the data?
We had monkeys perform a very simple task, akin to playing a video game where you move a cursor to obtain a reward. Monkeys excel at this due to their natural dexterity with their hands. Then, we examined how their neurons worked together during each movement.
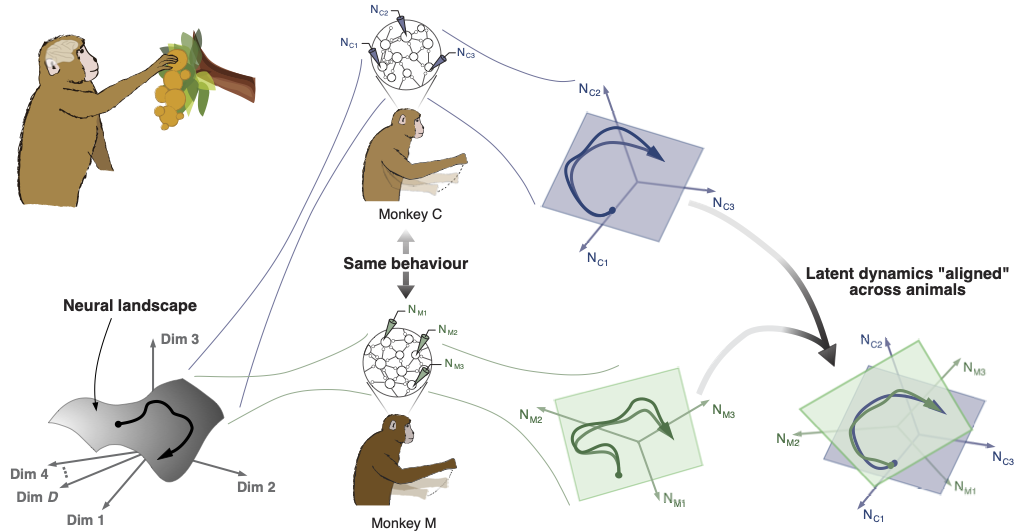
When you observe this data and plot it for two different monkeys, they appear distinct because you're examining different brains. However, if desired, you can look at the collective activity of the neurons from Monkey 2 and show this collective activity is the same as that of Monkey 1 after we do what we call “alignment”. To think of what I mean by alignment, imagine that the activity from Monkey 1 had the shape of your left hand, and that of Monkey 2 of your right hand: you can move your hands around and when the palms touch each other you’ll realise they are actually the same! The plotted correlations indicate the degree of similarity between them.
We conducted numerous comparisons both across monkeys and across mice. To gauge the similarity between their behaviours, we also compared within each monkey or mouse, when we were looking at the same neurons. This comparison allowed us to assess how similar the behaviours were within the same individual, across different individuals of the same species.
Were the results similar in mice to those in monkeys or were you surprised by what you found?
They were similar to what we expected, which complements the story: the more similar the behaviour of two animals, the more similar the collective activity of their neurons. I think this is because we examined other parts of the brain that are directly involved in moving and controlling the hand. However, it's worth noting that mice are not simply small monkeys, just as monkeys are not small humans, right? They are close, but there are distinctions.
So, I hope that if we look in closer detail at more complicated behaviours, we'll observe differences between the species. This is a question I am interested in pursuing in the future.
How do you combine neural recording data and computational models, like recurrent neural networks, to understand the role of neural manifolds in generating movement?
The figure on the paper was Joanna’s (one of my students) idea! We wanted to demonstrate that even with the same movements, you could have different neural activities resulting in the same movement. However, we couldn't find a way to alter the brain to change the activity. Perhaps it's possible if you train them to perform the same more cognitive task in different ways. But the method we employed involved instructing these neural networks to generate activity that produced the same movement but gradually became less similar when compared to a reference “mother network”. We had this "mother network”, and we attempted to make the activity of the other networks increasingly dissimilar while keeping the movement consistent.
We did this to demonstrate that, like many people were asking during my talk, the similarities in neural activity are not only about the behaviour being the same; it's also about having brains that are similar. For example, when a mouse is reaching to grasp, let's say, a piece of food, the neural activity it generates may be different from the activity that you or I generate when reaching to grab something like an orange in front of us, simply because our brains are different. So we wanted to emphasize that if you make the brains different, like these artificial networks, you could observe different activities, which was part of the controversy.
Where could this controversy be coming from?
I think part of the controversy in science stems from the fact that we are naturally sceptics and, to some extent, our beliefs shape our perceptions. Since science is a communal effort, it involves a combination of scepticism, intuition, and communal beliefs. So, when results align with our expectations, they may seem less interesting. Therefore, it's essential in science to engage in discussions and scientific arguments. From my perspective, discussions like the one during my talk are valuable because they can help clarify uncertainties, correct misunderstandings, or inspire new ideas for further exploration.
I think it is expected for different brains to arrive at the same solutions for the same task. Yet, what our research managed to achieve, and why it arguably garnered attention, is that we identified the level at which these brains are similar, encapsulated in this concept of neural manifolds.
Were the results you found surprising at all, or were they expected?
One challenge in the past was that, unlike today's improved technology, when electrodes are implanted into animal brains, the electrodes sometimes caused issues such as slight movement or scarring, resulting in changes in the recorded neurons over time. So, we faced the dilemma of determining whether the brain was truly generating the same activity during the same task when the neurons being recorded were not the same.
This predicament led us to develop the method we used in the study I presented in the first place. In that paper, we demonstrated we could address this issue and construct a model that predicts how the same monkey would perform the task two years later. Having spent so much time examining these plots, we had a strong conviction that this approach would be effective.
Perhaps younger scientists might not prioritise this aspect, but I believe it's crucial to deeply analyse—and look at—one's data, especially with the wealth of mathematical and programming tools available nowadays. We spent a lot of time doing this early on.
How does your team interpret what these results could mean?
We believe it's a promising approach to understanding the brain. Beyond the paper, we're enthusiastic about the new avenues it opens up for research. For instance, we're embarking on collaborations with other groups investigating various genetic models of diseases. We know that mice, like humans with the same disease, undergo genetic mutations that alter their brain functioning.
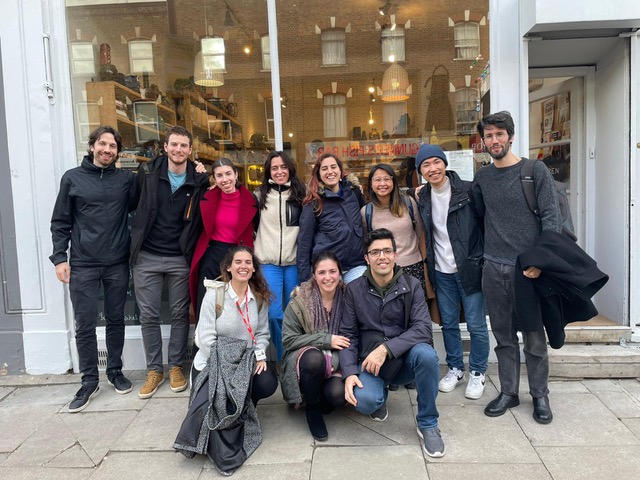
Our aim is to record neural activity during the same tasks and analyse how it differs across animals with different mutations, thereby linking changes in activity and behaviour to changes in genes. While it won't be straightforward, it will be fun and I’m sure we’ll learn something along the way.
Another aspect we're exploring is how animals learn more cognitive tasks, such as comprehending rules, and whether the solutions they devise are consistent. We're particularly interested in understanding when and why animals generate different activity patterns or, in other words, when our method to “align” activity across animals fail.
Why did you focus on rapid learning?
I think one of the most awesome things in our lives is learning new things. The intriguing thing about motor learning is that it feels trivial but it’s an important part of our lives—for example, getting better at cooking or taking up a new instrument—and the good thing is that this rapid learning can be observed within the same day in an experiment. It’s easier because you can record from the same neurons while an animal is performing a familiar task and then introduce a perturbation.
For example, if you're moving a cursor on a screen, you can rotate the cursor, causing it to move to the side when you move your hand up, or if you're interacting with a robot, the robot can push against your arm to move your arm away from where you want to go, among other possibilities. So, you can conduct these types of experiments and gather data, and—in our case—look at what happens in the brain.
Ultimately, our goal is to delve into more complex tasks, like learning to play the guitar proficiently. We would love to understand how we become skilled at something. Currently, we are exploring with models and data what occurs when, for instance, you're proficient at guitar and start picking up the bass, which is quite similar. However, the dream scenario would be to investigate how you learn to play the trumpet if you're unfamiliar with it.
What are some future projects you’re excited about?
I'm very interested in the various collaborations the lab is engaged in and a new brain-computer interface we are developing in the lab to ask many questions. One project I find particularly exciting involves a reversible model of stroke, where our colleagues can deactivate an entire brain region and observe resulting motor deficits. It's fascinating to learn how animals find alternative ways to perform movements despite a big part of their brain being turned off.
We're eager to understand how the brain accomplishes this feat, as it could have implications for stroke rehabilitation in the future.
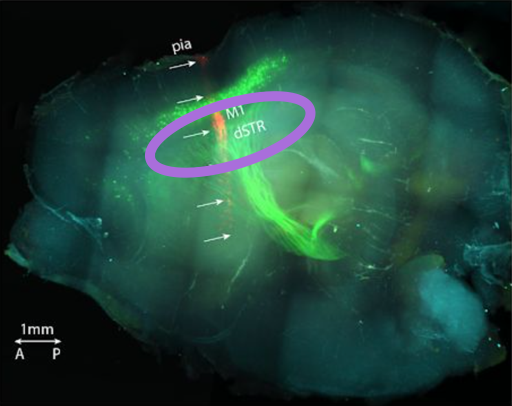
Additionally, our lab is also developing a brain-computer interface to investigate how manifolds in different brain regions can be altered through learning and understand the “rules” that different parts of the brain may use for learning.
What do you think is the role of neural manifolds in understanding or improving artificial intelligence?
A lot of the early work on population activity or neural manifolds involved training neural networks to perform tasks similar to those performed by animals or humans in the lab. Researchers then examined how these networks figured out tasks and looked at their neural manifolds, and found that artificial neural networks produced activity patterns resembling those observed in animals. This was surprising because these neural networks are mathematical models trained in a very artificial manner.
Another potential application, which some researchers are exploring, is to try to capture the brain's ability to learn and retain multiple skills simultaneously. By analysing neural manifolds, we can gain insights into how the brain achieves this, which could inform the development of artificial intelligence models capable of performing diverse tasks. These are two of the many avenues of research that people are working in. Additionally, there's also interest in applying similar approaches to models like ChatGPT, aiming to understand the rules obeyed by the grammar in our brains. I believe these methods offer a promising way to gain insight into complex models that are otherwise difficult to comprehend.
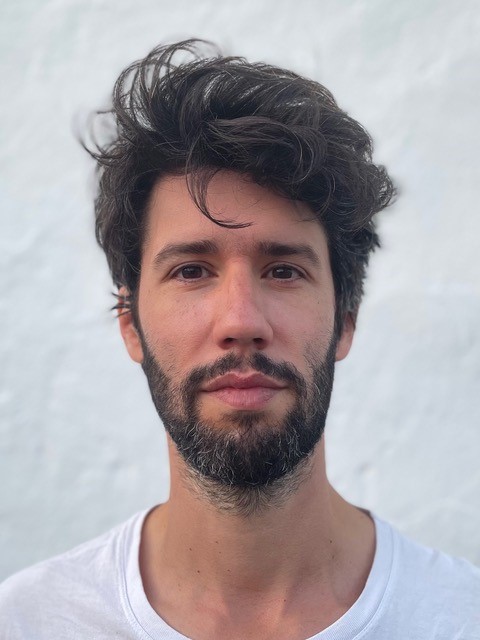
About Dr Juan Alvaro Gallego
Dr Juan Gallego is a Senior Lecturer in the Department of Bioengineering at Imperial College London, which he joined in January 2020. Prior to that, he held Postdoctoral Fellowships at the Spanish National Research Council (CSIC) and Northwestern University. He was awarded a PhD from CSIC and University Carlos III in 2013. His research focuses on understanding how animals including humans learn and control their movements through a combination of behavioural experiments, large-scale neural recordings, data analysis techniques, and computational models. He is also interested in applying this knowledge to advance neural interfaces to restore function to people with movement disorders. During his career, he has published thirty journal articles on these various topics. His research has been funded by the EU Commission, the UK Research Institutes, and the ERC. Dr Gallego is an elected board member of the Society for the Neural Control of Movement and is part of the program committee for COSYNE.